Frequently Asked Questions (FAQs)
- What is the purpose of this tool?
- What is the tool NOT intended to do?
- What do I have to know in order to use the tool?
- What if I don't know some of the input values?
- How were these input variables chosen, and not others?
- What outcomes does the tool estimate?
- For what conditions does the tool produce estimates?
- What is BMI (Body Mass Index)?
- How representative is the model of my company?
- How closely does the tool estimate my "real" costs?
- How was the tool created (basic methodology)?
- How was the tool created (advanced methodology)?
- Who developed this tool?
- Why was the tool developed?
1. What is the purpose of this tool?
This tool is designed to use input data provided by human resources or benefits personnel to calculate an estimate of the costs to an organization that are obesity related.
2. What is the tool NOT intended to do?
The tool is not intended to be used for making hiring or other personnel decisions.
3. What do I have to know in order to use the tool?
At a minimum, the user must enter the number of employees in the company. If available, other information that will increase the precision of the estimates includes:
- Type of industry (from a list of 12 industry categories)
- State or territory that best represents the location of the company
- Number of employees overall, by gender or age category (18 to 44 and 45 to 64), or by gender by age category
- Number of overweight or obese employees by the same stratifications
- Average wage information for employees by the same stratifications
- Proportion of employees receiving benefits by the same stratifications
- Share of total compensation going to benefits
4. What if I don't know some of the input values?
Default values from nationally representative datasets are provided for the optional inputs if the user does not have such information.
5. How were these input variables chosen, and not others?
These inputs are required to calculate the medical and work loss costs attributable to obesity and were chosen in part based on data availability and after discussions with potential users concerning which variables were most important to include in the model.
6. Which outcomes does the tool estimate?
The tool estimates the costs of obesity based on characteristics of your company. These include costs for medical expenditures and the dollar value of increased absenteeism resulting from obesity. Costs are estimated separately for four groups based on body mass index (BMI; measured as weight in kilograms divided by height in meters squared):
- Overweight (BMI 25-29.9)
- Obese 1 (BMI 30-34.9)
- Obese 2 (BMI 35-39.9)
- Obese 3 (BMI > 40)
7. For what conditions does the tool produce estimates?
- Overweight (BMI 25-29.9)
- Obese 1 (BMI 30-34.9)
- Obese 2 (BMI 35-39.9)
- Obese 3 (BMI > 40)
8. What is BMI (Body Mass Index)?
Body mass index (BMI). A measure of body weight relative to height. BMI is a measure that is often used to determine if a person is at a healthy weight, overweight, or obese, and whether a person’s health is at risk due to his or her weight. To figure out BMI, use the following formula:
[height (in)]2
For adults 20 years of age and older, a BMI of 18.5 to 24.9 is considered healthy (normal weight). A person with a BMI of 25 to 29.9 is considered overweight, and a person with a BMI of 30 or more is considered obese.
Note: BMI is used as a screening tool to identify possible weight problems for adults. However, BMI is not a diagnostic tool. For example, if a person has a high BMI, a health care provider would need to perform further assessments to determine if the excess weight is a health risk. These assessments might include evaluations of diet, physical activity, family history, blood tests and other appropriate health screenings.
It is important to remember that although BMI correlates with the amount of body fat, BMI does not directly measure body fat. As a result, some people, such as athletes, may have a BMI that identifies them as overweight even though they do not have excess body fat.
From: www.cdc.gov/nccdphp/dnpa/bmi/adult_BMI/about_adult_BMI.htm#Interpreted
9. How representative is the model of my company?
The inputs for the model are based on either nationally, state, or industry representative data. The extent to which the data is representative of your company depends on whether the employee profile in your company differs significantly from the national, state, or industry average. The greater the level of detail you input in the tool (e.g., number of employees by gender by age), the more representative the estimates will be for your company.
10. How closely does the tool estimate my "real" costs?
If your company’s employee profile is typical for your industry or state, then these estimates should be a good approximation of your costs. However, because of the uncertainty (variance) surrounding the estimates, actual costs could be higher or lower than those reported in the model. The degree of uncertainty decreases as the firm size increases and as more user-defined inputs are entered into the model.
11. How was the tool created (basic methodology)?
The basic methodology applies nationally representative data to compare medical expenditures and absenteeism costs between normal weight employees and those who are overweight or in one of three obesity classes. All else equal, the difference in these outcomes is a measure of the additional costs attributable to overweight and each obesity class. Multiplying these costs times the predicted number of employees in each BMI class allows for quantifying the total costs of obesity.
12. How was the tool created (advanced methodology)?
Obesity Prevalence
Obesity prevalence for four categories of obesity (overweight (25<BMI<30), obese 1 (30<BMI<35), obese 2 (35<BMI<40) and obese 3 (BMI>40)) were calculated by industry and by state. Within industries and states, the prevalence rates were calculated by gender, by age (18 to 44 and 45 to 64), and by age and gender.
Prevalence rates by state were obtained from self-reported height and weight from the 2008 Behavioral Risk Factor Surveillance System (BRFSS).
Prevalence rates by industry were obtained from self-reported height and weight from the 2008 NHIS. The industry categories were based on NHIS recodes of the 2007 North American Industry Classification System (NAICS) and the 2000 Standard Occupation Classification (SOC).206 Some industries were combined further due to small sample sizes in the industry/weight/age/gender subgroups. The 12 industry categories (NAICS codes) were Agriculture, Forestry Fishing, and Hunting (111-115); Construction (236-238); Education Services and Health Care and Social Assistance (611, 621-624); Arts, Entertainment and Recreation and Accommodation and Food Services (711-713, 721-722); Finance and Insurance and Real Estate and Rental and Leasing (521-524, 531-533); Information (511, 512, 515-519); Manufacturing (311-316, 321-327, 331-337, 339); Other Services except Public Administration (811-814); Professional, Scientific, and Technical Services, Management of Companies and Enterprises, and Administrative and Support and Waste Management and Remediation Services (541, 551, 561-562); Public Administration (921-928); Transportation and Warehousing and Utilities (481-488, 491-493, 221); and Wholesale and Retail Trade (423-425, 441-448, 451-454). If a sample size for the specified group (e.g., industry/weight/age/gender) was less than 70 people (before applying sample weights), then the estimated prevalence was replaced with the prevalence for the less stratified group (i.e., by gender by age estimates were replaced with by gender estimates; by age estimates were replaced with overall estimates for the industry). This occurred primarily for female subgroups within agriculture.
Prevalence rates for each weight category were adjusted for self-reporting by multiplying by a scaling factor. The scaling factor was the group-specific ratio of prevalence for the weight category based on measured BMI from the 2005 and 2006 NHANES to the self-reported prevalence in the NHIS (or BRFSS for state estimates). The NHANES could not be used directly since it did not provide industry codes or state identifiers. The approach assumes that self-reporting bias does not vary by industry but does allow self-reporting bias to vary by obesity category, age and sex.
Hourly Wages and Benefits
Average hourly wages by industry were estimated from the March 2008 Current Population Survey (CPS). Within industries, average hourly wages were calculated for the overall population of full-time workers ages 18 to 64 and by gender, by age, and by gender and age. Average hourly wages were calculated from average weekly earnings from the sole or primary job by dividing by 40 hours.
Estimates of total compensation, defined as wages plus the value of benefits offered to employees, were obtained by scaling average hourly wages by a benefits multiplier. The benefits multiplier is the percentage of an employee’s total compensation that is received as benefits. The national estimate for this share is 33% based on data from the Statistical Abstract of the United States.207 User-defined benefit multipliers are used when available. Average hourly wages for each industry and demographic group are a weighted average of wages for those with benefits and wages for those without benefits, with weights equal to the percent of full-time workers with benefits in each industry/group as calculated in the 2008 NHIS.
Average hourly wages do not vary by weight category as the CPS does not provide information about weight. Thus, the calculator assumes that the value of work time lost to illness is the same across all weight categories. There is some evidence in the literature that obese employees earn less than normal weight employees, but generally only for women.205
Total Medical Expenditures
Econometric modeling was used to estimate the costs associated with obesity using the 2003 through 2007 Medical Expenditure Panel Survey (MEPS; see Finkelstein et al. 2005, 2007 for examples of the methodology).3, 202 We estimated the total medical costs associated for each obesity category separately for the full-time working population ages 18 to 64 by gender and age. We pooled the five most recent years of data to increase the sample sizes available for the sub-group analyses.
Based on extensive specification testing as recommended by Manning and Mullahy (2001), Buntin and Zaslavsky (2004), Manning, Basu, and Mullahy (2005), and Hill and Miller (2010), we chose a two-part model of total expenditures.204, 205 The first part was a logit model for the probability of positive expenditures; the second part modeled expenditures for people with positive expenditures using generalized linear model (GLM) with log link and gamma variance function. Expected annual per capita expenditures were predicted by multiplying the probability of positive expenditures by expected expenditures conditional on having positive expenditures. All regressions included weight category (underweight (BMI<18.5), normal weight (omitted), overweight, obese 1, obese 2, and obese 3), age, age squared, gender, race/ethnicity, education, household income, smoking status, Census region, and year.
For each by gender and age subgroup, the medical expenditures per person attributable to obesity were calculated using the following method.
- Expenditures were predicted for each individual based on observed weight categories and other covariates.
- Then, we subtracted from that predicted expenditures for an otherwise identical person of normal weight.
- The difference in predicted expenditures from steps 1 and 2 were then averaged among each weight category.
This counterfactual indicates the extent to which medical expenditures per overweight or obese person would be lower in the absence of excess weight, all else constant. Total medical costs by gender, by age, and for the overall population were calculated by applying weighted averages to the costs estimated by gender and age.
Due to the lack of state identifiers and the small sample sizes within industries, the per-person estimates of medical expenditures attributable to excess weight are nationally representative. The total medical costs of overweight and obesity to companies vary by industry or state due to differences in prevalence of overweight and obesity across industries and states.
Medical Expenditures by Point of Service
In addition to providing estimates of total medical expenditures, the calculator includes a breakdown of the medical costs by the following points of service.
- Prescription drug costs
- Inpatient costs
- All other medical costs
The specification testing described above was also conducted for each point of service and the same econometric model that was used to calculate total medical expenditures was used to quantify cost estimates by point of service. Expenditures for each point of service were estimated separately by weight class for the overall full-time working population, ages 18-64. For each weight class, we summed these estimates and calculated the percentage of total medical costs spent on each point of service. We then applied these percentages to the total medical cost estimates of each weight class for overall population and by gender, age, and by gender and age to calculate estimates of medical expenditures by point of service.
Work Loss
The days of work loss associated with obesity were estimated using the 2004 through 2008 National Health Interview Survey (NHIS). We estimated the days of work loss separately for the same groups as in the medical expenditure analysis. The dependent variable was annual days of work missed. We used a negative binomial model with log link to account for the fact that the dependent variable was a count. The model included weight category, age, age squared, gender, race/ethnicity, education, household income, smoking status, whether paid hourly, years of work experience, family size, alcohol consumption, and functional limitations.
The number of annual work days missed attributable to obesity was calculated using similar steps as for medical expenditures. The difference between predicted work days lost at observed weight and predicted work loss for an otherwise identical person of normal weight was calculated for each person in the sample. The estimate of lost work days attributable to overweight and obesity was the average of this difference among people in each weight category. The calculator multiplies the estimates of the average days of work missed due to obesity by average hourly cost of an employee and by eight hours in a work day to report the financial costs of absenteeism due to obesity.
Due to the lack of state identifiers and the small sample sizes within industries, the estimates of lost work days per-person attributable to excess weight are nationally representative. The total value of lost work days to the company due to overweight and obesity varies by industry or state due to differences in prevalence of overweight and obesity across industries and states and variation in compensation across industries.
Disease Prevalence
The prevalence of hypertension, cardiovascular disease (CVD), diabetes, dyslipidemia, arthritis, asthma, depression, injury, chronic obstructive pulmonary disease (COPD), mental health/substance abuse problems, and back disorders by weight class were estimated using data from the 2003-2007 MEPS. Diseases were defined using Clinical Classification14 and ICD-9 codes based on self-reported diseases that were transcribed by professional coders and reported in the MEPS Medical Conditions files (see Table below). Prevalence was defined as the percentage of the population reporting treatment for or problems with the disease in the previous year. Due to the lack of state identifiers and the small sample sizes within industries, the prevalence rates are nationally representative.
Condition categories by Clinical Classification (CC)14 Code and 3-digit ICD-9 | |
---|---|
Hypertension | |
Hypertension without heart disease | ICD-9: 401 403 |
Cardiovascular Disease (CVD) | |
Coronary heart disease | ICD-9: 410-414 |
Congestive heart failure, nonhypertensive | ICD-9: 428 |
Stroke | ICD-9: 430 431 433 434 436 438 |
Other CVD | ICD-9: 390-400 402 405-409 415-427 429 432 435 437 439 440-459 745-747 |
Diabetes | ICD-9: 250 |
Dyslipidemia | ICD-9: 272 CC: 53 |
Arthritis | ICD-9: 274 354 390 391 443 446 710-716 719-721 725-729 |
Asthma | ICD-9: 493 CC: 128 |
Depression | ICD-9: 296 311 |
Injuries | CC: 225-244 |
COPD | CC: 127 |
Other MHSA | CC: 66 67 69-73 or (no Depression and CC: 650-652 654-660 662) |
Back Problems | CC: 205 |
13. Who developed this tool?
This tool was developed by RTI International and supported by the Centers for Disease Control and Prevention (CDC), the CDC Foundation, and the Northrop Grumman Corporation.
14. Why was the tool developed?
Obesity is a growing trend affecting workers all over the world. Many organizations realize the need to assess the costs of obesity as it relates to their bottom line. Forward thinking organizations are looking for ways to quantify the magnitude of this challenge and to use this information to consider the options and benefits of providing interventions and incentives to better manage the health of their employees.
The Obesity Cost Calculator is designed to use input data provided by human resources or benefits personnel to calculate an estimate of the costs to an organization that are obesity related.
Also in This Section
Microsoft
Word
Description: Microsoft Word is a word processing program
used to create and edit text documents. Text in Word documents can be easily
modified or copied for use in other applications.
File extensions: .doc, .rtf
Viewing: If you do not already have Word, you can
download Word Viewer for free*.
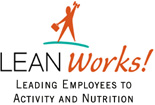
Contact Us:
- Centers for Disease Control and Prevention
1600 Clifton Rd
Atlanta, GA 30333 - 800-CDC-INFO
(800-232-4636)
TTY: (888) 232-6348
24 Hours/Every Day - cdcinfo@cdc.gov