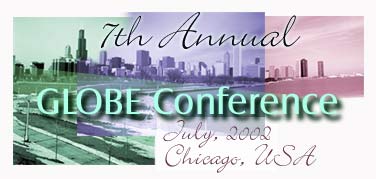
Embassy Suites - Downtown/Lakefront
Chicago, IL, USA
July 22-26, 2002
Science Report:
MUC-A-THONS and Land Cover Mapping: The Saga Continues
Russell G. Congalton* and Mimi Becker
*Department of Natural Resources
215 James Hall
University of New Hampshire
Durham, NH 03824
Email: russ.congalton@unh.edu
Homepage: http://www.globe.unh.edu/
ABSTRACT
The GLOBE Land Cover/Biology Investigation Team at the University of New Hampshire has been cooperating with teachers and students around the world to encourage and foster the collection of ground-based reference data through an intensive activity called a MUC-A-THON. In addition, the team has aided many schools in producing land cover maps from remotely sensed data of their area either by manual interpretation or automated image processing of their satellite imagery. This paper reviews the justification and methods needed to conduct a MUC-A-THON. It also documents some of the ongoing research that we are conducting as a result of GLOBE student-collected land cover data.
INTRODUCTION
Globally, there is a lack of accurate land cover maps available for use in environmental management that includes monitoring deforestation, biodiversity, water quality, ecosystem health, and urban sprawl. Important decisions are based on these maps, however, if accurate maps do not exist, many of these issues cannot be addressed adequately. Land cover mapping is an expensive and time-consuming process that does not always produce acceptable results. Many maps are produced without using reference data to validate the accuracy of the map and are assumed to be sufficient. It has been estimated that only 33% of the earth’s surface has been accurately mapped (Estes and Mooneyhan, 1994).
Students participating in the Global Learning and Observations to Benefit the Environment (GLOBE) Program have the opportunity to provide large quantities of accurate reference data necessary to validate and assess the accuracy of land cover maps representing many areas of the world. The GLOBE Program was established in 1994, through the Office of the Vice President, to coordinate the efforts of several federal agencies including: the National Aeronautics and Space Administration (NASA), National Oceanic and Atmospheric Administration (NOAA), Department of Education (DOE), the Environmental Protection Agency (EPA), the Department of State (DOS) and the National Science Foundation (NSF).
In the GLOBE Program, land cover is classified using The Modified UNESCO Classification (MUC) system, which was developed by the University of New Hampshire Land Cover/Biology Team using an existing international classification scheme created by the United Nations Educational, Scientific, and Cultural Organization (UNESCO, 1973). The new system incorporated new land cover types absent from many other classification systems. MUC contains all the characteristics of a good classification system, and allows for every possible land cover type on Earth to be put into a unique land cover class. Each MUC class is a distinct type of land cover, with a name and identification number, or MUC class "code". By using MUC, all the GLOBE data may be compiled into a single regional or global land cover data set. Therefore, ground collected data may be gathered and used to validate remotely sensed data following the same scientific protocols worldwide. There are ten Level 1 classes (Table 1). Classes 0-7 are natural land cover and classes 8-9 are developed. These ten classes can be further broken down into four levels of classification, with each level increasing in detail (GLOBE, 1997).
Level 1 |
Category |
0 |
Closed Forest |
1 |
Woodland |
2 |
Shrubland |
3 |
Dwarf-shrubland |
4 |
Herbaceous Vegetation |
5 |
Barren Land |
6 |
Wetland |
7 |
Open Water |
8 |
Cultivated Land |
9 |
Urban |
Table 1. First Level MUC classes
THE MUC-A-THON
A MUC-A-THON is a well planned and coordinated event conducted to collect a large number of land cover sample sites in a single day. Often this event occurs on a Saturday and may include one or more participating schools. Since the information being collected is the type of land cover on the ground described by the Modified UNESCO Classification scheme (MUC), we have taken to calling these exciting events MUC-A-THONS. It is typical that tens to hundreds of land cover sample sites be collected in single MUC-A-THON. This event provides an excellent mechanism for a GLOBE school or group of schools to begin to collect enough land cover data to really learn about their area and to analyze the remotely sensed imagery supplied by GLOBE. It is becoming more common for GLOBE schools to conduct annual or semi-annual MUC-A-THONS to get large amount of land cover data. The first MUC-A-THONS were conducted in Dutchess County, NY.
DUTCHESS COUNTY, NY LAND COVER MAPPING
The results presented here represent the scientific analysis of various image processing algorithms used for producing a land cover map of Dutchess County, NY from remotely sensed data. Without the efforts of the teachers and students in the GLOBE Program to collect the land cover data on the ground, this research would not have been possible. Many of the details of this work have been reported previously (Congalton et al. 2001). The final scientific analysis conducted by the Land Cover Team at the University of New Hampshire is presented here.
Dutchess County (Figure 1) occupies 800 square miles in southeastern New York. It is bordered to the west by the Hudson River and to the east by Connecticut. Poughkeepsie is located in the southeastern segment of the county, and is the only large urban area. The landscape is diverse and is dominated by agricultural fields, rolling hills, deciduous forests, and low mountains. The presence of agriculture over much of the landscape has contributed to the patchy distribution of land cover.
Figure 1. Study area
The GLOBE Land Cover Team at the University of New Hampshire (UNH) collaborated with GLOBE schools in Dutchess County, New York. Students and teachers from six schools collected reference data using the GLOBE Protocols and MUC. During this first-ever MUC-A-THON, students collected reference data at many study sites in Dutchess County. This large-scale data collection event was so successful that a second "MUC-A-THON" was held in April of 1999, and a third in May of 2000. This is a major breakthrough for the GLOBE Program since no other schools have collected as much land cover data.
The Land Cover/Biology Team at UNH created land cover maps using different image processing algorithms including: (1) supervised classification, (2) unsupervised classification, and (3) a hybrid approach that combines the benefits of supervised and unsupervised. The obvious question is which of these approaches is best. The only way to determine this answer is to perform an accuracy assessment on each land cover map and generate an error matrix for each. A statistical technique called the Kappa analysis (Congalton and Green 1999) can then be used to determine which land cover map is the most accurate. Without the land cover sample site data (ground samples) collected by the GLOBE schools in their three MUC-A-THONS, it would not have been possible to perform this accuracy assessment.
The error matrices from the accuracy assessment of the three image processing algorithms are presented in Tables 2-4. Table 2 shows the error matrix and summary statistics including overall, producer’s and user’s accuracies for the supervised classification. The overall accuracy here was 77%. Table 3 presents the results from the unsupervised classification with an overall accuracy of 70%. Table 4 documents the hybrid approach results with an overall accuracy of 80%. While it appears from the overall accuracies that the hybrid approach works the best, it is important to be able to statistically test if this is true. The results of the Kappa analysis are presented in Table 5. These results indicate that supervised and hybrid accuracies are not significantly different from each other. Therefore, it can not be stated that the hybrid is any better than the supervised approach. However, the unsupervised and hybrid accuracies are significantly different than each other. Therefore, we can say that the hybrid approach is significantly better than the unsupervised approach. Without conducting an accuracy assessment, none of these conclusions would be known.
AUBURN, ME CHANGE DETECTION ANALYSIS
The UNH GLOBE Land Cover Team has established a great relationship with Ms. Patricia Gaudreau, the director of the Auburn, ME School District’s Environmental Land Lab and Mr. Michael O’Brien, the GIS Coordinator for the city of Auburn, ME. GLOBE schools under Ms. Gaudreau’s direction and with our support have already conducted one MUC-A-THON and have collected over 100 sample sites. They plan on conducting several other MUC-A-THONS to collect more land cover data. The GLOBE students will use these data to make a land cover map from their remotely sensed satellite imagery and design a nature trail. The UNH GLOBE Land Cover Team supports this student effort and is using the student-collected data to aid in the creation and validation of a land cover change detection assessment. Land cover maps will be generated from two dates of imagery (Figures 2 and 3) and a map of land cover change will be created. A UNH graduate student is already at work on this analysis and plans to incorporate future GLOBE student-collected data into this project. Given the proximity of Auburn to UNH, this is a great site for us to continue to test and develop our land cover mapping and accuracy assessment methodologies.
NEW ZEALAND ENVIRONMENTAL MONITORING
We have recently established an agreement with a number of groups in New Zealand to aid in land cover mapping for ecological restoration and biodiversity analysis. The groups involved include: the UNH GLOBE Land Cover Team (Dr. Russell G. Congalton and Dr. Mimi L. Becker), GLOBE New Zealand (Mr. John Lockley – GLOBE Country Coordinator), Landcare Crown Research Institute – University of Waikato (Dr. Margaret Lawton), and the EcoQuest New Zealand Education Foundation (Mr. Jono Clark). Historically, New Zealand has converted large portions of its forestland to non-native monocultures. There is current interest in monitoring these changes and also restoring areas back to the native vegetation. This project is of interest to the entire country and is a unique opportunity to make use of GLOBE student-collected data. One of the most important areas of research in New Zealand currently is the Land Environments of New Zealand (LENZ) project. This project, funded by the Ministry of the Environment, is mapping all the terrestrial ecosystems in New Zealand. It is anticipated that the GLOBE student-collected data will provide replicated, fine-scale studies across all of New Zealand to complement this national land cover database. Initial work will be done in a small pilot area selected by consensus of the four team members. Mokia Island and Tiri Tiri Matangi have been suggested. Eventually, larger areas such as the Motueka River Catchment will be analyzed. Baseline land cover maps will be created by the UNH Land Cover team using ground data collected by GLOBE students for training area delineation. These base maps will be created in conjunction with ongoing, country-wide efforts throughout New Zealand by the Landcare Institute. Then a second date of imagery will be selected to analyze change. These maps will be used to monitor restoration efforts and to analyze biodiversity in the restored areas. We are extremely excited to bring together these groups for such an important project.
CONCLUSION
Land cover maps validated using student reference data can be important contributions to the remote sensing community. Moreover, with the help of students around the world, a larger area of the earth’s surface could be accurately mapped. This concerted effort of intensive global data collection has never been attempted before, and could be the catalyst for future mapping projects worldwide.
Congalton, R., R. Rowe, and M. Becker. 2001. Using GLOBE student-collected muc-a-thon data to aid in assessing the accuracy of a Landsat Thematic Mapper-derived land cover map of Dutchess County, New York. Proceedings of the Sixth Annual GLOBE Conference, Blaine, Washington. pp. 260-269.
Congalton, R.G., and K. Green, 1999. Assessing the Accuracy of Remotely Sensed Data: Principles and Practices. Lewis Publishers, Boca Raton, FL. 160 pp.
Estes, J.E, and W. Mooneyhan, 1994. Of Maps and Myths. Photogrammetric Engineering and Remote Sensing, Vol. 60, No. 5, pp. 517-524.
GLOBE Program Teacher’s Guide, 1997. Phase II, 1997 Edition. GLOBE Office, Washington, D.C.
United Nations Educational, Scientific and Cultural Organization (UNESCO). International classification and mapping of vegetation. 1973. Switzerland: 37 pp.
Table 2. Error matrix for the supervised classification of Dutchess County, NY.
Table 3. Error matrix for the unsupervised classification of Dutchess County, NY.
Table 4. Error matrix for the hybrid classification of Dutchess County, NY.
* indicates statistical significance at 95% confidence level.
Table 5. Kappa results for the three different classification approaches used to create the land cover map of Dutchess County, NY.
Figure 2. False color composite Landsat Thematic Mapper (TM) image of Auburn, ME and surroundings from September 16, 1993.
Figure 3. False color composite Landsat Thematic Mapper (TM) image of Auburn, ME and surroundings from August 26, 2000.