| |
| NeuroRx. 2005 October; 2(4): 541–553. | PMCID: PMC1201314 |
Copyright © 2005, The American Society for Experimental neuroTherapeutics, Inc. Medicinal Chemical Properties of Successful Central Nervous System Drugs Hassan Pajouhesh* and George R. Lenz† *Department of Medicinal Chemistry, Neuromed Technologies Inc., Vancouver, British Columbia, Canada V6T 1Z4 †GRLEN R&D Associates, Andover, Massachusetts 01810-5402 |
Abstract Summary: Fundamental physiochemical features of CNS drugs are related to their ability to penetrate the blood-brain barrier affinity and exhibit CNS activity. Factors relevant to the success of CNS drugs are reviewed. CNS drugs show values of molecular weight, lipophilicity, and hydrogen bond donor and acceptor that in general have a smaller range than general therapeutics. Pharmacokinetic properties can be manipulated by the medicinal chemist to a significant extent. The solubility, permeability, metabolic stability, protein binding, and human ether-ago-go-related gene inhibition of CNS compounds need to be optimized simultaneously with potency, selectivity, and other biological parameters. The balance between optimizing the physiochemical and pharmacokinetic properties to make the best compromises in properties is critical for designing new drugs likely to penetrate the blood brain barrier and affect relevant biological systems. This review is intended as a guide to designing CNS therapeutic agents with better drug-like properties. Keywords: CNS drug, ADME (absorption, distribution, metabolism, and excretion), physicochemical properties, pharmacokinetic properties, blood-brain barrier (BBB), QSAR |
INTRODUCTION The blood-brain barrier (BBB) and its penetration by neurotherapeutics was the subject of a recent issue of this journal. 1 This series of articles primarily had a biological orientation. From a medicinal chemical perspective, the ability to design drugs capable of penetrating the BBB and effecting the desired biological response is a formidable challenge. On the other hand, peripherally acting drugs need to possess specific physical-chemical properties that prevent them from crossing the BBB. In the broadest sense, moderately lipophilic drugs cross the BBB by passive diffusion and the hydrogen bonding properties of drugs can significantly influence their CNS uptake profiles. Polar molecules are generally poor CNS agents unless they undergo active transport across the CNS. Size, ionization properties, and molecular flexibility are other factors observed to influence transport of an organic compound across the BBB. 2 Additionally, an effective drug possesses an appropriate pharmacokinetic profile. One of the major consequences of inadequate pharmacokinetics of both developmental and marketed drugs is failure in advanced development and/or market withdrawal. 3Before the introduction of high-throughput screening (HTS) and combinatorial screening libraries, most screening for new CNS drugs was performed using biochemical methods, followed quickly by descriptive animal studies that could indicate whether or not a compound penetrated the BBB. A lead compound would then be profiled against receptor panels to look for selectivity. For instance, a synthetic derivative ABT-594, a very potent nonopiate analgesic, 4 derived from the Central American tree frog compound epibatidine, 5 was profiled against more than 70 receptors and ion channels before clinical investigation. With the introduction of HTS and subsequent huge combinatorial libraries to feed it, whole animal testing has been even further removed from the initial discovery process. Adding in informatics, genomics, and proteomics to the discovery process makes animal testing even more remote from the initial discovery process. In fact, significant resources can be invested in a lead compound and series before even whole cell assays are conducted. 6Despite the significant changes in drug discovery, multiple classes of compounds affecting CNS processes at various intervention points are successfully used clinically, and many more are in development. 7 These compounds possess tremendous chemical diversity and yet reach their target(s) in the brain. The question then becomes what physical- and medicinal-chemical characteristics do they possess that will enhance the design of new therapeutic agents at the chemistry and biology intersection. This article will focus on successful small molecule CNS therapeutics and their analogs, emphasizing their physical chemical and pharmacokinetic properties and characteristics that enable them to passively cross the BBB. We acknowledge that active transport and efflux are additional important mechanisms for transport in the CNS. |
BLOOD-BRAIN BARRIER The interface between the blood and an organ is mediated by endothelial cells that control the transfer of, inter alia, drugs from the blood stream into the cell and also from the cell to the blood. Basically, there are three methods of transfer for small molecules that can be classified according to whether there is an energy-requiring step. Two of these processes, passive and facilitated diffusion, are concentration driven and unidirectional according to the gradient. 8 Facilitative diffusion is relatively uncommon, but glucose is transferred into the CNS by a non-energy-dependent glucose transporter. The third of these processes, active transfer, requires an energy source (ATP) and can transfer molecules via a carrier against a gradient. In a global sense, the general requirements for passive and facilitated transport are common. However, depending on the organ, the epithelial cells can have differing specifics for allowing drugs to transfer from the blood to the cells in the organ. The CNS, being exquisitely sensitive to many compounds in the blood and also to drugs, is designed to be very selective in what is allowed in. As such, it is certainly an outlier as is demonstrated by the lack of correlation between intestinal Caco-2 cell and BBB active efflux. 9The reason the BBB is an outlier is that BBB epithelial cells form tight junctions that effectively preclude paracellular diffusion. In addition, the cells possess few pinocytotic vesicles and lack fenestration. Therefore, BBB transfer is through transcellular diffusion through the membranes. A drug undergoing transcellular diffusion can be metabolized by a formidable battery of metabolic enzymes. For example, decarboxylation of 3-(3,4-dihydroxyphenyl)-alanine to dopamine occurs during transit. An orally active CNS drug needs not only sufficient metabolic stability to maintain integrity in the intestine and liver but also across the BBB. Alternatively, a drug can be pumped back into the blood by an active transfer process, mainly through p-glycoprotein, using an ATP efflux mechanism. 10–12On a molecular level, the BBB is not homogenous but consists of a number of partially overlapping zones contained in a highly anisotropic lipid bilayer. 13 The conformational mobility of the lipid chains is relatively low at or near the water (blood)/lipid interface and increases strongly toward the interface at the center of the bilayer. The lipid-water interface is associated with a layer of perturbed water molecules with significantly different polarization properties. Because of this, the ability of these water molecules to form hydrogen bonds with drug molecules is dramatically reduced and forms part of the desolvation process. In addition, the hydrophilic/lipophilic interface at the blood/membrane boundary consists of perturbed and bound water, charged polar lipid head moieties connected to long lipid chains. As a result, a drug approaching the BBB is confronted with a thick layer that is capable of noncovalent interactions with the drug, similarly to that of receptor but with much looser steric requirements. The majority of drug BBB penetration is through passive diffusion through the cellular membrane. How this is accomplished has been the subject of significant research. |
ABSORPTION, DISTRIBUTION, METABOLISM, AND EXCRETION PROPERTIES OF CNS DRUGS For any drug or candidate to achieve optimum therapeutic efficacy, it must possess a high degree of potency and selectivity for interaction with a biological target as well as ability to attain target tissue concentrations that are above a certain threshold value. Absorption, distribution, metabolism, and excretion (ADME) processes play a pivotal role in defining the disposition of a drug candidate, and thus its therapeutic efficacy. Optimizing the chemical structure of lead candidates with respect to the ADME processes has become an integral part of the drug discovery paradigm. 14An important ADME characteristic is simply the solubility of the drug as only the amount of drug in solution is available for intestinal absorption and blood distribution. 15 Because diffusion across the BBB is a kinetic process and the blood and brain concentrations are in equilibrium, a concentration of drug in the blood high enough to produce a concentration of drug at its receptor in the brain is critical. 16 A drug molecule’s solubility is a function of many of the physical chemical properties that we will discuss further on in relation to BBB penetration. In addition, not only their bulk properties but also the placement of the polar and nonpolar areas of the molecule and how those regions influence the inter- and intramolecular forces in the crystalline state are relevant to solubility and BBB penetration because water has to break these interactions to bring the molecule into solution. General guidelines for solubility are classified as: moderate at 10–60 μg/ml and high over 60 μg/ml. In the blood, drugs reversibly bind to proteins like albumin and α-acidic glycoprotein. 17 The amount of protein binding of CNS drugs is an important consideration. The protein binding of CNS drugs tends to be rather high. A question that needs to be answered is: as these proteins, carrying the drugs molecules, approach the polar surface of the BBB, does the interaction cause the protein to release the bound drug? Physiochemical and biological properties are fundamental properties of ADME. Early assessment of the physiochemical properties of potential CNS drugs for their ability to cross the BBB is extremely important. Retrospectively, analysis of experimental data has provided guidelines for physical properties and has been used to derive computational algorithms to predict CNS efficacy. The initial analyses of ADME properties, e.g. anesthetic agents in the late nineteenth century, focused on the partition coefficient (LogP) between water and oil, basically the lipophilicity of the compound. This has served as one of the fundamental principles for drug discovery and design. 18 However, lipophilicity is a two-edged sword. Many other drug parameters are affected by lipophilicity. High lipophilicity frequently leads to compounds with high rapid metabolic turnover 19 and low solubility and poor absorption. As lipophilicity (LogP) increases, there is an increased probability of binding to hydrophobic protein targets other than the desired one, and therefore, there is more potential for toxicity. For example, the active sites for undesirable cytochrome P450 inhibition and human ether-ago-go-related gene (hERG) K-channel blockade are hydrophobic and bind lipophilic substrates. 20Whereas LogP is useful for prediction and optimization, it is clear that other physical-chemical properties are also of potential importance. The next major advance was due to Hansch and colleagues, 21 who developed regression analyses for these properties and initiated the field of quantitative structure activity relationships (QSAR). Hansch’s initial work was based on a large set of sedative-hypnotic barbituates where he demonstrated biological activity was almost entirely due to their Log P and their rate of metabolism was linearly related to LogP. Furthermore, optimal activity is observed at LogP = 2. 22 Other ADME parameters, including cytochrome P450 (CYP450) conversion and drug duration of action, could also be analyzed using QSAR techniques, 18 although it was subsequently acknowledged that, for accuracy, data sets for individual CYPs needed to be analyzed. 23As the pharmaceutical industry evolved and HTS and combinatorial chemistry with their huge chemical libraries became the norm, the demands for qualification of molecules with drug-like profiles, many of them virtual, quickly followed. 24,25 The design and use of molecular arrays as probes for biological function (chemical genomics) led to the National Institutes of Health (NIH) Road Map project. 26 The NIH effort is designed to generate a set of drug-like molecules that are targeted to probe the intersection of chemistry and biology space, albeit dilutely. 27 The growth of computing power allowed the development of in silico approaches that are based on laboratory-derived data sets. Although ADME covers a host of activities, the remainder of this article will concentrate on the first and major barrier to drug activity, how drugs cross the BBB, and what their physical chemical and pharmacokinetic properties and characteristics are. 28 |
QSAR STUDIES The QSAR studies on CNS active drugs and their analogs have been summarized through 1988. 18 Essentially, the large number of studies primarily used the Hansch approach where the observed biological activity is correlated by variation in the physical properties and/or structural properties among the molecules in the analog set. There are a large number of descriptors that have been evaluated in the derivation of QSAR equations. The most important of these are the physical-chemical properties describing electronic characteristics, steric effects, solvent partitioning (LogP), and in a much smaller number of cases, molecular weight. The most important of these parameters is lipophilicity that, in many cases, like anesthetics, barbiturates, benzodiazepines, etc., describes the biological activity. LogP in all the cases follows a parabolic curve that reflects the ability of the molecule to exist in relative proportions both in aqueous (blood) and lipid membrane environments. Among the electronic effects, the more important are hydrogen bonding ability, dipole-dipole interactions, and charge transfer phenomena. By far, the most important is hydrogen bonding ability. These early studies underlined the importance of LogP in understanding the activity of CNS drugs and their ability to penetrate the BBB. However, these QSAR equations were designed to both understand the biological activity of drugs as well as guide synthesis of novel analogs. As such, they were limited in scope and the data, and the conclusions derived from were not used to make semiquantitative hypotheses about general rules and molecular requirements for BBB penetration beyond general observations of lipophilicity. 29 In an interesting study that proved prescient, Levin determined that rat brain permeability was determined by LogP and had a molecular weight cutoff of 400 or less. 30 In another, Young et al. 31 investigated the effect of dipole moment and LogP in a series of centrally acting H 2-histamine antagonists related to cimetidine. They proposed that, because the molecules were very polar, LogP actually describes a desolvation effect where the molecules in their hydration spheres need to undergo desolvation at the receptor to realize the hydrogen bonding and dipole interactions with the receptor, in general terms, the BBB exterior membrane. A variation of LogP was introduced by Young et al., 32 who determined that partitioning of a series of H 2-histamine antagonists was best described by a combination of a combination of partition coefficients. The data were explained by the difference between the partition coefficients as measured in octanol/water and cyclohexane water (ΔLogP). Octanol, because of its hydroxyl group, is capable of hydrogen bonding, whereas cyclohexane, being a pure hydrocarbon (lipid) is not. They concluded that the difference between the two measures ΔLogP is a measure of the hydrogen bonding ability of the molecule. However, the generality of this relationship is unclear as a similar study on a series of H 1 antagonists only showed dependence on LogP. 33 Subsequently, Goodwin et al. 34 indicated that LogP is predominantly a measure of drug volume, or surface area, plus hydrogen bond acceptor potential. Thus, both hydrogen bonding potential and drug volume contribute to permeability. These general and cited studies, formed the basis for elucidation of the mechanism of BBB penetration by drugs that followed. Because most CNS drugs have either basic (mostly) or acidic properties, or are amphiphilic, molecular charge should be a component of BBB penetration attributes. Because these drugs are weak bases or acids (compared with strong fully ionized ones like sulfuric acid and sodium hydroxide) as measured by their pKa (log of the acid ionization constant), they are in equilibrium with their uncharged neutral species. In measuring LogP, the species in the water layer may be an equilibrium mixture of charged and neutral species. However, the species that is extracted and exists in the organic layer is neutral. 35 There are rare exceptions to this, like glycine. 36 So, in effect, what is being measured is a distribution ratio. The unraveling of LogP with charged species as a function of pH is complicated. In effect, the amount of uncharged neutral species in water is critical for membrane penetration. Fischer et al., 37 in a study of comparative properties for BBB penetration, estimated pKa limits for penetration between 4 and 10. Similar conclusions were made by Palm et al. 38 but using intestinal absorption in Caco-2 cells. Through the Hansch QSAR equations, lipophilicity, hydrogen bonding, and molecular weight have been identified as being generally critical to BBB penetration. However, with different parameters these qualities are also applicable to membrane transit in other types of cells in other organs. |
COMPUTATIONAL APPROACHES TO BBB PENETRATION For a computational ( in silico) method to be useful, it needs to have four attributes 39: - Accuracy is the key attribute. In particular, it should have a minimal number of false negatives.
- Interpretability by medicinal chemists and other related scientists to answer the questions of what compound is to be made next all the way to what compounds (real or virtual) should be in an optimization or screening library.
- Speed is obvious, but there is a trade-off in the execution and accuracy, although this gap is narrowing as computing power increases.
- Robustness is a vital consideration as computational programs become widely disseminated into a much wider circle of scientists beyond the core experts.
The data set for much of the original research on BBB penetration is based on a set of more than 100 compounds whose LogBBB was determined by radiolabeled drug determinations in blood and brain of anesthetized rats. 40 This is a slow, labor intensive, and costly process but has the highest predictability. The structures of these compounds are illustrated in a review. Although these LogP values are for the BBB, it should be mentioned that calculation of general LogP values probably has the highest accuracy among computational chemistry programs, whereas calculation of LogBBB is of more limited reliability. 41Much larger data sets, on the order of many thousands of compounds, have used a classification system whereby compounds are classified as CNS active, inactive, or questionable. Generally, these databases are the World Drug Index (WDI), Comprehensive Medicinal Chemistry database (CMC), the MDL Drug Data Report (MDDR), and the Available Chemicals Directory (ACD). As Clark 39 has pointed out, the CNS active drugs are readily identifiable (although active transport may be involved), whereas the CNS inactive are more difficult to identify. This is because the drugs may actually penetrate the CNS but are either removed by efflux mechanisms or may be metabolized to inactive compounds. |
COMPUTATIONAL QSAR The initial computational approach to BBB penetration by computing LogBBB was published by van de Waterbeemd and Kansy. 42 Their data set was based on a subset of the compounds studied by Young et al. 32 Although molecules are flexible, the study used the lowest energy conformation for the calculation and required construction of a three dimensional conformationally minimized structure for each molecule. As it turned out, based on later work, this was justified. The polar surface area (PSA) and the molecular volume components were the most important descriptors, with PSA strongly predominating. 42 PSA is defined as the surface area (Å 2) occupied by nitrogen and oxygen atoms and the polar hydrogens attached to them and is strongly reflective of hydrogen bonding capacity and polarity. Both of these forces are involved as the molecule approaches the polar surface of the membrane and desolvates as it moves into the lipid portion. Although the calculated results were indicative, the training set as later demonstrated was too small to extrapolate to the general case. Abraham constructed a larger set of molecules in an effort to reliably calculate LogBBB. 43 The QSAR equation derived contains terms for excess molecular refraction, solute polarizability, hydrogen bond acidity, and basicity and molecular volume. Although the agreement between calculation and experiment is good and this procedure has been used extensively by Abraham and his group, it fails Rule 2 above as medicinal chemists will find it difficult in using these types of descriptors in designing compounds. Because the initial PSA research used the low energy conformation of each molecule and most molecules have many accessible conformations at body temperature, Palm et al. 44 developed a dynamic PSA approach whereby the set of available conformations were used and the contribution of each to the overall PSA was calculated using a Boltzman distribution thereby taking into account conformational flexibility. Based on their results in intestinal Caco-2 cells, drugs with a PSA of 60 Å 2 or less are completely absorbed, whereas those with at least 140 Å 2 are not. This approach is computationally intensive and not suitable for analyzing large libraries. As it turned out, other investigators demonstrated that a single low-energy conformation is sufficient for determining PSA. Kelder estimated LogBBB using a set of 45 compounds, mostly the same set as others used, and compared PSAs for both single lowest energy conformations as well as a Boltzman average of the 100 lowest energy conformations. 45 There was essentially no difference between the two approaches, indicating the computationally intensive conformational calculations are not required. They further used these compounds as a training set and compared a set of 776 orally active CNS drugs that had entered phase II clinical trials against a set of 1590 non-CNS drugs that had also entered phase II. Using only a single low-energy conformation of each drug for the PSA calculation gave good agreement and separation based on PSA between the CNS and non-CNS drugs. Kelder found that non-CNS drugs transported passively and transcellularly needed a PSA of 120 Å 2 or less, whereas the drugs can be targeted to the CNS with a PSA less than 60–70 Å 2. Similar conclusions were made by van de Waterbeemd based on a study of marketed CNS and non-CNS drugs. 46 Their cutoff for PSA cutoff for CNS penetration is 90 Å 2 or below and a molecular weight cutoff of 450. Clark 47 also used PSA as a measure of LogBBB using the same small data set using a single low-energy conformation. Interestingly, using only the calculated PSA did not yield the best results. Adding the calculated LogP (ClogP) to the QSAR equation gave more accurate results, essentially adding a lipophilicity term to the hydrogen bonding and polarity terms inherent in PSA. The advantage to Clark’s QSAR equation is that by using a single conformer is fast and easy to calculate LogBBB and thus predict whether a molecule may pass the BBB. This makes the equation suitable for evaluating large screening libraries. It is reasonable to speculate on the reasons that use of single low-energy conformation of a drug yields a useful estimate of LogBBB and BBB penetration. First, many, but not all, of the drugs contained in the small set do not have a great deal of structural flexibility. This limits the set of low energy conformations to a relatively compact volume. Part of this is the consequence of the optimization process that a medicinal chemist undertakes during discovery where rigidity is incorporated into the molecule to increase biological activity among other factors. Second, the BBB acts as a molecular filter and not a barrier. Some compounds need to be let in and others kept out. There is a molecular recognition event when a drug approaches the external polar face of the BBB. Given the diverse nature of the CNS drugs, this interaction is more of a physical chemical nature than steric, i.e. it does not have the stricter steric requirements that a receptor-ligand pair would have. Because of the necessity of screening very large real or virtual libraries, on the order of a million compounds or more, approaches were investigated that would significantly speed the evaluation of each compound while maintaining the accuracy. Ertl has developed a topological PSA (TPSA) approach that fits these criteria. 48 TPSA is based on dissecting the contributions of polar groups in drugs contained in the WDI. Comparison with Clark’s results demonstrated almost no difference between the two approaches. The major advantage of TPSA is that it uses a two-dimensional structure and not the computationally more intensive three-dimensional representation. This enables two to three orders of magnitude increases in throughput. Because PSA is dependent upon hydrogen bonding and donating atoms, Österberg 49 investigated simplifying the PSA calculation by incorporating hydrogen bonding descriptors in place of PSA while including ClogP. There was high correlation between the two methods, indicating the importance of hydrogen bonding and lipophilicity for LogBBB. As with TPSA, this simplification allows BBB penetration evaluation of large actual and virtual libraries. This hydrogen bonding approach was later extended and developed into a pair of rather simple rules for predicting BBB penetration: - Because hydrogen bonding is primarily associated with oxygen and nitrogen moieties in a molecule, then, if the sum of the nitrogen (N) and oxygen (O) atoms in the molecule is five or less, then the molecule has a high probability of entering the CNS.
- If ClogP –(N + O) > 0, then LogBBB is likely to be positive and the compound has a high probability of entering the CNS.
The sum of the (N + O) atoms actually measures the hydrogen bond acceptors associated with nitrogen and oxygen moieties. It should be pointed out that there are other heteroatoms in drugs that can function as hydrogen bond acceptors (HBA) and total HBA, including (N + O) would probably give a better measure. A molecular recognition event occurs between a drug and the polar end of the phospholipid membrane at the initiation of BBB penetration. This type of interaction was explicitly incorporated in a membrane interaction QSAR (MI-QSAR). In addition to the descriptors used in studies above, Hopfinger and colleagues 50 used a molecular dynamics trajectory simulation of the drugs through a synthetic membrane. Figure 1 shows acyclovir, a slow penetrator) and diazepam (a fast penetrator) going through an artificial membrane DMPC that corresponds closely with biological membranes. Acyclovir has just passed the polar end while diazepam is almost through half of the bilayer. The illustration demonstrates the path the drugs need to follow from both the side and top views. It is important to realize that the membrane is fluid and especially the lipid side chains have large degrees of freedom to move and interact and thus retard penetration by the drugs. PSA and ClogP were, as before, the most important descriptors but, added to these, were descriptors for the interaction between the drug and the phospholipid polar head and drug molecular flexibility. The polar interaction descriptor recognizes the molecular recognition event at the start of the penetration, whereas the flexibility descriptor corresponds to the ability of a drug to essentially wiggle through the array of long chain lipids in the membrane. Veber et al. 51 have found, however, that increasing flexibility hinders membrane transit. It is likely, however, that the amount of molecular flexibility will follow a parabolic curve similar to LogP where some amount facilitates transit through the membrane, but too much conformational mobility interferes with the fluid lipid side chains. 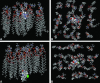 | FIG. 1.Molecular dynamics simulations of acyclovir and diazepam passive transport through a DMPC membrane. (Courtesy of Prof. A. J. Hopfinger, University of Illinois-Chicago, Chicago, IL. Copyright © 2005, The Chem21 Group, Inc., Chicago, IL. All rights (more ...) |
Hopfinger and colleagues 52 used another approach to BBB penetration in developing QSAR equations across diverse sets of molecules based on a 150-compound data set. This QSAR approach uses cluster analyses employing four-dimensional molecular similarities to partition compounds into clusters, and membrane-interaction MI-QSAR analysis to build models for each cluster. In effect, this means identifying and using common pharmacophores to group large numbers of diverse molecules into sets and building QSARs based on each set with the objective of building optimum QSAR equations for each. Provided it is computationally tractable to identify these pharmacophores in this manner, this technique should be of use in screening large libraries as it potentially contains a basis for generating relevant screening models for wide chemical diversity. |
QSAR SUMMARY Examination of the QSAR descriptors that have appeared in the various equations provides insight into the important molecular factors that govern BBB penetrations. Importantly, because these studies appeared before a summary of the same properties calculated from all the orally active drugs, numbering 74, approved since 1983 for CNS use appeared, the underlying validity of the conclusion and their molecular implications can be made. 53Lipophilicity Lipophilicity was the first of the descriptors to be identified as important for CNS penetration. Hansch and Leo 54 reasoned that highly lipophilic molecules will partitioned into the lipid interior of membranes and will be retained there. However, ClogP correlates nicely with LogBBB with increasing lipophilicity increasing brain penetration. For several classes of CNS active substances, Hansch and Leo 54 found that blood-brain barrier penetration is optimal when the LogP values are in the range of 1.5-2.7, with the mean value of 2.1. An analysis of small drug-like molecules suggested that for better brain permeation 46 and for good intestinal permeability 55 the LogD values need to be greater than 0 and less than 3. In comparison, the mean value for ClogP for the marketed CNS drugs is 2.5, which is in good agreement with the range found by Hansch et al. 22Molecular weight CNS drugs have significantly reduced molecular weights (MW) compared with other therapeutics. Levin 30 has a cutoff for BBB penetration of 400. Van de Waterbeemd 56 has suggested that MW should be kept below 450 to facilitate brain penetration and to be lower than that for oral absorption. 46 The rules for molecular weight have been reviewed, where small molecules may undergo significant passive lipid-mediated transport through the blood brain barrier, when the molecular mass is kept in or below a 400- to 600-Da range. 57 For marketed CNS drugs, the mean value of MW is 310, compared with a mean MW of 377 for all marketed orally active drug. 53Hydrogen bonding All the QSAR equations emphasize the importance of hydrogen bonding whether through polarity, PSA, hydrogen bond donor and acceptor counts, or simply counting heteroatoms capable of hydrogen bonding. All of these measurements are correlated, for instance, (O + N) atom count is highly correlated with PSA but measures hydrogen bond acceptors. CNS penetration requires a sum of these heteroatoms of 5 or less. 49 Compounds with high hydrogen bond forming potential, such as peptides with their amide groups, peptides even as small as di- or tripeptides, have minimal distribution through the BBB. 11 Increasing hydrogen bonding decreases BBB penetration. The marketed CNS drugs on average have (O + N) = 4.32, PSA = 16.3%, 2.12 hydrogen bond acceptors and 1.5 hydrogen bond donors. 53 This study indicates that polar properties of CNS drug are reduced relative to other parameters. Polar surface area PSA has been used as a predictor for BBB penetration by many investigators. 6,58 In general, drugs aimed at the CNS tend to have lower polar surface areas than other classes. 59,60 PSA for CNS drugs is significantly less than for other therapeutics with PSA for CNS penetration estimated at 60–70 Å 2 through 90 Å 2. 45,46 The upper limit for PSA for a molecule to penetrate the brain is around 90 Å 2. Molecular volume and flexibility These descriptors both need further research. Molecular volume is a function of MW and structure and takes into account all the accessible conformations available to the molecule under physiological conditions. This actually relates to rotatable bonds and the number of rings in the molecule. Rotatable bond count is now a widely used filter following the finding that greater than ten rotatable bonds correlates with decreased rat oral bioavailability. 51 CNS drugs have significantly fewer rotatable bonds than other drug classes. Most centrally acting compounds have rotatable bond count of five or less. 53 Based on the single conformation PSA calculations, it appears that the conformational range is limited in CNS drugs. Molecular flexibility relates to the ease by which the molecule transverses the membrane, and it appears that limited flexibility is advantageous but significant is disadvantageous. 52 More research is needed to determine more general and quantitative measurements. Charge Many CNS drugs are basic and exist in an equilibrium between their charged and neutral states under physiological conditions or are amphiphilic if they also possess an acidic group. Possession of a positive charge at pH 7–8 tends to favor brain permeation. 61 Additionally, compounds possessing a tertiary nitrogen (a feature of many CNS drugs) show a higher degree of brain permeation. As molecules partition into membrane lipids as neutral species, this will be a function of both the concentration of the neutral species as well as its lipophilic properties. Strong bases and acids are thereby pretty much precluded from BBB penetration, which accords well with the known difficulty that carboxylic acids have in penetrating the CNS. 62 Fischer et al. 37 put pKa limits on BBB penetration of between 4 and 10. Pharmacokinetic properties A potential drug’s pharmacokinetic properties to a significant extent can be manipulated by the medicinal chemist. However, historically, these properties have not been considered by most medicinal chemists, who in many cases used increases in receptor binding for their optimization strategy. In this section. we briefly look at the pharmacokinetic properties that impact on ADME properties of CNS drugs. These parameters include: - Metabolic stability
- Metabolic liability
- Permeability
- Protein binding
- hERG inhibition
Metabolic stability. First-pass metabolism influences oral bioavailability and toxicology of drugs. The liver and intestines are the major sites of such metabolism. Many discovery compounds exhibit low bioavailability because of high rates of metabolism. Rapid metabolism is one of the primary reasons for molecules not reaching adequate systemic levels. These are phase I cytochrome P450 oxidations or phase II conjugations. Metabolism reduces the circulating drug concentration and increases elimination. Typically, if the percentage remaining of a compound at 60 min is less than 20%, the compound considered to be metabolically unstable. Low metabolic stability results in a high clearance and short half-life of a compound. The greater than 80% remaining of drug at 60 min is considered to be ideal. 63 Metabolic liability. CYPs constitute the most important family of biotransformation enzymes involved in drug metabolism, playing an important role in the disposition of drugs and their pharmacological and toxicologicals effects. 64 It comprises a superfamily of isoforms that are vital in the metabolism of drugs. The most common used isoforms are CYP3A4, CYP2C9, CYP2D6, CYP1A2, and CYP2C19. Inhibition of CYP enzymes is the most common cause of drug interactions. Commonly a concentration of 10 or 30 μ m of drug that inhibits the metabolic activity of a given enzyme by less than 50% is considered to have an acceptable level of CYP inhibition, although the less the better. To maximize oral absorption, a successful CNS drug must have no significant CYP2D6 metabolism and be a nonpotent CYP3A4 inducer. CYP3A4 and 2D6 are of great interest because of their high probability to be involved in the metabolism of many coadministered drugs. A compound that is potent inhibitor of one of these two enzymes has a good chance of inhibiting the metabolism of coadminstered drugs, causing their plasma levels to be elevated, possibly into toxic ranges. In fact, mibefradil, the first T-type calcium channel blocker, was introduced to the market in 1997, and then abruptly withdrawn. 65 Pharmacokinetic interactions with other drugs metabolized by P450, CYP3A4, and 2D6, eventually led to withdrawal of mibefradil from the clinic. Permeability. Oral absorption of drugs is also determined by their permeability. The relative contribution of the transcellular and paracellular pathways, to overall absorption of a compound is highly dependent on its physicochemical properties of the compounds. Most relevant physicochemical properties that affect membrane permeability and are related to each other have already been mentioned. 66 However, lipophilicity (log D) and MW are the two main components that affect the permeability the most. Other factors that influence the permeability are bioisosteric replacements. 67 For example, replacement of a carboxylic acid with a tetrazole can improve permeability. Protein binding. Protein binding is a key factor for BBB penetration. CNS drugs that are normally weakly basic molecules bind to both human serum albumin (HSA) and α 1-acid glycoprotein (AGP). Depending on the drug and the target, high affinity for plasma proteins may consequently be an asset or a drawback for efficacy. HSA is the most abundant protein in blood plasma and serves as a transport protein and considered to have low affinity and high capacity for binding, whereas AGP has low capacity and high affinity for binding. 68 Drug-protein interaction is a reversible process and a successful CNS drug should not be an efficient P-glycoprotein substrate ( in vivo) and not a high-affinity serum albumin ligand (K d <10 μ m). 69 hERG inhibition. FDA concern over the risk of life-threatening arrhythmias associated with QT interval prolonging drugs has led to recommendation of more thorough preclinical and clinical cardiovascular safety assessments. In particular, most of the QT-prolonging drugs have been shown to inhibit the K + channels encoded by the hERG. 70 Drugs that interact with Ca 2+ and Na + channels have a great tendency to block hERG. For better risk assessment, a more than 30-fold margin between hERG IC 50 and effective unbound plasma concentrations are recommended for drugs in clinical development. 71 It needs to be pointed out that challenge is to minimize hERG activity to eliminate a major risk factor, but not at the expense of discarding therapeutically useful drugs that are urgently required to treat a myriad of diseases for which there is presently a paucity of quality treatments. |
COMPARATIVE CLASSIFICATION METHODS An alternative way of evaluating BBB permeation is to conduct retrospective analyses based on large databases containing qualitative data on drugs, such as the WDI, MDDR, and CMC. The approach is to compare attributes across large numbers of drugs to determine what their common attributes are and what the range of these are. Adenot extracted a large CNS library from the WDI containing approximately 1700 compounds segregated according to positive and negative BBB penetration and whether the molecule was a Pgp substrate. 72 Discrimination analysis yielded an initially surprising result. Most of the non-BBB-penetrating compounds have a number of heteroatoms larger than 8, whereas most of the BBB permeable compounds have a number of heteroatoms lower than 9. The reason that the number of heteroatoms classified most of the compounds is due to its correlation to the polar surface area. In this study, heteroatoms included oxygen and nitrogen as well as phosphorus, sulfur, and halogens, explaining the difference with Österberg’s studies. 49 This approach, however, compares two sets of drugs looking for differences. As a result, the similarities are factored out. Neural networks are being used as part of an effort to qualify compounds based on their “drug-likeness” for inclusion in large screening libraries. Sadowski and Kubinyi 73 encoded and analyzed over 200,000 molecules from WDI and ACD using topological descriptors. Neural network analysis qualified drug-likeness on non-drug-likeness success percentage of approximately 80%. A similar study by Ajay 74 used a Bayesian neural network to discriminate between drug-likeness and non-drug-likeness. Subsequently, Ajay and Murcko 75 published a study on designing libraries with CNS activity. They reported the development of a neural network trained on different sets of about 9000 compounds from CMC and MDDR drug databases separated into CNS-active and CNS-inactive drugs. They used an automated classification scheme based on therapeutic use. After training, classification of test sets of about 13,000 CNS-active and 53,000 CNS-inactive compounds from both databases was performed with approximately 80% accuracy. These neural network-based classifications appear to be practical tools for in silico screening of large compound libraries for identifying potential leads and early ADME profiling but not for high-resolution physical chemical understanding of BBB penetration. Although the previous comparative analyses are useful for large libraries, Lipinski et al. 76 at Pfizer were looking for a set of general rules that would govern drug-like properties aimed primarily at solubility and membrane penetration that could be used by medicinal chemists. Lipinski et al. noted that with the advent of HTS and combinatorial chemistry starting in the late 1980s, the trend for lead molecules identified by HTS was toward increasing molecular weight and increasing lipophilicity. Both of these contributed toward decreased solubility and membrane penetration. A database of about 2500 orally active drugs that had entered at least phase II, based on United States Adopted Name (USAN) or International Non-proprietary Name (INN) names, was used to analyze the physical chemical properties of these drugs. From this analysis, the “Rule of Five” was developed. The “Rule of Five” is so named because all the essential physical properties are parameters of five. According to this rule, a good absorption and permeability is likely if: - Molecular weight is ≤500
- Oil/water distribution coefficient (LogP) is ≤5
- Hydrogen bond donors ≤5 (expressed as the sum of OHs and NHs)
- Hydrogen bond acceptor ≤10 (expressed as the sum of Ns and Os)
A fifth rule was added later: - Number of rotatable bonds ≤10
This was originally based on rat studies 34 but is supported by the observation that drugs discontinued during the clinical development process have more rotatable bonds than those that are successfully marketed. 77 This effect may very well be a reflection of a conformationally mobile compound trying to permeate through mobile lipid chains in the bilayer. These rules were explicitly stated as not applying to actively transported substrates. If any two of the rules were exceeded, the compounds were likely to possess poor permeability and solubility properties. 78 Because, however, these properties are all correlated in individual molecules, exceptional strength in one could counteract a substandard score in another. Because Lipinski’s data set contained drugs that entered clinical trials, the probability existed that many of these failed on the path to approval. Wenlock 77 evaluated a set of marketed drugs and those that failed in clinical trials. The results are shown in Table 1. The agreement between Lipinski’s Rules (clinical candidates set) and Wenlock’s marketed drug data set is excellent. Vieth 79 also studied a set of 1193 marketed drugs with results essentially confirming those of Wenlock and Lipinski. 80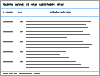 | TABLE 1. Comparison of Oral Lipinski’s Dataset and Wenlock’s Marketed Drugs Dataset* |
Lipinski 80 also laid down a set of rules derived from the set of 1500 drugs that were filtered from USAN or INN names for good CNS penetration. CNS penetration is likely if: - Molecular weight ≤400
- Log p ≤ 5
- Hydrogen bond donor ≤3
- Hydrogen bond acceptor ≤7
This study indicated that, for CNS penetration, the physical properties in general have a smaller range than general therapeutics. These “Rules” certainly confirmed the observations made independently by earlier workers. Lipinski’s contribution is that the study was based on clinically studied drugs and looked at their overall requirements not against a set of control drugs where common factors were filtered out. Lipinski’s Rule of Five has thus provided medicinal chemists with a simple mnemonic for identifying compounds with medicinally relevant physical chemical properties. As such, medicinal chemists have enthusiastically adopted the Rule of Five. 81Two studies have contrasted physical chemical properties of CNS-marketed drugs to either non-CNS drugs in general or to other classes of non-CNS drugs. Mahar Doan 82 conducted both biochemical and computational experiments on 48 CNS and 45 non-CNS drugs. The results (Table 2) did not differentiate between the two classes in molecular weight, volume, hydrogen bond acceptors, and number of aromatic rings. Significant differences were observed with hydrogen bond donors, ClogP, PSA, and molecular flexibility (rotatable bonds). In general, although there are some differences, perhaps due to the smaller data set, the results are in agreement with both laboratory and computational results. 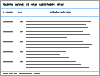 | TABLE 2. Mean (Range) of Physical Chemical Properties of CNS and Non-CNS Drugs from Mahar Doan |
An interesting study was published by Leeson and Davis 53 that used the year of market introduction of drugs to determine not only their physical chemical properties but also how they may have changed as a function of time. The time course was grouped into two parts, launched before 1983 (864 drugs) and launched between 1983 and 2002 (329 drugs). The pre-1983 was taken from Vieth’s 79 compilation and from 1983 from the last chapter in Annual Reports in Medicinal Chemistry. In contrast to all the other studies, Leeson’s was subdivided into six therapeutic areas, i.e. CNS, cardiovascular, anti-infective, etc. This study demonstrated that cardiovascular and CNS drugs were both outliers compared to the other classes (Table 3). For the 74 CNS drugs, the results show, compared with all drugs, that these drugs have statistically different (ρ = 0.001 − 0.01) means and medians in some physical chemical aspects and do not differ in others. They have significantly reduced molecular weight, polar properties %PSA [(O+N), total hydrogen bond acceptors], (OH + NH) hydrogen bond donors), and rotatable bonds (molecular flexibility). Thus, indicating that CNS drugs are smaller with a more compact and less flexible structure, and that the surface has fewer polar groups able to function as hydrogen bond donors and acceptors and that compared to the total surface area, the PSA is reduced. 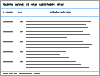 | TABLE 3. Comparison of All Marketed Oral Drugs between 1983 and 2002 and CNS and Gastrointestinal-Metabolic Drugs According to Leeson |
Interestingly, there is little difference between the physical chemical properties of all drugs and the number of rings and lipophilicity as measured by ClogP. The average ClogP for CNS drugs is 2.50, which is close to that of cardiovascular and gastrointestinal drugs but significantly different from those of anti-infective, respiratory, and inflammation, and cancer therapeutics. |
SUMMARY The requirements for passive CNS penetration by drugs is reasonably well understood, but additional work still needs to be done on the effect and optimal range of molecular flexibility on penetration. This will probably occur through additional molecular dynamics simulation of membrane transit. To function as a drug, a molecule has to meet a set of physical chemical requirements in addition to the steric and energetic requirements at its receptor. Many of these requirements are the same for both CNS and non-CNS drugs. For instance, whereas lipophilicity is important for CNS penetration, the ClogP average values for CNS and cardiovascular drugs are the same. Based on the data in the tables, CNS drugs tend to be more lipophilic, be less polar, have less flexibility, and have lower molecular weights than drugs used for other therapeutic indications. In addition, the molecular volume of CNS drugs appears to be smaller than other drugs. In terms of design of new drugs or analogs, besides the physical chemical attributes listed above, the fact that these attributes are correlated is important. Thus, changing a molecule to adjust one attribute will change others as well. This was clearly shown by a simple QSAR equation where LogP is clearly defined by molecular weight and hydrogen bond donors and acceptors. In designing new drugs, there is a balancing act required to address the physical chemical requirements and making the best compromises in properties from those available and the actuator requirements at the receptor. At the end, attributes of a successful CNS drug are shown in Table 4 and can be used as a guide to design CNS therapeutic agents with better drug-like properties. 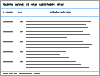 | TABLE 4. Attributes of a Successful CNS Drug |
As this article focused on passive transport of drugs into the CNS, other factors like active transport, efflux, and metabolism need to be addressed and unraveled before a potential CNS drug can enter the clinical development pathway. The entire area of active transport proteins is undergoing intensive research, and their steric and energetic requirements will be the next major area to aid in both design of new CNS drugs and the development of new and safer agents. |
REFERENCES 1. Pardridge WM. The blood-brain barrier and neurotherapeutics. NeuroRx 2: 1–2, 2005. [PubMed]2. Mouritsen OG, Jorgensen K. A new look at lipid membrane structure in relation to drug research. Pharm Res 15: 1507–1519, 1998. [PubMed]3. Lin JH, Lu A-Y. Role of pharmacokinetics and metabolism in drug discovery and development. Pharmacol Rev 49: 403–449, 1997. [PubMed]4. Bannon WW, Deceker MW, Holladay MW, Curzon P, Donelly-Roberts D, Puttfarcken PS, Bitner RS, Diaz A, Dickenson AH, Porsolt RD, Williams M, Arneric SP. Broad spectrum, non-opioid analgesic activity by selective modulation of neuronal nicotinic acetylcholine receptors. Science 279: 77–81, 1998. [PubMed]5. Daly JW, Myers CW, Whittaker N. Further classification of skin alkaloids from neotropical poison frogs (Dendrobatidae), with a general survey of toxic/noxious substances in the amphia. Toxicon 25: 1023–1095, 1987. [PubMed]6. Lenz GR. Technical problems in getting results. In: From data to drugs: strategies for benefiting from the new drug discovery technologies (Haberman AB, Lenz GR, Vaccaro DE, eds.), pp 95–114. London: Scrip Reports, 1999. 7. Williams M, Coyle JT, Shaikh S, Decker MW. Same brain, new decade: challenges in CNS drug discovery in the postgenomic, proteomic era. Annu Rep Med Chem 36: 1–10, 2001. 8. Schneeberger EE, Lynch RD. Structure, function, and regulation of cellular tight junctions. Am J Physiol (Lond) 262: L647–L661, 1992. [PubMed]9. Faasen F, Vogel G, Spanings H, Vromans H. Caco-2 permeability, P-glycoprotein transport ratios and brain penetration of heterocyclic drugs. Int J Pharm 263: 113–122, 2003. [PubMed]10. Graff CL, Pollack GM. Drug transport at the blood-brain barrier and the choroid plexus. Curr Drug Metab 5: 95–108, 2004. [PubMed]11. Pardridge WM. CNS drug design based on principles of blood-brain barrier transport. J Neurochem 70: 1781–1792, 1998. [PubMed]12. Schlosshauer B, Steuer H. Comparative anatomy, physiology and in vitro models of the blood-brain and blood-retina barrier. Curr Med Chem - Central Nervous System Agents 2: 175–186, 2002. 13. Sippl W. Computational approaches for the prediction of blood-brain barrier permeation. Curr Med Chem -Central Nervous System Agents, 2: 211–227, 2002. 14. Dennis M. Absorption processes, In: Comprehensive medicinal chemistry. Vol. 5 (Sammes PG, Taylor JB, eds.), pp 1–44. Oxford, UK: Pergamon, 1990. 15. Kerns EH, Di L. Pharmaceutical profiling in drug discovery. Drug Discov Today 8: 316–323, 2003. [PubMed]16. Faller B, Wohnsland F. Physicochemical parameters as tool in drug discovery and lead optimization. In: Testa B, Van de Waterbeemd H, Folker G, Guy R. Pharmacokinetics optimization in drug research. Zurich, Switzerland: Wiley-VCH; 257–273, 2001. 17. Ellingboe J. Application of statistical analysis techniques for discovery compound property optimization. AAPS Workshop on Optimization of Drug-Like Properties during Lead Optimization. Parsippany, NJ, 19–22 September, 2004. 18. Gupta SP. QSAR studies on drugs acting at the central nervous system. Chem Rev 89: 1765–1800, 1989. 19. Van de Waterbeemd H, Smith DA, Beaumont K, Walker DK. Property-based design: optimization of drug absorption and pharmacokinetics. J Med Chem 44: 1313–1333, 2001. [PubMed]20. Ekins S. Predicting undesirable drug interactions with promiscuous proteins in silico. Drug Discov Today 9: 276–285, 2004. [PubMed]21. Leo A, Hansch C, Elkins D. Partition coefficients and their uses. Chem Rev 71: 525–615, 1971. 22. Hansch C, Steward AR, Anderson SM, Bentley D. The parabolic dependence of drug action upon lipophilic character as revealed by a study of hypnotics. J Med Chem 11: 1–11, 1967. 23. Gao H, Hansch C. QSAR of P450 oxidation: on the value of comparing kcat and Km with kcat/ Km. Drug Metab Rev 28: 513–526, 1996. [PubMed]24. Mertsch K, Maas J. Blood-brain barrier penetration and drug development from an industrial point of view. Curr Med Chem - Central Nervous System Agents 2: 187–201, 2002. 25. Darvas F, Dorman G, Urge L, Szabo I, Ronai Z, Sasavari-Szekely M. Combinatorial chemistry. Facing the challenge of chemical genomics. Pure Appl Chem 73: 1487–1498, 2001. 26. Strausberg RL, Schreiber SL. From knowing to controlling: a path from genomics to drugs using small molecule probes. Science 30: 294–295. 27. Schreiber SL. The small molecule approach to biology. Chem Eng News, pp 51–61, 3 March 2003. 28. Ekins S, Waller CL, Swaan PW, Cruciani G, Wrighton SA, Wikel JH. Progress in predicting human ADME parameters in silico. J Pharmacol Toxicol Methods 44: 251–272, 2000. [PubMed]29. Tute MS. History and objectives of quantitative drug design. In: Comprehensive medicinal chemistry. Vol. 4 (Sammes PG, Taylor JB, eds.), pp 1–32. Oxford, Pergamon, 1990. 30. Levin VA. Relationship of octanol/water partition coefficient and molecular weight to rat brain capillary permeability. J Med Chem 23: 682–684, 1980. [PubMed]31. Young RC, Durant GJ, Emmett JC, Ganellin CR, Graham MJ, Mitchell RC, Prain H D, Roantree ML. Dipole moment in relation to hydrogen receptor histamine antagonist activity for cimetidine analogs. J Med Chem 29: 44–49, 1986. [PubMed]32. Young RC, Mitchell RC, Brown TH, Ganellin CR, Griffiths R, Jones M, Rana KK, Saunders D, Smith IR, Sore NE, Wilks TJ. Development of a new physicochemical model for brain penetration and its application to the design of centrally acting H 2 receptor antagonists. J Med Chem 31: 656–671, 1988. [PubMed]33. ter Laak AM, Tsai RS, Donné-Op den Kelder GM, Carrupt P-A, Testa B, Timmerman H. Lipophilicity and hydrogen-bonding capacity of H1-antihistaminic agents in relation to their central sedative side-effects. Eur J Pharm Sci 2: 373–384, 1994. 34. Goodwin JT, Conradi RA, Ho NFH, Burton PS. Physicochemical determinants of passive membrane permeability: role of solute hydrogen-bonding potential and volume. J Med Chem 44: 3721–3729, 2001. [PubMed]35. Taylor PJ. Hydrophobic properties of drugs. In: Comprehensive medicinal chemistry. Vol. 4: (Sammes PG, Taylor JB, eds.), pp 241–294. Oxford, UK: Pergamon, 1990. 36. Taylor PJ. Hydrophobic properties of drugs. In: Comprehensive medicinal chemistry. Vol. 4: (Sammes PG, Taylor JB, eds.), p 263. Oxford, UK: Pergamon, 1990. 37. Fischer H, Gottschlich R, Seelig A. Blood-brain barrier permeation: molecular parameters governing passive diffusion. J Membrane Biol 165: 201–211, 1998. [PubMed]38. Palm K, Luthman K, Ros J, Gråsjo J, Artursson P. Effect of molecular charge on intestinal epithelial drug transport: pH-dependent transport of cationic drugs. J Pharmacol Exp Ther 291: 435–443, 1999. [PubMed]39. Clark DE. Prediction of intestinal absorption and blood-brain barrier penetration by computational methods. Comb Chem High Throughput Screening 4: 477–496, 2001. 40. Ecker GF, Noe CR. In silico prediction models for blood-brain barrier permeation. Curr Med Chem 11: 1617–1628, 2004. [PubMed]41. Jorgenson WL. The many roles of computation in drug discovery. Science 303: 1813–1818, 2004. [PubMed]42. van de Waterbeemd H, Kansy M. Hydrogen bonding capacity and brain penetration. Chimia 46: 299–303, 1992. 43. Abraham MH. Scales of solute hydrogen-bonding: their construction and application to physicochemical and biochemical processes. Chem Soc Rev 22: 72–83, 1993. 44. Palm K, Luthman K, Ungell A-L, Strandlund G, Artursson P. Correlation of drug absorption with molecular surface properties. J Pharm Sci 85: 32–39, 1996. [PubMed]45. Kelder J, Grootenhuis PDJ, Bayada DM, Delbressine LPC, Ploemen J-P. Polar molecular surface as a dominating determinant for oral absorption and brain penetration of drugs. Pharm Res 16: 1514–1519, 1999. [PubMed]46. van de Waterbeemd H, Camenish G, Folkers G, Chretien JR, Raevsky OA. Estimation of blood-brain barrier crossing of drugs using molecular size and shape, and H-bonding characteristics. J Drug Target 6: 151–165, 1998. [PubMed]47. Clark DE. Rapid calculation of polar molecular surface area and its application to the prediction of transport phenomena 1. Prediction of intestinal absorption. J Pharm Sci 88: 807–814, 1999. [PubMed]48. Ertl P, Rohde B, Selzer P. Fast calculation of molecular surface area as a sum of fragments-based contributions and its application to prediction of drug transport properties. J Med Chem 42: 3714–3717, 2000. 49. Österberg T, Norinder U. Prediction of polar surface area and drug transport processes using simple parameters and PLS statistics. J Chem Inf Comput Sci 40: 1408–1411, 2000. [PubMed]50. Iyer M, Mishra R, Han Y, Hopfinger AJ. Predicting blood-brain barrier partitioning of organic molecules using membrane-interaction QSAR analysis. Pharm Res 19: 1611–1621, 2002. [PubMed]51. Veber DF, Johnson SR, Cheng H-Y, Smith BR, Ward KW, Kopple KD. Molecular properties that influence the oral bioavailability of drug candidates. J Med Chem 45: 2515–2623, 2002. 52. Pan D, Iyer M, Liu J, Li Y, Hopfinger AJ. Constructing optimum blood brain barrier QSAR models using a combination of 4D-molecular similarity measures and cluster analysis. J Chem Inf Comput Sci 44: 2083–2098, 2004. [PubMed]53. Leeson PD, Davis AM. Tine-related differences in the physical property profiles of oral drugs. J Med Chem 47: 6338–6348, 2004. [PubMed]54. Hansch C, Leo AJ. Substituent constant for correlation analysis in chemistry and biology. New York: Wiley, 1979. 55. Fichert T, Yazdanian M, Proudfoot JR. A structure-permeability study of small drug-like molecules. Bioorganic Med Chem Lett 13: 719–722, 2003. 56. Van de waterbeemed, Atkinson F, Cole S, Green C. Lipophilicity and other parameters affecting brain penetration. Curr Med Chem- Central Nervous System Agents 2: 229–240, 2002. 57. Hansch C, Bjorkroth J, Leo AJ. Hydrophobicity and central nervous system agents: on the principle of minimal hydrophobicity in drug design. Pharm Sci 76: 663, 1987. 58. Feng RM. Assessment of blood-brain barrier penetration: in silico, in vitro and in vivo. Curr Drug Metab 3: 647–657, 2002. [PubMed]59. Skaaeda T, Okamura N, Nagata S, Yagami T, Horinouchi M, Okumura K, Yamahita F, Hashida M. Molecular and pharmacokinetic properties of 222 commercially available oral drugs in humans. Biol Pharm Bull 24: 935–940, 2001. [PubMed]60. Abraham MH, Chadha HS, Martins F, Mitchell RC, Bradbury MW, Gratton JA. Hydrogen bonding part 46: a review of the correlation and prediction of transport properties by an LFER method: physicochemical properties brain penetration and skin permeability. Pestic Sci 55: 78–88, 1999. 61. Clark DE. In silico prediction of blood-brain barrier permeation, Drug Discovery Today 8: 927–933, 2003. [PubMed]62. Austin RP, Davis AM, Manners CN. Partitioning of ionizing molecules between aqueous buffers and phospholipids vesicles. J Pharm Sci 84: 1180–1183, 1995. [PubMed]63. Lin JH, Rodrigues AD. In vitro model for early studies of drug metabolism. In: Pharmacokinetics optimization in drug research: biological, physicochemical and computational strategies (Testa, Van de Waterbeemed H, Folker G, Guy R, eds.), pp 217–243. New York: Wiley-VCH, 2001. 64. Graaf deC, Vermeulen EPN, Feenstra NK. Cytochrome P450 in silico: an integrative modeling approach. J Med Chem 48: 2726–2755, 2005. 65. Ertel SI, Ertel EA, Clozel JP. T-type Ca2+ channels and pharmacological blockade: potential pathophysiological relevance. Cardioavasc Drugs Ther 11: 723–739, 1997. 66. Van de waterbeemed H. The fundamental variables of the biopharmaceutics classification system (BCS): a commentary. Eur J Pharm Sci 7: 1–3, 1998. [PubMed]67. Liljebris C J, Larson SD, Ogg D, Palazuk JB, Bleasdale E. Investigation of potential bioisosteric replacements for the carboxyl groups of peptidomimetic inhibitors of protein tyrosine phosphatase 1B: identification of a tetrazole-containing inhibitor with cellular activity. J Med Chem 45: 1785–1798, 2002. [PubMed]68. Feng RM, Assessment of blood-brain barrier penetration: in silico, in vitro and in vivo. Curr Drug Metab 3: 647–657, 2002. [PubMed]69. Raub JT. Early preclinical evaluation in support of hit identification and lead optimization for brain exposure. AAPS Workshop on Optimization of Drug-Like Properties During Lead Optimization. Parsippany, NJ, 19–22 September, 2004. 70. Recanatini M, Poluzzi E, Masetti M, Cavalli A, De Ponti F. QT prolongation through hERG K+ channel blockade: current knowledge and strategies for the early prediction during drug development. Med Res Rev 25: 133–166, 2005. [PubMed]71. Redfern SW, Carlsson L, Davis SA, Lynch GW, MacKenzie I, Palethorpe S, Siegl S KP, Strang I, Sullivan TA, Wallis R. Relationships between preclinical cardiac electrophysiology, clinical QT interval prolongation and torsade de pointes for a broad range of drugs: evidence for a provisional safety margin in drug development. Cardiovasc Res 58: 32–45, 2003. [PubMed]72. Adenot M, Lahana R. Blood-brain barrier permeation models: discriminating between potential CNS and non-CNS drugs including P-glycoprotein substrates. J Chem Inf Comp Sci 44: 239–248, 2004. 73. Sadowski J, Kubinyi H. A scoring scheme for discriminating between drugs and nondrugs. J Med Chem 41: 3325–3329, 1998. [PubMed]74. Ajay, Walters WP, Murcko MA. Can we learn to distinguish between “drug-like” and “nondrug-like” molecules? J Med Chem 41: 3314–3324, 1998. [PubMed]75. Ajay, Walters WP, Murcko MA. Designing libraries with CNS activity. J Med Chem 41: 4942–4951, 1998. 76. Lipinski CA, Lombardo F, Dominy BW, Feeney PJ. Experimental and computational approaches to estimate solubility and permeability in drug discovery and development settings. Adv Drug Deliv Rev 23: 3–25, 1997. 77. Wenlock MC, Austin RP, Barton P, Davis AM, Leeson PD. A comparison of physiochemical property profiles of development and marketed oral drugs. J Med Chem 46: 1250–1256, 2003. [PubMed]78. Lipinski CA. Drug-like properties and the causes of poor solubility and poor permeability. J Pharmacol Toxicol Methods 44: 235–249, 2000. [PubMed]79. Vieth M, Siegel MG, Higgs RE, Watson IA, Robertson DH, Savin KA, Durst GL, Hipskind PA. Characteristic physical properties and structural fragments of marketed oral drugs. J Med Chem 47: 224–232, 2004. [PubMed]80. Lipinski CA. Drew University Medical Chemistry Special Topics Course. July 1999. 81. Lipinski CA. Lead- and drug-like compounds: the rule-of-five revolution. Drug Discov Today Technol 1: 337–341, 2004. 82. Mahar Doan KM, Humphreys JE, Webster LO, Wring SA, Shampine LJ, Serabit-Singh CJ, Adkinson KK, Polli JW. Passive permeability and P-glycoprotein-mediated efflux differentiate central nervous system (CNS) and non-CNS marketed drugs. J Pharmacol Exp Ther 303: 1029–1037, 2002. [PubMed] |
| |