Appendix A – Assessing Attrition Bias
- Introduction
- Attrition and Bias
- Model of Attrition Bias
- Using the Model to Assess Current Standards
- Attrition Trade-offs Assuming a Constant Relative Bias
- Using the Attrition Bias Model to Create a Standard
A. Introduction
In a randomized controlled trial (RCT), researchers use random assignment to form two groups of study participants that are the basis for estimating intervention effects. Carried out correctly, the groups formed by random assignment have similar observable and unobservable characteristics, allowing any differences in outcomes between the two groups to be attributed to the intervention alone, within a known degree of statistical precision.
Though randomization (done correctly) results in statistically similar groups at baseline, the two groups also need to be equivalent at follow-up, which introduces the issue of attrition. Attrition occurs when an outcome is not measured for all participants initially assigned to the two groups. Attrition can occur for the overall sample, and it can differ between the two groups; both aspects can affect the equivalence of the groups. Both overall and differential attrition create potential for bias when the characteristics of sample members who respond in one group differ systematically from those of the members who respond in the other.
To support its efforts to assess design validity, the What Works Clearinghouse (WWC) needs a standard by which it can assess the likelihood that findings of RCTs may be biased due to attrition. This appendix develops the basis for the RCT attrition standard. It uses a statistical model to assess the extent of bias for different rates of overall and differential attrition under different assumptions regarding the extent to which respondent outcomes are correlated with the propensity to respond. The validity of these assumptions is explored using data from a past experimental evaluation.
A key finding is that there is a trade-off between overall attrition rates and differential attrition rates such that a higher rate of overall attrition can be offset by a lower rate of differential attrition (and vice versa). For example, the bias associated with an overall attrition rate of 10% and a differential attrition rate of 5% can be equal to the bias associated with an overall attrition rate of 30% and a differential attrition rate of 2%.
Assessing design validity requires considering both overall and differential attrition within a framework in which both contribute to possible bias. An approach for doing so is developed in the next section. Under various assumptions about tolerances for potential bias, the approach yields a set of attrition rates that falls within the tolerance and a set that falls outside it. Because different topic areas may have factors generating attrition that lead to more or less potential for bias, the approach allows for refinement within a review protocol that expands or contracts the set of rates that yield tolerable bias. This approach is the basis on which WWC attrition standards can be set.
Top
B. Attrition and Bias
Both overall and differential attrition may bias the estimated effect of an intervention.6 However, the sources of attrition and their relation to outcomes rarely can be observed or known with confidence (an important exception being clearly exogenous “acts of nature,” such as hurricanes or earthquakes, which can cause entire school districts to drop out of a study), which limits the extent to which attrition bias can be quantified. The approach here is to develop a model of attrition bias that yields potential bias under assumptions about the correlation between response and outcome. This section describes the model and its key parameters. It goes on to identify values of the parameters that are consistent with the WWC’s current standards, and it assesses the plausibility of the parameters using data from a recent randomized trial
Top
1. Model of Attrition Bias
Attrition that arises completely at random reduces sample sizes but does not create bias. However, researchers rarely know whether attrition is random and not related to outcomes. When attrition is related to outcomes, different rates of attrition between the treatment and control groups can lead to biased impacts. Furthermore, if the relationship between attrition and outcomes differs between the treatment and control groups, then attrition can lead to bias even if the attrition rate is the same in both groups. The focus here is to model the relationship between outcomes and attrition in a way that allows it to be manipulated and allows bias to be assessed under different combinations of overall and differential attrition.
To set up the model, consider a variable representing an individual’s latent (unobserved) propensity to respond, z. Assume z has a N(0,1) distribution. If the proportion of individuals who responded is ρ, an individual is a respondent if his or her value of z exceeds a threshold:
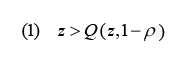
where the quantile function, Q, is the inverse of the cumulative distribution function. That is, if z is greater than the value that corresponds to a particular percentile of the z distribution (given ρ), then an individual responds at follow-up.
The outcome at follow-up, y, is the key quantity of interest. It can be viewed as the sum of two unobserved quantities, the first a factor that is unrelated to attrition (u) and the second the propensity to respond (z). The outcome can be modeled as:
where u is a random variable that is assumed to be normally distributed N(0,1),Θ is the proportion of the variation in y that is explained by z, and d takes a value of +1 or –1 to allow y to be positively or negatively correlated with z.7 Note that there are no covariates and the model assumes no effect of the treatment on the outcome. If Θ is one, the entire outcome is explained by the propensity to respond. If Θ is zero, none of the outcome is explained by the propensity to respond, which is the case when attrition is completely random.
The proportion of individuals responding at follow-up may differ by treatment status. Therefore, for treatment and control group members,
If α is the same for both treatment and control group members, then equal rates of attrition in the treatment and control groups do not compromise the causal validity of the impact because the same kind of individuals attrite from both groups.8 However, if the rates of attrition differ between the treatment and control groups, then the causal validity of the impact is compromised even when αt = αc. If αt ≠ αc, then impacts will be biased even if the attrition rate is the same in both groups because the types of students who attrite differ between the treatment and control groups.9
In this model, bias is the difference between yt and yc among respondents. It is generated by differences in the response rates (ρt and ρc) or in the proportion of the variation in y explained by z (Θt and Θc) for the two groups.
Top
2. Using the Model to Assess Current Standards
The inputs to the model are the parameters Θt, Θc, δt, δc, ρt, and ρc. With values chosen for the parameters, the model yields outcomes and estimates of bias once the two random variables z and u are given values.
Using a program written in R, 5,000 draws of zt, zc, ut, and uc were created and inserted into the model. For each individual, follow-up response (0 or 1) was then determined using equation (1), and the outcome was determined using equation (2).
Bias is the difference in mean outcomes between treatment and control respondents. Table A1 reports bias in effect size units for various assumptions about the parameters. The key finding in this table is that given a set of assumptions regarding the correlation between outcomes and the propensity to respond (these assumptions vary by column), bias can be reduced by either increasing the overall response rate or reducing the differential response rate. For example, column 4 shows that an overall response rate of 60% yields a bias of 0.05 only if the differential rate is 2% or less, but that if the overall rate is 90%, the differential rate can be as high as 5%.
TABLE A1
Bias by Response Rate and Proportion of Outcome Explained by Response (Effect Size Units)
|
(1) |
(2) |
(3) |
(4) |
(5) |
(6) |
(7) |
(8) |
ρt |
ρc |
αt = 0.075
αc =0.05 |
αt = 0.10
αc = 0.05 |
αt = 0.15
αc = 0.05 |
αt = 0.20
αc = 0.15 |
αt = 0.30
αc = 0.20 |
αt = 0.50
αc = 0.20 |
αt = 1.00
αc = 1.00 |
αt = 1.00
αc = -1.00 |
0.900 |
0.900 |
0.01 |
0.02 |
0.03 |
0.01 |
0.02 |
0.05 |
0.00 |
0.39 |
0.890 |
0.970 |
0.02 |
0.03 |
0.04 |
0.03 |
0.04 |
0.07 |
0.03 |
0.39 |
0.875 |
0.925 |
0.03 |
0.04 |
0.06 |
0.05 |
0.06 |
0.10 |
0.08 |
0.39 |
0.865 |
0.935 |
0.04 |
0.05 |
0.07 |
0.06 |
0.08 |
0.12 |
0.12 |
0.39 |
0.850 |
0.950 |
0.05 |
0.06 |
0.08 |
0.08 |
0.10 |
0.15 |
0.17 |
0.38 |
|
|
|
|
|
|
|
|
|
|
0.800 |
0.800 |
0.02 |
0.03 |
0.06 |
0.02 |
0.03 |
0.09 |
0.00 |
0.70 |
0.790 |
0.810 |
0.02 |
0.04 |
0.07 |
0.03 |
0.05 |
0.11 |
0.03 |
0.70 |
0.775 |
0.825 |
0.04 |
0.05 |
0.08 |
0.05 |
0.07 |
0.13 |
0.07 |
0.70 |
0.765 |
0.835 |
0.04 |
0.06 |
0.09 |
0.06 |
0.09 |
0.15 |
0.10 |
0.70 |
0.750 |
0.850 |
0.05 |
0.07 |
0.10 |
0.08 |
0.11 |
0.18 |
0.15 |
0.70 |
|
|
|
|
|
|
|
|
|
|
0.700 |
0.700 |
0.02 |
0.05 |
0.08 |
0.03 |
0.05 |
0.13 |
0.00 |
0.99 |
0.690 |
0.710 |
0.03 |
0.05 |
0.09 |
0.04 |
0.06 |
0.15 |
0.03 |
0.99 |
0.675 |
0.725 |
0.04 |
0.07 |
0.10 |
0.06 |
0.09 |
0.17 |
0.07 |
0.99 |
0.665 |
0.735 |
0.05 |
0.07 |
0.11 |
0.07 |
0.10 |
0.19 |
0.10 |
0.99 |
0.650 |
0.750 |
0.06 |
0.09 |
0.13 |
0.09 |
0.12 |
0.21 |
0.15 |
0.99 |
|
|
|
|
|
|
|
|
|
|
0.600 |
0.600 |
0.03 |
0.06 |
0.11 |
0.04 |
0.06 |
0.17 |
0.00 |
1.29 |
0.590 |
0.610 |
0.04 |
0.07 |
0.12 |
0.05 |
0.08 |
0.18 |
0.03 |
1.29 |
0.575 |
0.625 |
0.05 |
0.08 |
0.13 |
0.07 |
0.10 |
0.21 |
0.07 |
1.29 |
0.565 |
0.635 |
0.06 |
0.09 |
0.14 |
0.08 |
0.12 |
0.23 |
0.10 |
1.29 |
0.550 |
0.650 |
0.07 |
0.10 |
0.15 |
0.10 |
0.14 |
0.25 |
0.15 |
1.29 |
But what assumptions are appropriate regarding the extent to which response is related to outcome (the magnitudes of α coefficients that vary across the columns of Table A1)? We could infer possible appropriate assumptions from existing studies if we could somehow measure the extent of differences in outcomes between respondents and nonrespondents, and whether those differences are themselves different between the treatment and control groups. We could then compare those observed differences to what those differences would be for different values of αt and αc using our model of attrition. Of course, we cannot do this directly, because we do not observe outcomes for nonrespondents. However, in studies that have both follow-up and baseline test scores, we can use the baseline test scores as proxies for the follow-up test scores.
The example used here is Mathematica’s evaluation of education technology interventions. The evaluation had overall response rates above 90% for its sample and almost no differential response, which means that it is close to the first line of Table A1 (equal response rates of 90% in the groups). The study’s data allow calculations of differences in baseline test scores for follow-up respondents and nonrespondents. Baseline test scores are highly correlated with follow-up test scores, which means the baseline scores can proxy for follow-up scores.
The education technology study had four interventions that were implemented in four grade levels (first, fourth, sixth, and ninth) that essentially operated as distinct studies. Overall effect size differences between respondents and nonrespondents for the four study components were 0.41, 0.44, 0.51, and 0.23, an average of 0.40. The differences between the treatment and control groups in these respondent-nonrespondent differences were 0.10, 0.11, 0.10, and 0.10.
Table A2 shows the difference in effect size units between respondents and nonrespondents, and the difference in that difference between the treatment and control groups for the same α assumptions as in Table A1, but restricting attention to the case of 90% response and no differential response (the same rates observed in the education technology data). In Table A2, the closest match for the respondent-nonrespondent difference of 0.40 is found in the first column, in which the difference is 0.49. The closest match for the treatment-control difference in the respondent-nonrespondent difference is also in the first column, in which the difference-in-difference is 0.10. In other words, in the education technology study, response had little correlation with the baseline test score (our proxy for the study’s outcome measure), and this correlation did not differ significantly between the treatment and control groups.
TABLE A2
Overall Differences Between Respondants and Nonrespondants and the Difference in
That Difference Between the Treatment and Control Groups in the Case of
90% Response Rate and no Differential Attrition
|
(1) |
(2) |
(3) |
(4) |
(5) |
(6) |
(7) |
(8) |
|
αt = 0.075
αc = 0.05 |
αt = 0.10
αc = 0.05 |
αt = 0.15
αc = 0.05 |
αt = 0.20
αc = 0.15 |
αt = 0.30
αc = 0.20 |
αt = 0.50
αc = 0.20 |
αt = 1.00
αc = 1.00 |
αt = 1.00
αc = -1.00 |
Difference between
all respondents
and
all nonrespondents |
0.49 |
0.52 |
0.60 |
0.81 |
0.97 |
1.12 |
1.95 |
0.00 |
Difference between
the
treatment and
control
groups
in the
difference
between
respondents and nonrespondents |
0.10 |
0.18 |
0.32 |
0.12 |
0.20 |
0.50 |
0.00 |
3.90 |
Intuitively, this conclusion is reasonable because students were not likely to attrite from the study because of their treatment or control status. The classroom was randomly assigned to use or not use a technology product and students had no discretion. Attrition in the education technology evaluation is more likely related to family mobility because of both the students’ age and the nature of the intervention. However, for other populations of students, such as older students who volunteer to participate in a dropout prevention program, attrition may be more correlated with the outcome.
Top
3. Attrition Trade-offs Assuming a Constant Relative Bias
The trade-off between response rates can be illustrated graphically by assuming a threshold degree of tolerable bias and examining values of overall and differential response that exceed or fall below the threshold. Figure A1 uses a bias threshold of 0.05 standard deviations of the outcome measure. The green region shows combinations of overall and differential attrition that yield attrition bias less than 0.05 under pessimistic (but still reasonable) assumptions (column 4 in Tables A1 and A2), the yellow region shows additional combinations that yield attrition bias less than 0.05 under the most optimistic assumptions (column 1 in the tables), and the red region shows combinations that yield bias greater than 0.05 even under the most optimistic assumptions.
FIGURE A1
Trade-offs Between Overall and Differential Attrition
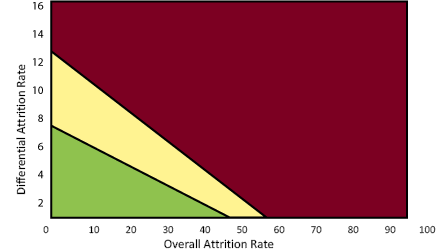
The model shows that both the overall attrition rate and the differential attrition rate can be viewed as contributing to bias, and it illuminates a relationship between the two rates. Operationalizing a standard requires choosing an appropriate degree of bias. There is no right or wrong answer to the amount of bias that can be tolerated. Empirically, the WWC would accept as evidence of effectiveness a study that reported an effect size of 0.25 that was statistically insignificant even though the true effect of the intervention might be as low as 0.20 (the WWC deems an effect size of 0.25 to be substantively important and factors this into its ratings for studies that meet standards).
To get some indication of how large the relative bias is, note that for a nationally normed test, a difference of 0.05 represents about 2 percentile points for a student at the 50th percentile. For example, if the reported effect suggests the intervention will move the student from the 50th percentile to the 60th percentile (a 0.25 effect size), the true effect may be to move the student from the 50th percentile to the 58th percentile (a 0.20 effect size). Doubling the tolerable bias to 0.10 means that an intervention that reportedly moves a student from the 50th percentile to the 60th percentile may move the student only to the 56th percentile. A relative bias of 67% (with a true effect of an increase of 6 percentile points and a reported effect of an increase of 10 percentile points, the bias would be 4 percentile points) seems large.
Top
4. Using the Attrition Bias Model to Create a Standard
In developing the topic area review protocol, the principal investigator (PI) considers the types of samples and likely relationship between attrition and student outcomes for studies in the topic area. When a PI has reason to believe that much of the attrition is exogenous—for example, parent mobility with young children—more optimistic assumptions regarding the relationship between attrition and outcome might be appropriate. On the other hand, when a PI has reason to believe that much of the attrition is endogenous—for example, high school students choosing whether to participate in an intervention—more conservative assumptions may be appropriate. The combinations of overall and differential attrition that are acceptable given either optimistic or conservative assumptions are illustrated in Figure A1, and translate into evidence standards ratings:
- For a study in the green area, attrition is expected to result in an acceptable level of bias even under conservative assumptions, which yields a rating of Meets Evidence Standards.
- For a study in the red area, attrition is expected to result in an unacceptable level of bias even under optimistic assumptions, and the study can receive a rating no higher than Meets Evidence Standards with Reservations, provided it establishes baseline equivalence of the analysis sample.
- For a study in the yellow area, the PI’s judgment about the sources of attrition for the topic area determine whether a study Meets Evidence Standards. If a PI believes that optimistic assumptions are appropriate for the topic area, then a study that falls in this range is treated as if it were in the green area. If a PI believes that conservative assumptions are appropriate, then a study that falls in this range is treated as if it were in the red area.
To help reviewers implement this standard, the WWC needs to develop a simple formula to determine whether a study falls in the red, yellow, or green region for a topic area. The inputs to this formula will be the overall and differential attrition rates, which are already collected by WWC reviewers. When entire school districts are lost from a study due to clearly exogenous “acts of nature,” the attrition standard will be applied to the remaining districts (that is, the districts lost due to the act of nature will not count against the attrition rate). Future considerations may include attrition in multilevel models.
6 Throughout this paper, the word bias refers to a deviation from the true impact for the analysis sample. An alternative definition of bias could also include deviation from the true impact for a larger population. We focus on the narrower goal of achieving causal validity for the analysis sample because nearly all studies reviewed by the WWC involve purposeful samples of students and schools.
7 In a regression of y on z, Θ would be the regression R2.
8 Those who attrite, nonetheless, will differ systematically from those who do not attrite, which possibly creates issues for external validity.
9It is possible that a difference in the rate of attrition between groups could offset a difference between αt and αc. However, throughout this appendix, we conservatively assume the opposite—that these differences are reinforcing, not offsetting.
Top