Containerized Cargo Shipper's Behavior in China:
A Discrete Choice Analysis
Piyush Tiwari*
Infrastructure Development
Finance Co., Ltd.
Hidekazu Itoh*
Masayuki Doi*
University of Tsukuba
ABSTRACT
Shippers choose ports for export or import of goods based on a number of variables, including location, preferences for particular shipping line services, and facilities offered. A huge port infrastructure investment is necessary to attract shippers, and ports compete with each other for business. This paper models the port and shipping line choice behavior of shippers in China, using a shipper-level database obtained from a 1998 survey of containerized cargo shippers. We used a discrete choice model where each shipper chooses among 10 shipping line and port combinations and makes decisions based on various shipper and port characteristics. This paper incorporates the shipping line choice behavior through model specification by nesting the choices in a hierarchical fashion where shippers choose from Chinese and non-Chinese shipping lines and then from ports or vice versa. The results indicate that the distance of the shipper from the port, the number of ship calls at the port, the efficiency of the port infrastructure, and the number of routes offered at the port strongly influence decisions to use a port.
INTRODUCTION
Shippers choose shipping lines and ports through which their goods can be moved reliably and economically, particularly for containerized high value- added cargo. In an earlier study (Tiwari et al. 2003), we modeled the choice of ports and shipping lines by light manufacturing industrial cargo shippers in China. Our study emphasized the importance of joint modeling the choice of ports and shipping lines.
Policy changes in China during the last two decades (e.g., economic liberalization and trade, the unification of mainland China and Hong Kong, and membership in the World Trade Organization) have strengthened the role of ports in the economy. Ports and shipping lines play a key role in the export and import of goods. Imports constituted 17.5% of the Chinese gross domestic product (GDP) and exports were 21.9% of the GDP (US$) in 1998.
China has 16 major coastal ports (SSB Annual).
Figure 1 shows the location of the key ports. The ports of Shanghai and Qingdao are relatively close to each other and are more likely to compete with one another than more distant ports. The ports of Tianjin and Dalian compete against one another as they are close to Beijing. In 1998, the port of Shanghai, the largest containerized cargo handler in China, handled 28.4% of this cargo, measured in 20-foot equivalent units (TEUs). Port shares for Dalian, Tianjin, and Qingdao in total TEUs were 4.9%, 9.5%, and 11.3%, respectively. Other ports in China handled about 45.9% of the total cargo in TEUs (Year Book House of China 1999; SSB Annual).
Chinese ports have started to compete among themselves, including traditionally busy ports such as Hong Kong. Ports in South China that are closer to cargo origins are growing by more than 40%, while Hong Kong, which depends on South China for about 80% of its cargo, is seeing only single-digit increases (Mongeluzzo 2002).
China, an emerging market economy, is in the process of formulating policies intended to make its ports more efficient. The port sector in China has undergone many changes during the last decade and is poised for significant gains in the future. Key areas being considered include strengthening of port infrastructure and issues related to the market entry or exit of ports and related services and shipping lines. It is essential to quantify the impact that changes in key port infrastructure or shipping line variables would have on the demand for port and shipping line services.
This paper attempts to fill the gap in the literature on this topic by formulating a model of port and shipping line choice decisions by shippers in China. Specifically, the model seeks to specify and empirically estimate the underlying factors that influence the port and shipping line selection behavior of containerized cargo shippers in China. The model estimates market elasticities that measure changes in market shares of various ports and shipping lines due to changes in key infrastructure variables.
This study is important for two reasons. First, it supplements the currently limited amount of literature available on port and shipping line choice behavior of shippers, particularly for China. Second, as nations become more "global" and their industries are affected by the pressures of international competition, services must be provided on an internationally competitive basis. Thus, port authorities must understand the necessity of improving their services in order to respond to greater competition among ports and the growing pressure from shippers for lower port and shipping charges.
Ports form a vital link in the overall trading chain and, consequently, their level of utilization determines to a large extent their domestic and international competitiveness. In order to maintain a competitive edge in these markets, port authorities must understand the underlying factors that affect their competitiveness.
The objectives of this paper are to 1) estimate the demand for ports and shipping lines in China, 2) determine what factors affect the demand for ports and shipping lines, and 3) estimate the market elasticity parameters of demand. This analysis covers shippers from most of the Chinese coastal regions but excludes Guangdong and Hainan provinces, which ship most of their cargo from the ports of Hong Kong and Yantian, because these provinces are not covered by our dataset.
The paper is structured as follows. The next section briefly reviews the literature, followed by a section describing our model. Next, we present key statistics based on the study data, and the final two sections discuss the results and conclusions.
LITERATURE REVIEW
Earlier literature in this area focused on analyzing the performance of shipping lines or factors influencing the choice of ports. Our earlier study (Tiwari et al. 2003) hypothesized that shippers choose shipping lines and ports simultaneously. This is important because most earlier studies are based on the assumption that shippers' deal with forwarders and base their decisions only on service factors. The port and shipping line choices would then be made by forwarders on behalf of shippers. This is not always the case in Asia. Shippers base their logistic decisions on an overall cost-minimizing strategy. They choose shipping lines and ports so that their goods can be shipped economically and efficiently to market locations. Although our earlier study emphasized the need for direct modeling of shipping line and port choice by shippers, our empirical results only weakly supported the hypothesis. In this analysis, we include all types of containerized cargo to and from China (extending the Tiwari et al. database) and test a hypothesis similar to that proposed in our earlier study.
Prior research analyzing factors responsible for port and shipping line efficiency guided our choice of variables for this study. Network and scale economies affect shippers' choice of ports. Studies estimating port performance indicate that the number of ship calls is an important factor in determining the performance of a port. The speed at which cargo moves through a port influences its value.
Slack (1985) analyzed port end users and freight forwarders engaged in trans-Atlantic container trade between the United States and Europe and found that the number of sailings was the most important criterion for port choice. Bird's (1988a and 1988b) results from perception analysis of European freight forwarders indicate that the frequency of ship service is the main reason for port choice. Tongzon (1995) also confirmed that time is critical in freight forwarding and the frequency of shipping service is the major determinant of time.
Investigations of what influences the choice of shipping line have identified three categories of factors: route (e.g., frequency, capacity, convenience, directness, flexibility, and transit time), cost (freight rate and other costs), and service (delays, reliability and urgency, avoidance of damage, loss and theft, fast response to problems, cooperation between shipper and carrier, and documentation and tracing capability) (Gilmour 1976; McGinnis 1979; Ogden and Rattray 1982; Brooks 1985; Wilson et al. 1986; and Meyrick and D'Este 1989). These studies found that shippers are generally risk averse in their choice of shipping lines and thus have limited options. Service factors, particularly service frequency, take precedence over price (Bayliss and Edwards 1970; Brooks 1984, 1985; Wilson et al. 1986; Meyrick and D'Este 1989; Pearson 1980).
On the other hand, Suthiwartnarueput's (1988) study on the efficiency of the shipping industry in Thailand suggests that the most important service attribute is cost, followed by punctuality, transit times, frequency of sailings, directness of sailings, as well as past loss and damage experience. Jamaluddin (1995), with reference to the Far East/Europe trade, reported that the six service factors to which shippers
attach the most importance were freight rate, cargo care and handling, knowledgeability, punctuality, transit time, and service frequency. Furthermore, the six service attributes to which carriers
attach the most importance are knowledgeability, freight rate, cargo care and handling, punctuality, transit time, and service frequency.
Chiu (1996) evaluated the logistics performance of liner shipping in Taiwan and found that the most important service attributes for shippers
were prompt response by the carrier to problems, transit time reliability, documentation services, notice of delay, and assistance with loss or damage claims from the carrier. For carriers,
the five most important service attributes of carriers were transit time reliability, prompt response by them to problems, knowing the needs of the shippers, their own reputation, and knowledgeability of sales personnel.
Previous studies concluded that service attributes significantly influence distribution and logistics activities. Services such as transit time, frequency of service, reliability of delivery, speed of claims response, on-time pickup and delivery, as well as other aspects of physical distribution, are perceived as crucial by shippers.
MODEL AND VARIABLES
This paper extends our earlier analysis, which looked at shippers' logistics decisions for light manufacturing industrial cargo in China. That analysis was based on a multinomial logit (MNL) model in which alternatives available to shippers were combinations of ports and shipping lines. The methodology is briefly discussed below.
Shippers require a shipping line and port to move goods. In our model, because of data limitation, we considered only two groups of shipping lines (Chinese and non-Chinese). We assumed (a rather strong assumption but we explain our rationale later) that all service aspects of shipping lines are subsumed by grouping shipping lines this way. Shippers maximize profits by minimizing their cost of transportation and while doing so they will choose a combination of shipping line and port that is the most cost-effective in terms of the overall chain of production. This paper classifies choices as combinations of five ports and two shipping lines. The total number of choices available to a shipper is 10 (2 shipping lines x 5 ports)
(figure 2). We estimated the probabilities of choosing
alternative i (i = 1,...,10) using a discrete choice modeling framework.
The simplest and most convenient functional form for a discrete choice probability of alternative i is the standard MNL form (McFadden 1981).
,
where
N = {1,...,n} denotes the set of n
discrete port-shipping line choices,
Zi = a vector of K
attributes specific to choice i,
and
β = a vector of corresponding cost parameters.1
The simple MNL model is constrained by the Independence of Irrelevant Alternatives (IIA), which implies that the cross-elasticities of the probability shares must be equal (Boersch-Supan and Pitkin 1988).
McFadden (1981) generalized the MNL specification as the nested multinomial logit (NMNL) model based on a hierarchy that groups alternatives into subsets of similar choices. The choice within a cluster and the choices among the clusters within each nest are described by a conditional logit choice probability and conform to the IIA assumption. Following McFadden, this paper formulates an NMNL model with shipping lines and ports at different levels of hierarchy. Figure 2 shows the various illustrative specifications of the NMNL models considered in this paper.
Mathematically, the probability of choosing alternative ij
in the NMNL model is
P(ij) = P(i) x P(j|i)
where
i
= number of subsets (e.g., in figure 2, two subsets in NMNL Tree 1 and five subsets in NMNL Tree 2) and each subset has some or all of j
= shipping lines-port combinations (some or all of the total of 10),
P(i) = the marginal choice probability of subset i, and
P(j|i) = the conditional probability of choosing alternative j
from the alternatives included in subset i.
The conditional probabilities of choosing alternative j
in subset i
have the form of MNL choice probabilities
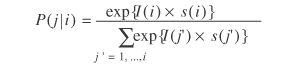
The marginal choice probability is represented as

where the "inclusive values" I
(i) is defined by
are weighted by "similarity coefficients" s
(i). These similarity coefficients refer to their respective subsets and characterize the degree of substitutability among the alternatives in the subset. Values of similarity coefficients between zero and one are a measure of the importance of similarities and dissimilarities among choices. If, however, these values are equal to one, the trees reduce to simple MNL.
This paper estimates the probability of choosing alternative i based on the following variables.
Port Characteristics
Ship calls is an important variable. The sign for this variable is ambiguous, because, as explained earlier, an increase in the frequency of ship calls is an attractive variable for exporters and importers. A positive sign may be expected for this variable. However, if the ship call variable is already high for a port, increased congestion may result and have a negative effect.
Total TEUs handled at the port
may represent the use of the port by shippers. However, a higher volume of TEUs may also represent congestion. We do not have any a priori notion about the sign of this variable.
A higher number of TEUs per berth at the port
means that one berth caters to a large number of TEUs. As will be discussed in more detail in the Results section, we believe this may represent congestion at the berth and shippers are expected to react negatively to this variable.
TEUs of cargo per crane
loaded and unloaded from a ship is also another time-related factor. As will be discussed in more detail in the Results section, we believe a higher value for this variable indicates efficiency in loading and unloading a ship's cargo. This variable is expected to influence the shippers' decisions positively.
The usage factor
is defined as the handling volume (thousand tons) per length of quay. The expected sign for this factor is negative, because a higher value for this variable represents congestion.
The number of routes offered
is another frequency of shipping-related variable. The greater the number of routes offered at a port, the quicker it is for shippers to move their goods to various destinations.
Port and loading charges is another variable that would affect the decisions of shippers. However, these charges are the same for all ports (Water Transportation Ministry 1997).
Shipping Line Characteristics
The literature in this area considers marketing to be a service factor. Advertising, frequency, and quality of service are crucial factors in determining the selection of a shipping line. Traditionally in China, forwarding and logistics have been tightly controlled. The entry of foreign companies has not been easy. Foreign companies can operate only if they have a Class A license from the Ministry of Foreign Trade and Economic Cooperation. The license strictly limits the services companies can offer and the cities or regions in which they can operate.
In a highly regulated environment, it is difficult to distinguish shipping lines on the basis of services or competitive factors. However, to capture some aspect of competition we classified shipping lines as Chinese and non-Chinese based on their flag of registration. Chinese shippers prefer Chinese shipping lines for two reasons: their business relationship has been built over a number of years, and the licensing system offers the most profitable routes to the Chinese shipping lines. Because foreign shipping lines are relatively new to the Chinese markets, cost and service data are limited. Thus, this paper tries to capture the differences in the attitudes of shippers toward shipping lines using the NMNL model.
We have good data for two characteristics related to shipping lines.
- Total TEUs handled
during the year by shipping lines. We do not have an a priori expectation regarding its sign.
- Number of vessels.
The greater the number of vessels operated by a shipping line, the lower the turnaround time for shippers' cargo. The number of vessels is highly related to frequency and timeliness and is an important service variable. We expect that it has a positive sign.
Shippers' Characteristics
The characteristics of the shippers themselves influence their decisions regarding port and shipping line choices. The variable we considered in our model is the distance of the shipper from the port.
We imputed the distance of shippers from ports based on our calculation from China's Road Map Collection
(Measurement Publishing 1994) and China's Route Map
(PTPH 2000). The farther the port is from the shipper's location, the less likely the port will be chosen. Distance is expected to be negatively related to port choice.
Cargo type also influences the choice of port and shipping line. This paper, however, considers only containerized cargo so the type of cargo is not a variable that will determine the choice of ports and shipping lines.
DATA
The data used in this paper are from the Survey of International Containerized Cargo Flows on the Yellow Sea Rim, conducted by the International Center for the Study of East Asian Development in Japan for 1998. The data do not include domestic cargo flows within China. The total sample size is 2,424 and covers shippers located in 9 provinces and 6 cities of China (Guangdong and Hainan provinces are not covered). The survey asked shippers about their choice of shipping line and port through which they export or import goods. The data report only the behavior of shippers. We supplemented these data with various port infrastructure-related variables such as number of berths and cranes, port charges, number of ship calls, routes offered, and so forth, from the China Statistical Yearbook, China Shipping Development Annual Report 1998,
and the Year Book of China Transportation & Communications.
The data indicate that the most used port in China is at Shanghai, with 4.22 million total TEUs handled in 1998. Shanghai's better infrastructure accounts for this; it has 28 cranes and 19 berths. In 1998, 9,660 ships called at the port of Shanghai, traveling on 624 routes. Qingdao and Tianjin followed Shanghai with about 1.5 million and 1.3 million total TEUs, respectively.
The data used in this paper include some characteristics of shipping lines, for example, number of vessels and TEUs handled. However, due to the confidential nature of the data, we could not obtain the names of the shipping lines. This lack of information kept us from incorporating shipping line service-related variables. Moreover, the survey provided only quantitative variable data and no information on qualitative or behavioral aspects of shipping lines. As mentioned earlier, we classified shipping lines into two groups according to their country of registration: Chinese and non-Chinese. Of those surveyed, the Chinese shipping lines had the largest fleets, with an average of 128 vessels. The total TEUs handled by these shipping lines is shown in
table 1.
RESULTS
As formulated in this paper, a shipper has a choice of 10 port-shipping line combinations. Based on the characteristics of shipping lines and ports, shippers choose the combinations that minimize costs. Based on model estimation trials, we retained only those variables that were significant at the 95% level, with the exception of the constant term. In our model, the constant term interacts with various alternatives to take advantage of nesting, and technically this increases the degrees of freedom. All other variables are alternative-specific.
The NMNL structure requires that we impute values not only for a combination that is chosen by the shipper but also for other choices. We imputed these values for all levels of combinations for each of the variables as averages for the variables. The results are presented in
table 2.
We tried five nesting specifications (including MNL) of the model. Though there was little difference in the performance statistics (likelihood value, McFadden's Rho-squared, and ex-post correct predictions) of MNL and NMNL specifications, theoretical reasons suggest that NMNL specification is better than MNL, because unlike MNL it is not constrained by IIA (McFadden 1981).
Among NMNL specifications, Tree 3 (figure 2) performs slightly better than other specifications, and we computed elasticity of market shares based on this specification. The value of McFadden's Rho-squared of NMNL Tree 3 is 0.4042. The ex-post percentage of correct prediction is 58.75%. The dissimilarity coefficients of all NMNL trees differ from one for different levels of hierarchy and are greater than one at some or all levels for all except Tree 3. This suggests that trees other than Tree 3 are inconsistent (Boersch-Supan and Pitkin 1988). Because Tree 3 shows better performance, this suggests that shippers compare Chinese shipping lines and port combinations based on shipping line and port characteristics and the ability to choose one combination conditional on the presence of other alternatives. However, among non-Chinese combinations, choices are independent of the presence of other alternatives (like MNL structure). Results indicate that the distance of the port from shippers is an important determinant of port choice. If the port is far from the shipper's location, the probability of its being chosen by the shipper decreases. In fact, distance is so important in the overall decision process of shippers that many shippers have located closer to ports. An increase in the TEUs per berth, ceteris paribus, decreases the probability of choice of that port. The TEUs per crane at a port has a positive sign, as expected, capturing the efficiency.
The estimated coefficient for TEUs per berth is negative, while the coefficient of TEUs per crane is positive. This confirms our hypothesis that, for China, TEUs per berth represent port congestion, while TEUs per crane indicate handling efficiency. The following discussion explains our hypothesis and expectations about signs.
The port of Shanghai is the largest port in China, handling more than 4 million TEUs in 1998. Furthermore, Shanghai is a river port and it suffers from accumulated silt, making it unable to accommodate large container vessels. This historically important port is located near the largest economic center of China. As mentioned earlier, Shanghai handled 222,000 TEUs per berth in 1998.
Qingdao, whose economy is partly supported by trade and direct investment from Korea, is the second largest container port. In 1998, it handled 1.5 million TEUs; however, there are only 5 container berths at Qingdao (the same number as Dalian but fewer than Tianjin). As a result, Qingdao's reported container-handling volume was 300,000 TEUs per berth. The port authority at Qingdao developed new deep-water berths relatively late compared with Tianjin or Dalian and suffered from terminal area congestion for a long time. Berth undercapacity and terminal area congestion mean other vessels cannot choose their desired port call timing and may have to wait offshore until berths are vacant. Some vessels carrying cargoes requiring quick turnover avoid such congested ports and shift to other ports that can handle 150,000 to 180,000 TEUs per berth.
The ship size allowed by the water depth, the number of berths, and limited terminal areas determine the absolute container port capacity. Congestion due to the limited port capacity is a discouraging factor.
Two variables, the number of vessels and total TEUs handled by the shipping line during the year, were dropped from our estimated models because they were not significant. As mentioned earlier, this paper does not include variables related to cost and service characteristics due to limited data. We opine that classifying shipping lines as Chinese and non-Chinese would capture essential differences.
These results, when translated into market share elasticities, present an interesting picture. The proximity of shippers to ports plays an important role in determining their choices. Market share elasticities are useful for port planning and shipping line operations and, in turn, lead to economic development through advantages to shippers. Market share elasticities with respect to shipper-port distance differ depending on shipping lines and ports. Non-Chinese shipping line users tend to stay with the ports of their choice more than Chinese shipping line users, presumably because non-Chinese shipping line calls are limited to certain ports.
If a shipper is located far away from a port, ceteris paribus,
preference for that port is low. The magnitude of decrease in market share relative to the shipper's distance from ports also depends on the chosen shipping line.
Table 3 presents distance elasticities and shows how the market share of ports changes if the shipper's distance from the port increases by 1%. For example, if the distance of a shipper from Dalian increases by 1% and if the shipper uses Chinese shipping lines (row 1), the market share of this combination is reduced by 6.2% (column 1), while the market shares of all other port-shipping line combinations (columns 25) increase by 0.28%; for other port-shipping line alternatives with non-Chinese shipping lines (columns 610) the increase is 0.26%. Moreover, if shippers using non-Chinese shipping lines to move goods through Dalian (row 6) change their location and the distance from this port increases by 1%, the market share of the Dalian-non-Chinese shipping line combination decreases by 5.9% (column 6), while the market shares of all other port-shipping lines combinations increase by 0.83% (columns 710).
Distance elasticities of market shares are high for new and secondary ports, and Dalian and Qingdao have the potential to increase their shares by improving their accessibility. On the other hand, Shanghai tends to retain its shippers due to economic maturity and related logistic functions achieved during its long port history.
As table 3 shows, ports most affected by distance increases are the "other ports," whose market share decreases by a large percentage. Of all ports surveyed, Tianjin is the least affected. Furthermore, Chinese shipping lines are the most affected. These elasticity estimates are smaller in magnitude than we estimated in our earlier study.
Table 4 presents market share elasticities for TEUs per berth. These numbers indicate the changes in the port-shipping line market shares shown in the column with respect to a 1% increase in TEUs per berth at a port. The elasticity values are highest for Qingdao, followed by Shanghai. Although Qingdao is the second largest container handling port, congestion is a serious problem. However, Qingdao's congestion relief efforts and efficiency improvement through port area development and other measures can be expected to enlarge its share.
The elasticity of market shares for TEUs per crane (table 5) indicates that with an increase of TEUs per crane, Qingdao's market share will increase the most followed by Shanghai.
Table 6 shows that with an increase in the number of routes offered at a port, Shanghai would gain the most. With an increase in ship calls, however, Shanghai loses the most, because, as mentioned earlier, Shanghai is served by the largest number of routes
(table 7).
These results are interesting and indicate that after Shanghai, Qingdao is experiencing higher levels of use and now faces congestion problems. Qingdao's cargo handling services are efficient and an increase in the routes offered and improved infrastructure facilities would bring more cargo to this port.
Dalian has a new container terminal and the results indicate that its market share is less elastic than the other major ports with respect to congestion. Since its market share elasticity with respect to routes is second highest after Shanghai, Dalian is expected to experience increased demand due to greater economic activity and ship calls.
In sum, secondary and new ports other than Shanghai have the potential to increase their market shares through accessibility improvements, congestion relief, and route diversification. Addressing these challenges could make the Chinese port system more efficient and further increase inter-port competition.
CONCLUSION
China's acceptance into the World Trade Organization has generated significant interest in the forwarding and logistics services sector. Demand has grown for quality forwarding and logistics services, better services at ports, and upgraded port infrastructure.
Newer Chinese ports are starting to compete with older, more established ports. For example, newer ports on the southeastern coast of China have challenged the traditional monopoly of Hong Kong (80% of the cargo leaving Hong Kong comes from South China). Although this paper does not include Hainan and Guangdong provinces, where a lot of the Chinese cargo going to the port of Hong Kong originates, ports in these regions compete with Hong Kong.
China is a country of cargo originators, and the international competitiveness of its goods depends on cost-effective and efficient shipment. With a view to attract more cargo, Chinese ports are competing with each other.
The analysis of shippers' choice of shipping lines and ports is essential for policy formulation related to improving port infrastructure and services, in addition to market entry/exit decisions of shipping lines. This paper models the shipping line and port choice behavior of Chinese shippers. This is one of the few studies that models this behavior using an empirical model, and it may be the first study that models the joint choice of shipping lines and ports in China.
The data used in this paper are unique and from a survey of shippers conducted by the International Center for the Study of East Asian Development in Japan for 1998. Results indicate that Chinese shippers and forwarders are conservative and prefer Chinese shipping lines primarily because of long-established relationships and the availability of larger fleets. The shippers are indifferent to foreign shipping lines, but choose them based on the ports from which they would like to import or export their cargo. However, foreign shipping lines have only recently started operating in China and a change in the behavior of Chinese shippers can be expected in near future.
Of the variables we studied, high TEUs per berth indicate congestion and negatively affect shippers' decisions. High TEUs per crane indicate efficiency in moving cargo and show a positive coefficient. The distance of a port from a shipper's location and the number of ship calls at a port are important variables that determine the choice of a port. Distance and ship calls both have negative elasticity. Ports offering more routes have higher market share elasticity. Another variable that affects the choice of port is the handling value (thousand tons of cargo per meter of quay). This variable also captures congestion and has a negative coefficient.
ACKNOWLEDGMENTS
The authors would like to express their sincere gratitude to two anonymous referees whose comments helped in substantially improving the quality of this paper.
REFERENCES
Bayliss, B.T. and S.L. Edwards. 1970. Industrial Demand for Transport.
London, England: Ministry of Transport.
Bird, J. 1988a. Freight Forwarders Speak: The Perception of Route Competition via Seaports in the European Communities Research Project (Part I). Maritime Policy and Management
15(1):3555.
_____. 1988b. Freight Forwarders Speak: The Perception of Route Competition via Seaports in the European Communities Research Project (Part II). Maritime Policy and Management
15(2):107125.
Boersch-Supan, A. and J. Pitkin. 1988. On Discrete Choice Models of Housing Demand. Journal of Urban Economics
24(2):153172.
Brooks, M.R. 1984. An Alternative Theoretical Approach to the Evaluation of Liner Shipping: Part 1. Situational Factors. Maritime Policy and Management 11:3543.
_____. 1985. An Alternative Theoretical Approach to the Evaluation of Liner Shipping: Part 2. Choice/Criteria. Maritime Policy and Management
12:145155.
Chiu, R.H. 1996. Logistics Performance of Liner Shipping in Taiwan, Ph.D. dissertation. Department of Maritime Studies and International Transport, University of Wales, College of Cardiff, UK.
Gilmour, M.R. 1976. Some Policy Implications of Subjective Factors in the Modal Choice for Freight Movements. The Logistics and Transportation Review
12:3957.
International Centre for the Study of East Asian Development. 1999. Survey of Cargo Flows in the Yellow Sea Rim (in Japanese). March.
Jamaluddin, T.T.M.S. 1995. Marketing of Freight Liner Shipping Services with Reference to the Far East-Europe Trade: A Malaysian Perspective", Ph.D. dissertation, Department of Maritime Studies and International Transport, University of Wales College of Cardiff, UK.
McFadden, D. 1981. Econometric Models of Probabilistic Choice. Structure Analysis of Discrete Data with Econometric Applications.
Edited by C.F. Manski and D. McFadden. Cambridge, MA: MIT Press.
McGinnis, M.A. 1979. Shipper Attributes Towards Freight Transport Choice: A Factor Analytic Study. International Journal of Physical Distribution and Materials Management
10:2534.
Measurement Publishing Co. 1994. Surveying and Mapping Press
(in Chinese). Beijing, China.
Meyrick, S. and G. D'Este. 1989. More Than the Bottom Line: How Users Select a Shipping Service. Proceeding of the 14th Australian Transportation Research Forum,
Perth, Australia, pp. 6581.
Mongeluzzo, B. 2002. Spanning the Globe. The Journal of Commerce,
November 1824.
Ogden, K.W. and A.L. Rattray. 1982. Analysis of Freight Mode Choice. Proceedings of the 7th Australian Transport Research Forum,
Hobart, Australia, pp. 249276.
Pearson, R. 1980. Containerline Performance and Service Quality. Marine Transport Center, University of Liverpool, Liverpool, England.
People's Transportation Publishing House (PTPH). 2000. China's Route Map
(in Chinese). Beijing, China.
Slack, B. 1985. Containerization, Inter-Port Competition and Port Selection. Maritime Policy and Management
12(4).
State Statistical Bureau (SSB). Annual. China Statistical Yearbook
(in Chinese). Beijing, China.
Suthiwartnarueput, K. 1988. The Exploration of Sea Transport Efficiency: With a Concentration on the Case of Thailand, Ph.D. dissertation. Department of Maritime Studies and International Transport, University of Wales, College of Cardiff, UK.
Tiwari, P., H. Itoh, and M. Doi. 2003. Shippers' Port and Carrier Selection Behaviour in China: A Discrete Choice Model. Maritime Economics and Logistics
5(1).
Tongzon, J.L. 1995. Determinants of Port Performance and Efficiency. Transportation Research: Part A
29(3):245252.
Water Transportation Ministry of the P.R.C. 1997. The Rule of Port Charges (Foreign Trade)
(in Chinese). Beijing, China: People's Transportation Publishing House.
_____. 1999. China Shipping Development Annual Report 1998
(in Chinese, a part in English). Beijing, China: People's Transportation Publishing House.
Wilson, F.R., B.J. Bisson, and K.B. Kobia. 1986. Factors that Determine Mode Choice in the Transportation of General Freight. Transportation Research Record
1061:2631.
Year Book House of China Transportation & Communications. 1999. Year Book of China Transportation & Communications
(in Chinese). Beijing, China.
Address for Correspondence and End Notes
Authors' addresses: Piyush Tiwari (corresponding author), Urban Specialist, Infrastructure
Development Finance Co., Ltd., The Capital Court, 6th Floor, Olof Palme Marg, Munirka,
New Delhi 110 067 India.
Email: piyush@idfc.com.
Hidekazu Itoh, Institute of Policy and Planning Sciences, University of Tsukuba,
Tsukuba 305-8573 Japan.
Email: hito@kwansei.ac.jp.
Masayuki Doi, Institute of Policy and Planning Sciences, University of Tsukuba,
Tsukuba 305-8573 Japan.
Email: doi@sk.tsukuba.ac.jp.
KEYWORDS: China, discrete choice model, port choice, shipping.
1.
The choice probability can be derived from cost minimization of shippers by
defining Ci* =Ziβ + εi
as the stochastic cost of port-shipping line choice i
where εi
follows a type I extreme value distribution (see McFadden 1981).
|