1997 Science Program Review
Review Home | Agenda | Review Doc | Posters | Collaborations | Service | Publications | Staff | Logistics
CHAPTER 2: Research on Intraseasonal to Interannual Variability
Much of CDC research is focused on understanding and predicting climate variability on longer than synoptic time scales. We are interested not only in the coherent phenomena that occur on these time scales such as floods, droughts, atmospheric blocking, the tropical MJO, and of course ENSO, but also in the variations of the statistics of weather systems. Some of these phenomena are of shorter duration than what one might typically associate with `climate' variability. At CDC, however, we recognize that a description of climate variability in terms of seasonal mean anomalies can often give a bland and even misleading view of what most people experience as unusual weather. Hence our broader focus.We use a combination of empirical and modeling approaches to these problems. The empirical studies range from simple descriptions employing a variety of observational datasets to `linear inverse modeling' studies. The latter are designed to throw light on the underlying dynamics, and also yield statistical forecast models that can be competitive with general circulation models. Our modeling studies are conducted using models ranging in complexity from simple planetary Rossby wave propagation models to the global NCEP atmospheric GCM.
In the last four years, CDC scientists have made important contributions to the understanding and prediction of seasonal tropical SST anomalies and their impact on the extratropical atmosphere. They have also made significant contributions to the fundamental dynamics of extratropical low-frequency variability, especially the dynamics governing the statistics of weather systems, and have pursued novel approaches to the problem of anticipating unusual weather. Some of this research is summarized below. More details can be found in the peer-reviewed journal articles at the end of the chapter.
2.1 Understanding and predicting seasonal tropical SST variations
2.1.1 SST variations in the tropical Indo-Pacific ocean
For several years, CDC scientists have been supplying skillful forecasts of Indo-Pacific SST anomalies, which are an important part of the ENSO phenomenon. These forecasts are published in two publications of the National Weather Service: the quarterly Experimental Long-Lead Forecast Bulletin, and the monthly Climate Diagnostics Bulletin, and are also available on the World Wide Web.The forecasts are made using Linear Inverse Modeling, which is a method of extracting the dynamical parameters of a system from data. The assumption is made that the dynamics can be modeled as a stable linear multivariate process driven by geographically coherent white noise. Physically, the white noise represents the broad-band nonlinear dynamics affecting the more slowly varying `deterministic' (and predictable) part of a measure d signal. This assumption has been found to be remarkably appropriate for the description of ENSO as manifested in Indo-Pacific SST anomalies. The assumption passes a variety of tests, including the difficult `Tau-test', which suggests not only that the deterministic dynamics can be treated as linear and stable, but also that the parameters associated with these deterministic linear dynamics can be treated as seasonally independent.
The inverse modeling yields a system propagator (or Green function) which is used in making forecasts. The eigenfunctions of this propagator matrix represent the natural modes of variability of the system. Now if a dynamical system is stable, its eigenmodes must all individually decay. How then can the observed predictable growth of SST anomalies occur? The answer is that since the modes are not orthogonal, temporary SST anomaly growth can still occur from their constructive interference. It can be shown that the domain-averaged square of Indo-Pacific SST anomalies can amplify by as much as a factor of 5 over 7 months by this mechanism. The initial SST anomaly pattern associated with this optimal growth is shown in Fig. 2.1a. The model predicts that if such a pattern were actually to occur as an initial condition, it would evolve 7 months later into the amplified mature ENSO pattern in Fig. 2.1b. That this is more than an interesting theoretical possibility is demonstrated in Fig. 2.2. The plot shows that whenever the evolving observed SST anomaly field projects strongly on the optimal pattern in Fig. 2.1a, it also tends to project strongly on the pattern in Fig. 2.1b seven months later. In other words, it leads to ENSO.
a) ![]() |
![]() |
Figure 2.1 | Figure 2.2 |
An example of a forecast made with our multivariate first-order linear inverse model (actually a hindcast, but of independent data) is given in Fig. 2.3. The figure shows the observed 3-month mean SST anomaly field for January, February and March (JFM) 1972, the 2-season SST anomaly forecast made for JAS 1972 using this as the initial condition, and the observed SST anomaly field for JAS 1972. The observed field undergoes a substantial change in pattern, magnitude, and sign over the two seasons, yet the model correctly predicts most elements of this change. This is an example of a good, but not the best, forecast that can be made using our model. It was chosen to illustrate the points made above, and also highlight the multivariate nature of the coupled dynamics that are clearly reasonably well represented in the model. A univariate Markov model would be unable to predict either the growth or the changes of sign in Fig. 2.3. It would merely have the initial condition decay to climatology, possibly with different decay rates at different points, over the domain.
![]() |
![]() |
![]() |
Figure 2.3 |
Finally, our assumption of statistical stationarity might appear inconsistent with the clear observed preference of El Niño to occur during certain times of year. In fact there is no inconsistency. In our description, the observed phase locking to the annual cycle is transmitted to the SST anomalies through a seasonally dependent variance of the stochastic forcing. Indeed the stochastic forcing diagnosed from SST anomaly data using a fluctuation-dissipation relation appropriate only to a stable linear process yields a seasonally dependent forcing variance. When our stable linear inverse model is driven with this nonstationary stochastic forcing, the correct phase locking of El Niño to the annual cycle is obtained. We emphasize again that the forcing variance is the only seasonally varying specified quantity in the model, and that it is neither the deterministic dynamics alone nor the stochastic forcing alone but the interplay of the two which accounts for the observed growth of El Niño events and their phase locking to the annual cycle.
2.1.2 SST variations in the tropical Atlantic ocean
The inverse modeling approach has also been applied to the problem of understanding and predicting SST variations in the tropical Atlantic ocean, described in many studies as being dominated by a north-south SST dipole pattern. Two different inverse models, based on SST data in the tropical Atlantic alone and in the tropics as a whole, were constructed. It was found that the global tropical model is more skillful than the Atlantic model in predicting SST anomalies in the Caribbean and north tropical Atlantic ocean, but not in the equatorial and south tropical Atlantic oceans, where it is difficult to beat persistence.To help resolve the debate as to whether the dipole found in various EOF analyses is an artifact of the technique or a physically real structure, 6-month `influence functions' (not shown) were determined for area-averaged SST anomalies in the north and south tropical Atlantic oceans. The 6-month influence function for the north (south) Atlantic SST represents the optimal initial SST pattern for maximizing SST over the north (south) Atlantic 6 months later. In other words, it shows at each geographical location the sensitivity of the area-averaged north (south) Atlantic SST to the SST at that location 6 months earlier. The maps constructed using only the tropical Atlantic data were found to be similar and of opposite sign, with both leading to a clear dipole in six months. On the other hand, the maps constructed using the global tropical SST data showed a large sensitivity of the north, but not the south, Atlantic SSTs to the SSTs in the Indo-Pacific basin, especially Niño-3. These results suggest that the tropical Atlantic SST dipole is a real phenomenon, but that the influence of the Indo-Pacific disrupts its northern branch so that the dipole is rarely seen in its pure form.
2.2 The impact of tropical SST variations on the low-frequency variability of the extratropical atmosphere
The impact of ENSO is detectable worldwide. The recent progress in predicting tropical Pacific SSTs several seasons ahead therefore raises the hope that at least some aspects of interannual variability may also be predictable elsewhere, perhaps even in the apparently turbulent extratropics. Many GCM groups are assessing the impact of SST variations on the global atmospheric circulation in pursuit of this goal. CDC scientists are also involved in this effort through a collaboration with NCEP's Coupled Model Project. We have access to the output from all the model runs performed under the project. A 12-member ensemble of 45-year runs, made with the (T40,18-level) atmospheric GCM with different initial conditions but the same observed global SST fields for 1950-95 as evolving specified boundary conditions, has proved especially valuable. NCEP has also generously provided us with the GCM itself, so we can perform our own experiments.2.2.1 Signal-to-noise ratio and predictability
How large is the tropical SST-forced signal in the extratropics? The question is surprisingly difficult to answer. One possible answer is given by the 45-year runs mentioned above. The SST-forced signal in these runs is defined as the 12-member ensemble average minus climatology. The top panel of Fig. 2.4 shows the total variance of the 540 (= 45x12) simulated winter mean 200 mb height fields. The SST-forced contribution to this, i.e. the variance of the 45 ensem ble-averaged winters, is shown in the middle panel. The bottom panel shows the ratio of the SST-forced variance to the total variance. Clearly, the SST signal explains only a small part of the total variability of seasonal means in middle latitudes, and its influence is mostly confined to the Pacific-North American (PNA) sector.![]() |
![]() |
![]() |
Figure 2.4 |
![]() |
![]() |
Figure 2.5 |
![]() |
![]() |
![]() |
Figure 2.6 |
2.2.2 The importance of the different `flavors' of El Niño
Small though the SST-forced signal is in middle latitudes, it is at present the only predictable seasonal signal, and as such it is important to get it right. The question arises as to whether it is sensitive to the details of the tropical SST forcing. If it is, the accuracy of tropical SST predictions will need to be high: it will not be sufficient to issue forecasts of area-averaged SST in, say, the eastern equatorial Pacific ocean alone.Fig. 2.7 shows the observed anomalous tropical SST and 500 mb heights in the PNA sector for four El Niño winters. The height fields are appreciably different from one another, and so are the SST fields. To what extent are the differences in t he former attributable to those in the latter? An answer can again be given using the 45-year runs discussed above. Fig. 2.8 shows the SST-forced 500 mb height signals in the four cases. The patterns are similar to one another, despite the tropical SSTs (and the simulated rainfall fields, shown in the tropical sections) being appreciably different. This suggests that the differences in the height fields in Fig. 2.7 are not mainly due to the differences in the SST fields. The 500 mb height signals in Fig. 2.8 are relatively insensitive to the pattern of the SST forcing; they show more sensitivity to its amplitude. However, even such modest differences in the signals for different El Niño events may prove important in regional predictions. As an example, Fig. 2.9 shows the precipitation signals along the west coast of North America for 9 recent El Niño events. The variation between events is not negligible.
![]() Figure 2.7 |
![]() Figure 2.8 |
![]() Figure 2.9 |
2.2.3 Identifying the most sensitive areas of tropical SST forcing

The
Green function thus estimated was then used to construct SST
influence functions (i.e., sensitivity maps) for linear measures of
the extratropical response in certain target regions of interest.
As explained in the previous section, such a map represents the
optimal SST anomaly pattern for maximizing the response in the
target region, and also shows, at each geographical location, the
sensitivity of the response in the target region to an SST anomaly
at that geographical location. Fig.
2.11 shows the SST influence functions for area-averaged
precipitation in five different regions of North America. The
figure dramatically illustrates the very different sensitivities of
the GCM's precipitation in these regions to tropical SST forcing,
and also identifies the most sensitive areas of SST forcing for
generating large precipitation anomalies in the target areas.
2.2.4 Nonlinear aspects of the response
The prevailing view of the global atmospheric climate signal associated with ENSO is that of a linear response, with the spatial pattern of anomalies in the warm (El Niño) phase opposite to that in the cold (La Niña) phase. We find that this paradigm is flawed. The upper and lower panels of Fig. 2.12 show observational composites of the tropical rainfall and 500 mb eddy height anomalies in northern winter for El Niño and La Niña, respectively. The patterns in the lower panel are clearly not opposite to those in the upper panel. The extrema of both the rainfall and 500 mb height anomaly fields in La Niña are shifted well westward of their El Niño counterparts.Given the short observational record from which they are derived, one might question the statistical significance of these results. Also, the rainfall and 500 mb height composites in Fig. 2.12 are not derived from the same set of ENSO events: the rainfall composites are based on 4 warm and 4 cold events since 1974; the height composites on 9 warm and 9 cold events since 1950. Finally and perhaps most importantly, the composites are not for strictly equal and opposite SST anomalies.
![]() |
![]() |
Figure 2.12 |
To settle these doubts, 40 integrations of the NCEP GCM were performed with a warm ENSO-like SST life cycle as boundary forcing. The experiment was then repeated with the sign of the SST forcing reversed. Fig. 2.13 shows the ensemble-average rainfall and 500 mb height anomalies obtained in the two GCM experiments, in an identical format to that of Fig. 2.12. Even though the SST forcing is exactly equal and opposite in the experiments, there is an asymmetry of the response similar to that in Fig. 2.12. The basic reason for this asymmetry, we believe, is that tropical rainfall responds to the total, rather than the anomalous, SST.
![]() |
![]() |
Figure 2.13 |
Given this evidence that the nonlinearity seen in Fig. 2.12 is real, and predictable to some extent, one would hope that the nonlinear NCEP GCM would have a substantial advantage over linear models in forecasting ENSO-related seasonal anomalies over the PNA region. In fact the advantage is only slight. The skill of the simplest possible linear forecast -- that the seasonal anomaly pattern is the observational composite of Fig. 2.6a during warm, and the opposite pattern during cold, ENSO winters -- when plotted in Fig. 2.5 (not shown), turns out to be very similar to that of the NCEP GCM.
2.3 The basic dynamics of extratropical low-frequency variability
Much of extratropical low-frequency variability is apparently unpredictable noise, unrelated to tropical SST variations. Although its statistical structure is different from that of synoptic variability, with variance maxima over the eastern rather than the western Pacific and Atlantic oceans, it is strongly affected by the behavior of synoptic weather systems as they approach and decay in these regions of diffluent flow. Much of its unpredictability therefore ultimately arises from the unpredictability of synoptic weather systems. The low-frequency variability is also affected by the behavior of tropically forced Rossby waves, such as those associated with the MJO, in these diffluence regions. A clear understanding of eddy-mean flow interactions in these regions (as well as of the eddies themselves) would be welcome, but has remained elusive for decades. Progress in this area will have large implications for the predictability of intraseasonal, interannual and even interdecadal variability. Recent work by CDC scientists has helped clarify several aspects of the problem, which we summarize next.2.3.1 Low-frequency Rossby wave dynamics
Extratropical low-frequency anomalies tend to be `equivalent barotropic', in the sense that they extend through the troposphere with relatively little vertical tilt. If their structures were precisely separable in the horizontal and vertical coordinates, their dynamics would be governed by the barotropic vorticity equation at an equivalent barotropic level somewhere in the upper troposphere. Many investigators have studied low-frequency variability in terms of upper tropospheric Rossby wave dynamics, and a great deal has been learned about atmospheric teleconnections in this way. In a seminal 1983 paper, Simmons, Wallace and Branstator (SWB) found that low-frequency planetary scale Rossby waves evolving on the zonally varying climatological upper tropospheric flow were weakly unstable, and had structures strongly reminiscent of those of the observed variability. Because of their slow growth, SWB doubted that such waves would appear in a pure form on synoptic charts, and argued instead that they would be more evident in long-term statistics, especially in a scenario in which the ambient flow is constantly perturbed by random forcing. Fig. 2.14 shows the streamfunction of the most
unstable Rossby wave in its two quadrature phases, obtained from
our own eigenanalysis of the observed wintertime upper tropospheric
flow. Except near the pole, the resemblance to observed
low-frequency structures is undeniable. The amplitude pattern of
the wave (not shown, but imagine the sum of the squares of the two
panels) is similar to the observed variance of low-pass filtered
300 mb streamfunction anomalies, given in the upper left panel of
Fig. 2.15. The resemblance, though far
from perfect, is impressive considering the vast simplification
made to the dynamics. One is tempted to think: here is the essence
of low-frequency variability; a few such Rossby waves, continually
excited by white noise forcing, with a little extra push from
anomalous tropical SSTs during ENSO events. Unfortunately, a closer
look (published in three papers in the Journal of the
Atmospheric Sciences) reveals that this engagingly simple view
is not valid in any quantitatively useful sense. The basic
difficulty is that the forcing cannot be treated as random with a
simple statistical structure. If it were white, the linear
barotropic model, given an initial streamfunction anomaly
x(0), would predict a most probable anomaly at a later time as
x(t) = G(t) x(0), where G is the barotropic
propagator. The lower right panel of Fig.
2.15 shows the poor average skill of 640 10-day forecasts of
low-pass 300 mb streamfunction made in this manner, using observed
initial conditions in the winters of 1985-93. Given that one does
not expect such a simple model to produce accurate forecasts, only
reasonably accurate statistics, one might consider this test too
harsh. Unfortunately, the model does not fare any better at
reproducing the observed statistics. For a white noise forcing, it
predicts that the lag-covariances C(t) are linked to the
zero-lag covariance C(0) as C(t) = G(t) C(0).
The remaining two panels of Fig. 2.15
compare the observed 10-day lag-covariance C(10) of the
low-pass 300 mb streamfunction with that predicted using the
observed C(0) as the zero-lag covariance. The comparison is
poor; there is even an error of sign over large areas.


Figure 2.15
A red noise forcing produces somewhat better, but still unrealistic, lag-covariances (not shown). We have systematically investigated the statistics generated by a hierarchy of stochastic forcing distributions, and concluded that extratropical low-frequency variability cannot be viewed as stochastically forced barotropic Rossby waves evolving on the climatological zonally varying upper tropospheric flow. The spatial and temporal structure of the observed variability cannot be understood without also taking into account the detailed spatial and temporal structure of the forcing, respectively.
2.3.2 The most sensitive areas of Rossby wave forcing, and their dependence on season

In addition to highlighting the sensitivity of western U.S. height anomalies to different forcing areas at different times of year, Fig. 2.16 makes another important point. For an arbitrary forcing F, the response R in the target region is the projection of F on the sensitivity maps S in Fig. 2.16; we write R = S * F. The fact that S is strongly time-dependent then raises the possibility of interactions across time scales. In other words, it is possible for a steady forcing F to produce an unsteady response, and equally, for an unsteady forcing to produce a seasonal-mean response. Fig. 2.16 suggests that such multiscale interactions would be relatively strong in the transition seasons. Chapter 3 discusses a study of the 1988 U.S. drought in which they may have played a significant role.
2.3.3 Understanding and predicting the statistics of synoptic weather systems
Extratropical synoptic variability, with variance maxima over the western Pacific and Atlantic oceans (often referred to as storm-tracks), is strongly affected by the slow variations of the background flow in space and time. The variations of the storm tracks, in turn, affect the variations of the background flow, again in both space and time. As mentioned earlier, a proper understanding and modeling of this interaction remains an outstanding problem in meteorology. Some progress has been made by uncoupling the two components of the problem, and asking questions such as 1) given a background flow, to what extent can one predict the storm tracks? and 2) given the storm tracks, to what extent can one predict the structure of the background flow?Research conducted at CDC has shed new light on the first of these questions. Two different, but complementary, studies have been performed. In the first, the focus was on clarifying the roles of two apparently competing processes in storm-track organization by a zonally varying background flow: low-level baroclinicity, and upper-level horizontal flow deformation. We addressed this issue in the simplest model capable of generating storm-tracks, a 2-level quasi-geostrophic model with periodic boundary conditions on a midlatitude beta plane. Idealized background flows, with various combinations of baroclinicity and deformation, were prescribed as superpositions of an equivalent barotropic stationary wave on a zonally symmetric baroclinic flow. In such flows the relative strengths of the zonally varying baroclinicity and horizontal deformation are controlled by a single parameter r, the ratio of the stationary wave amplitudes at the upper and lower levels. For r -> 1, the baroclinicity is zonally uniform. In this case the model generates a storm track (Fig. 2.17a) that is located in the entrance region of the upper level jet, just downstream of the point of minimum deformation. For r -> 0, both the baroclinicity and deformation fields vary zonally. The model's storm track (Fig. 2.17c) is now located in the exit region of the upper jet, just downstream of the point of maximum baroclinicity. For intermediate values of r, the model produces two distinct storm tracks in the jet entrance and exit regions (Fig. 2.17b). Thus both baroclinicity and horizontal flow deformation are important, but with very different effects on storm track organization.
![]() |
![]() |
![]() |
Figure 2.17 |
Our goal is to understand storm track organization well enough to be able to predict the different storm tracks associ ated with different background flows, in both observations and GCM simulations. A reasonably simple and accurate `storm-track model' would be useful for understanding seasonal anomalies, diagnosing GCM errors, and parameterizing synoptic eddy fluxes in simple models of low-frequency variability. The midlatitude beta plane model with periodic boundary conditions, though useful for investigating storm track sensitivities to certain features of the background flow, is too simple for this more ambitious purpose.
We have subsequently constructed a more sophisticated, but still very simple, storm-track model that is applicable in realistic settings. We consider a 2-level hemispheric quasi-geostrophic model linearized about a specified background flow, and force it with Gaussian white noise. For seasonal-mean flows, the model is baroclinically stable for a reasonable choice of damping parameters. As emphasized by several investigators, synoptic eddies can still grow on an exponentially stable flow for a finite time, either through local baroclinic and barotropic energy interactions with the flow or in response to the stochastic forcing. In a state of statistical equilibrium, a fluctuation-dissipation relation (FDR; also referred to as the Liapunov equation) links the covariance of the eddies to the structure of the background flow and the covariance of the forcing. We assume that the white noise forcing always has the same constant covariance. Under this assumption, the FDR reduces to a one-to-one link between the synoptic-eddy covariance and the background flow, and so given any background flow, it can be solved to obtain the eddy covariance associated with that flow. All other second-order statistics such as eddy kinetic energy, momentum and heat fluxes, and power spectra, can then also be predicted. This is our storm track model.


Figure 2.18
For long-term mean flows such as the wintertime climatology, the model produces rather realistic climatological storm tracks. Fig. 2.18 shows the observed and predicted synoptic-eddy streamfunction variances at 400 mb, and also the 400 mb streamfunction tendencies induced by the observed and predicted eddy vorticity fluxes. In view of the simplicity of the 2-level quasi-geostrophic dynamics and the drastic assumptions made about the forcing, the model's predictions based only on a knowledge of the climatological mean flow are astonishing. We also find that the FDR is sensitive enough to background flow changes that it is able to predict important aspects of the observed seasonal variation of storm tracks associated with the seasonal variation of the background flow. Fig. 2.19 compares the observed and predicted 400 mb synoptic eddy kinetic energy maps for January and April. The observed Pacific storm track is weaker than the Atlantic storm track in January (even though the Pacific jet is stronger than the Atlantic jet), and stronger than it in April. The model successfully captures this behavior.

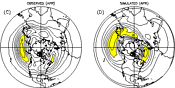
Figure 2.19
The success of these calculations suggests that it is not necessary to invoke either exponential baroclinic instability or the details of synoptic eddy excitation to understand most of the observed characteristics of extratropical storm tracks, and their slow variations in time. Rather, the dynamics of nonmodal synoptic eddy growth in the Pacific and Atlantic jets, and the propagation and dispersion of the eddy activity in the diffluent regions downstream of the jets, appear sufficient for this purpose.
2.3.4 Zonal mean flow - stationary wave interactions

What is the dynamical
significance of the variability of the zonal mean flow? Given that
it affects and is affected by the variability of the stationary
waves, one is again looking at a coupled eddy-mean flow interaction
problem. As before, some insight may be gained by uncoupling it and
asking questions such as: given the anomalous zonal mean flow for a
winter, to what extent can one predict the anomalous stationary
waves for that winter? We have attempted to answer this question
for the winters of 1977-94 using a steady linear baroclinic
stationary wave model. For each winter, we specify the terms
representing the advection of the climatological stationary waves
by the anomalous zonal mean circulation as a steady forcing, and
interpret the response to this forcing as a zonal
anomaly-forced signal. Fig. 2.21
compares the standard deviation of the 17 eddy 500 mb height
signals thus obtained with the standard deviation of the 17
observed eddy 500 mb height anomaly fields. The point of this
comparison is similar to that of the upper and middle panels of Fig. 2.4. The zonal anomaly-forced signals
in Fig. 2.21 apparently "explain" a
much larger fraction of the total seasonal variability than
explained by the SST-forced signals in Fig.
2.4.
These calculations suggest that a substantial portion of the low-frequency variability of the zonally asymmetric circulation is associated with that of the zonal mean circulation. We recognize, of course, that both are mostly unpredictable noise. Nevertheless, the fact that the zonally symmetric variability is relatively low-dimensional noise (in its EOF space) suggests that the zonally asymmetric variability is also relatively low-dimensional noise. It is interesting to note in this context that nearly 64% of the variance of the 17 signals in Fig. 2.21 is explained by a single pattern associated with the anomalous zonal wind profile in Fig. 2.20.
2.3.5 The role of extratropical SST variations

Despite many studies, the impact of such extratropical SST variations on low-frequency atmospheric variability remains unclear. Empirical lead-lag relationships studied by CDC scientists strengthen the view that the SST variability is more of a response to the atmospheric variability than a generator of it. In other words, the extratropical atmosphere cares less about anomalous extratropical SSTs than vice versa. This still leaves the question open, however, as to precisely how much it does care.
Many atmospheric GCM experiments have been performed with prescribed extratropical SST anomalies to determine the atmospheric response to them. The results have been variable and confusing, not only because the response is weak and difficult to establish in short integrations, but also because it is sensitive to the precise location of the prescribed SST anomalies in relation to the atmospheric jet streams and their associated storm tracks.
CDC
scientists are taking some of the first steps in systematically
investigating these sensitivities. A series of long NCEP GCM
integrations have been performed using an idealized SST anomaly
pattern in the northwest Pacific (Fig.
2.23), with amplitude roughly double the standard deviation of
observed SST anomalies in the region. Four sets of 96-month runs
have been made for both perpetual January and perpetual February
conditions. The lower two panels of Fig.
2.23 show the ensemble-average 500 mb height responses obtained
in the two cases. Both are much weaker than the response to
tropical SST anomalies discussed in the previous section, and very
different from one another. Their associated vertical structures
(not shown) are also very different: the January response is
baroclinic; the February response is nearly equivalent barotropic.
These differences highlight the large sensitivity of the response
to the relatively minor changes that occur in the Pacific jet and
storm track from January to February.
2.4 The predictability of extreme climate and weather events
In essence, weather and climate prediction are both problems of predicting the conditional probability distribution Pf of anomalies given that some event (a particular initial condition, ENSO, or a doubling of Carbon Dioxide, for example) has occurred. The usefulness of the prediction depends upon how different Pf is from the unconditional distribution P. If the distributions are normal, they are completely defined by their means and standard deviations; we may write Pf= N (s,





The usefulness of a forecast is then determined by the values of
f/
and s/
. If
f/
<< 1, as in short range weather
prediction, the maximum likelihood forecast s is
deterministic. Such a forecast is useful regardless of whether
s/
is large or small. In
extended-range weather prediction, seasonal forecasting, and global
change scenarios, however,
f/
~ 1. In such a
situation, s can still be useful as a forecast if s/
>> 1. In most cases, however,
s/
<< 1, and consistent with
this the average skill of s is low (e.g., Fig 2.5).
The problem, then, is how to make optimal use of forecasts when
s/ << 1 and
f/
] ~ 1, that is, when Pf
is close to P. There are two possibilities. One is to use
the fact that even in such situations the differences between
Pf and P are relatively large on the tails of
the distributions, and so focus on predicting the altered
probabilities of extreme events. The other is to use the fact that
even if the average forecast skill of s is low, the forecast
is more skillful in some cases than others, either because
s/
] is relatively large or
f/
is relatively small. One may attempt to
identify such cases beforehand, that is, "forecast the forecast
skill." CDC scientists have been investigating both of these
possibilities, the first in the context of seasonal predictions
over North America, and the second in the context of medium to
extended range weather prediction.
2.4.1 The altered risks of extreme events
Even apparently small changes of the normal distribution can have large implications for the probabilities of extreme events. For example, a shift of half a standard deviation to the right (s/






It is clearly important to determine Pf and P as accurately as possible if one is to draw inferences about the altered risks of extreme events associated with ENSO. One may attempt to do this either through a large ensemble of GCM simulations with and without ENSO, or through an analysis of past observed behavior. The two approaches are complementary. Both have their advantages and drawbacks, and both are on our research agenda.
We have made some progress with the
empirical approach in estimating the altered risks of extreme U.S.
precipitation anomalies associated with El Niño and La
Niña. For this study a long-term SOI time series and NCDC
Climate Division precipitation data for 1896-1995 were used. The
results suggest that the odds of extremely dry or wet spring
seasons in the southwestern U.S. are significantly changed as much
as three seasons in advance by the occurrence of ENSO. The altered
odds are most evident at a one-season lead. Fig. 2.24 shows the relative risk, compared to
a climatological risk of 20%, of the occurrence of extreme
springtime precipitation anomalies associated with El Niño
(upper panel) and La Niña (lower panel) in the preceding
winter. There is a great deal of information of both scientific and
practical interest on these maps. The green areas marked `Wet' are
those for which there is a substantially higher risk of an
extremely wet spring accompanied by a substantially lower risk of
an extremely dry spring. The yellow areas marked `Dry' are those
for which the opposite is true, that is, a substantially higher
risk of an extremely dry spring accompanied by a substantially
lower risk of an extremely wet spring. In the olive green areas
marked `Both', there is a higher risk of both extremely dry and
extremely wet springs due to increased variability associated with
ENSO (
f/
> 1), and in the red areas marked
`Reduced', there is a reduced risk of both extremely dry and
extremely wet springs due to reduced variability (
f/
< 1). Note also the interesting
asymmetries in the maps for El Niño and La Niña.
2.4.2 Forecasting the forecast skill of weather events
No weather or seasonal forecasting system displays uniform forecast skill. In the s/






Fig. 2.25 shows
some results from a recently completed study of the error/spread
relationship in 105 cases of 17-member ensemble forecasts made at
NCEP in the winter of 1995/96. In each case, the error E is
defined at each gridpoint as the magnitude of the ensemble-mean
forecast error, and the spread
f as the mean deviation
of the individual members from the ensemble-mean. The two
quantities are then averaged over all 105 cases and all grid
points. The blue and black curves in Fig.
2.25 show such averages of E and
f, respectively, for the 250 mb
streamfunction as a function of forecast lead time. Ideally, the
two curves would be identical. The fact that they are not suggests
that there is room for improvement in the NCEP ense mble
forecasting system; it is possible that both initial condition and
model errors are being undersampled in generating the
ensembles.
The red curve in Fig. 2.25 shows the
correlation of the variations of E and f over the 105 cases,
obtained first at each grid point and then averaged over all grid
points. It reaches a maximum of about 0.3 at forecast day 5, and
decreases rapidly after that. One expects the correlation to decay
for longer lead times, because at longer lead times the conditional
probability distribution N(s,
f) tends to the
climatological distribution N(0,
). The ensemble mean forecast is then
always close to climatology, and therefore its error varies from
case to case with standard deviation
. The ensemble spread, however, remains
approximately constant near
, and so the
correlation drops.
The error/spread relationship between the ensemble-mean error
E and the ensemble spread f is thus fairly weak in
the NCEP forecast ensembles. As we saw in regard to the
extratropical impact of ENSO in the previous sections, however,
weak linear correlations can give a misleading impression of the
utility of the connection between two quantities. Table 2.1 is a contingency table of ensemble-mean
error and ensemble spread for the same 105 forecasts of 250 mb
streamfunction. The entries are proportional to the joint
probability of obtaining the error and spread values in the
indicated quintiles. Thus an entry in the ith row
and jth column represents the probability of the
simultaneous occurrence of E in its ith quintile and
f in its jth quintile. If there
were no relationship between E and
f, all the entries would be 0.2. If
there were a perfect linear relationship, all the diagonal elements
would be 1 and all the off-diagonal elements would be zero. The
actual values are only marginally different from 0.2 over most of
the table, but they are substantially different from 0.2 at its
corners. This means, for example, that when
]f is in its highest quintile, the chances of
E being in its highest quintile are 3.4 times the chances of
its being in its lowest quintile. There is thus some utility in the
relationship between spread and error, which is obscured by the low
linear correlation.
Spread quintile | ||||||
---|---|---|---|---|---|---|
0.1 | 0.3 | 0.5 | 0.7 | 0.9 | ||
Error quintile | 0.1 | 0.34 | 0.22 | 0.19 | 0.14 | 0.10 |
0.3 | 0.23 | 0.22 | 0.20 | 0.20 | 0.14 | |
0.5 | 0.18 | 0.22 | 0.21 | 0.21 | 0.18 | |
0.7 | 0.16 | 0.19 | 0.20 | 0.21 | 0.23 | |
0.9 | 0.09 | 0.15 | 0.18 | 0.24 | 0.34 |
Text contributed by: P. Sardeshmukh, J. Barsugli, M. Borges, R. Dole, M. Hoerling, M. Newman, S. Peng, C. Penland, J. Whitaker, and K. Wolter.
References:
-
Journal Articles
- Borges, M. D. and P. D. Sardeshmukh 1995: Barotropic Rossby
wave dynamics of zonally varying upper level flows during northern
winter. J. Atmos. Sci., 52, 3779-3796.
Deser, C. and M. S. Timlin 1997: Atmosphere-Ocean Interaction on weekly time scales in the North Atlantic and Pacific. J. Climate, 10, 393-408.
Hoerling, M. P. and M. Ting 1994: On the organization of extratropical transients during El Niño. J. Climate, 7, 745-766.
Hoerling, M. P., M. Ting and A. Kumar 1995: Zonal flow-stationary wave relationship during El Niño: Implications for seasonal forecasting. J. Climate, 8, 1838-1852.
Kumar, A. and M. P. Hoerling 1995: Prospects and limitations of seasonal atmospheric GCM predictions. Bull. Amer. Meteor. Soc., 76, 335-345.
Kumar, A., M. Hoerling, M. Ji, A. Leetmaa and P. D. Sardeshmukh 1996: Assessing a GCM's suitability for making seasonal predictions. J. Climate, 9, 115-129.
Kumar, A. and M. P. Hoerling 1997: Interpretation and implications of observed inter-El Niño variability. J. Climate, 10, 83-91.
Newman, M., P. D. Sardeshmukh and C. Penland 1997: Stochastic forcing of the wintertime extratropical flow. J. Atmos. Sci, 54, 435-455.
Penland, C. and M. Ghil 1993: Forecasting Northern Hemisphere 700mb geopotential height anomalies using empirical normal modes. Mon. Wea. Rev., 121, 2355-2371.
Penland, C. and T. Magorian 1993: Prediction of Niño 3 sea-surface temperature anomalies using linear inverse modeling. J. Climate, 6, 1067-1075.
Penland, C. and L. Matrosova 1994: A balance condition for stochastic numerical models with application to the El Niño - Southern Oscillation. J. Climate, 7, 1352-1372.
Penland, C. and P. D. Sardeshmukh 1995: The optimal growth of tropical sea surface temperature anomalies. J. Climate, 8, 1999-2024.
Penland, C. and P. D. Sardeshmukh 1995: Error and sensitivity analysis of geophysical systems. J. Climate, 8, 1988-1998.
Penland, C. 1996: A stochastic model of IndoPacific sea surface temperature anomalies. Physica D, 98, 534-558.
Sardeshmukh, P. D., M. Newman and M. D. Borges 1997: Free Barotropic Rossby wave dynamics of the wintertime low-frequency flow. J. Atmos. Sci., 54, 5-23.
Ting, M. and P. D. Sardeshmukh 1993: Factors determining the extratropical response to equatorial diabatic heating anomalies. J. Atmos. Sci, 50, 907-918.
Ting, M. and M. P. Hoerling 1993: Dynamics of stationary wave anomalies during the 1986/87 El Niño. Climate Dyn., 9, 147-164.
Ting, M., M. P. Hoerling, T.-Y. Xu and A. Kumar 1996: Northern Hemisphere teleconnection patterns during extreme phases of the zonal mean circulation. J. Climate, 9, 2614-2623.
Whitaker, J. S. and R. M. Dole 1995: Organization of storm tracks in zonally varying flows. J. Atmos. Sci., 52, 1178-1191.
-
Submitted or in Press
- Borges, M. D. and P. D. Sardeshmukh 1997: Application of
perturbation theory to the stability analysis of realistic
atmospheric flows. Tellus, in press.
Hall, N. M. J. and P. D. Sardeshmukh 1997: Is the time-mean Northern Hemisphere flow baroclinically unstable? J. Atmos. Sci, accepted.
Hoerling, M. P., A. Kumar and M. Zhong 1997: El Niño, La Niña, and the nonlinearity of their teleconnections. J. Climate, 10, in press.
Hoerling, M. P. and A. Kumar 1997: Origins of extreme climate states during the 1982-83 ENSO winter. J. Climate, 10, in press.
Hoerling, M. P. and A. Kumar 1997: Why do North American climate anomalies differ from one El Niño event to another? Geophys. Res. Lett., in press.
Newman, M. and P. D. Sardeshmukh 1996: The impact of the annual cycle upon the North Pacific/North American response to low frequency forcing. J. Atmos. Sci., submitted.
Sardeshmukh, P. D., J. J. Barsugli and S. Zhang 1996: The nonlinear atmospheric response to anomalous tropical SST forcing. J. Atmos. Sci., submitted.
Whitaker, J. S. and P. D. Sardeshmukh 1997: A linear theory of extratropical synoptic eddy statistics. J. Atmos. Sci., accepted.