Printer-friendly
Technical Report (168 KB)
Purpose:
The proposal to create a revised approach
to the designation of underserved areas
is summarized in a separate document entitled,
“Proposal for a Method to Designate Communities
as Underserved.” That document outlines
the proposed methods and illustrates how
it would be used in practice. This document
is intended to provide the technical background
to how the proposed method was developed.
The principal authors of this document
are, alphabetically: Laurie Goldsmith,
Mark Holmes, Jan Ostermann, and Tom Ricketts.
We begin with five guiding principles
shaping the analysis plan. These principles
guided the application of many of the
technical approaches to creating and adjusting
the method:
- Simplicity: The new system
must be simple to understand.
- Science-based: The new system
must be based on scientifically recognized
methods and be replicable.
- Face Validity: The new system
must be intuitive and have face validity.
For example, scores that were applied
to communities should give heavier weight
to conditions that are generally accepted
to indicate need for services and which
reduce access; those scores should be
cumulative, and the scoring should readily
identify areas, populations and communities
recognized as underserved.
- Retaining designations for places
with safety net practitioners:
Federally-supported safety net resources
which are currently serving uninsured,
low-income people or persons without
reasonable access to primary care have
demonstrated that, as facilities, their
service populations qualify as underserved.
The new system should not dramatically
affect the overall number of designations
for places with safety net practitioners—in
particular, places with Community Health
Centers (CHCs) or other Federal Qualified
Health Centers (FQHCs), Rural Health
Centers (RHCs), and National Health
Service Corps personnel (NHSC).
- Acceptable performance: The
use of more contemporary data with the
proposed rule published September 1,
1998 would have resulted in the loss
of designation of a very large proportion
of areas and populations. The new proposal
should recognize that, over time there
will be changes in the factors that
predict underservice and allow for future
adjustment of the indicators. We used
many different evaluating criteria for
this guiding principle, including the
model’s ability to predict current HPSA
and MUA status, but the fundamental
criterion was whether the method fairly
and consistently identified places and
people who were in need of primary health
care and who had barriers to meeting
those needs.
The General
Approach
The overall approach for deriving an
empirical, data driven system to identify
underserved areas and populations is to
estimate the effect of demographic factors
on the population-to-practitioner ratio,
using a sample of counties as proxies
for a health care market. These effects
are then translated to a score which is
added to an adjusted ratio for a total
“need” measure. Thus, the implementation
is similar to the current IPCS or MUA
method in that it creates a “score” or
“index” of underservice, however, the
proposed system’s score is based on an
adjusted ratio that is meant to represent
an “effective” or “apparent” population
and its primary health care needs.
There are eight steps to the project,
which we divide for expository purposes
into two distinct “Tasks”.
Task One:
Calculate The Factors Affecting Ratios
(“Analysis”)
This is the analytical portion of the
project in which we explore the degree
to which observable demographic characteristics
tend to be associated with population
to provider ratios. The specific steps
in this task include:
- Create an age-sex adjusted population.
- Calculate the base population-provider
ratio for regression to determine weights
for need variables.
- Select study sample primary care
service area proxies.
- Create factor scores to control
for interactions of variables.
- Run regression models to
create weights for community variables.
Task Two:
Calculate The Scores Based On These Factors
(“Computation”)
This is the portion of the process in
which scores are assigned to geographic
areas based on the weights calculated
in Task One.
- Calculate the base population-practitioner
ratio for designation determination
- Calculate the scores for each area
based on the values for each variables
for each area and add to the ratio.
- Step 8: Compare the ratio to a
designation threshold ratio.
We describe each of these steps in detail
in the following sections.
Task 1: Analysis
Step 1: Create
an age-sex adjusted population
Using estimated visit rates from individual-level
surveys, we weight the population to create
a “base population.” In this manner,
populations can be compared across areas.
The use of these data for this adjustment
are discussed in detail in reports and
background papers for the proposal including
the report
that estimates the national impact of
the NPRM-2 proposal, “National Impact
Analysis of a Proposed Method to Designate
Communities as Underserved” dated September
7, 2001; the background paper, “Designating
Underserved Populations. A Proposal For
An Integrated System Of Identifying Communities
With Multiple Access Challenges,” which
is in draft form; and the “Executive Summary”
of the “Designating …” paper which has
been circulated in draft form to the Bureau
of Primary Health Care.
The weights are summarized in Table 1.
Table 1: Visit
weights for age-sex adjustment
|
0-4 |
5-17 |
18-44 |
45-64 |
65-74 |
75
and over |
Female |
4.046 |
2.256 |
5.007 |
5.480 |
6.710 |
8.160 |
Male |
5.164 |
2.499 |
2.867 |
4.410 |
6.052 |
8.056 |
The weighted sum of these populations
is calculated as 4.046 * (# Females 0-4)
+ 2.256 * (# Females 5-17) +…+ 8.056 *(
# Males 75 and over) and equals an age-sex
adjusted number of visits for a particular
population. Dividing this number of visits
by the mean visit rate (3.741) creates
a “base population”. Areas with equal
base populations (and equal demographics)
have an equal need for primary care visits
per year. This adjustment allows us to
compare, say, the population-based visit
differentials between an area with a high
concentration of elderly (with a higher
need for visits) and an area with a high
population of middle aged individuals
(with a lower need for visits). The visit
rates were obtained from the Medical Expenditure
Panel Survey (1996) and were calculated
for non-poor, white, non-Hispanic individuals.
Employment status, which was included
in the MEPS survey and was a significant
correlate of use of service, was also
intercorrelated with the other variables
and was not included in the final visit
calculation.
Step 2: Calculate
the base population-provider ratio for
regression to determine weights for need
variables
With the base population in hand, we
calculate the population-provider ratio
to use in the regression to determine
factor weights. The number of practitioners
is calculated as

where all practitioners are measured
in FTE units and the practitioner total
includes NPs, PAs and CNMs weighted for
relative productivity and scope of practice.
The number of practitioners calculated
for this step is different than the number
of practitioners calculated for determining
designation. The number of practitioners
used in the regression to determine weights
for the need variables only represent
those practitioners that are considered
to be the private supply. That is, the
practitioners who would choose to practice
in the community without federal support
or incentives to practice in state- or
federally-operated facilities. As such,
government practitioners (whether federal
or state) are not counted here. Community
Health Center practitioners who are not
federal employees, however, are counted
since many of these are not “placed” into
communities but are practitioners already
located in the area that are “reclassified”
as CHC practitioners for later subtraction
from the practitioner supply at a later
step. For the estimation of the formula,
an area with no practitioners is dropped
from use in the regression analysis to
determine weights for the need variables
as a ratio is undefined (not calculable).
Step 3: Select
study sample
A sample of counties and county
equivalents that serve as proxies for
a health care market are then selected
for analysis to derive formula weights.
This step was done to identify places
which functioned as primary care service
areas and which reported stable, reliable,
usable data. Many U.S. counties meet
these general qualifications and the process
selected a range of counties that met
certain criteria, including:
- populations below 125,000
- area below 900 square miles
- base population to provider ratio
below 4250
The third criterion effectively eliminated
very small counties and counties with
unusual distributions of health practitioners.
The goal was to determine the relationship
of area characteristics to practitioner
supply under “normal” conditions in order
to create stable estimates of those relationships
in order to apply them to all appropriate
populations and areas.
These sample selection criteria were
varied; we tested over 2000 combinations
in the estimation process described in
the next step to test for robustness and
sensitivity. The variations included
testing within the following ranges: population
80,000-150,000; area 700-1200 sq. miles;
ratio 3000-4250. Overall, the estimations
derived from the models were not substantially
different among the different samples
The study sample contained 1643 counties.
Counties were chosen because they are
well-defined and are not endogenous to
the current system.
Using currently designated areas would
lead to biased conclusions due to the
fact the subcounty areas are carefully
and deliberately constructed for purposes
of designation. Furthermore, dividing
a county into a subcounty-designated and
subcounty-undesignated would generate
an extremely large number of possible
observations in the analysis since the
county could be divided in many different
ways and into many subsets of county parts.
Finally, since some data are calculated
and available primarily on a county level,
measurement error is minimized by using
counties. Using other units of analysis
requires interpolating values for subcounty
and multicounty areas based on the constituent
geographic units.
Step 4: Create
factors
The proposed designation process, in
keeping with the original MUA/MUP and
HPSA approaches, identified commonly available
statistics that correlated with a small
number of primary care practitioners-to-population
ratio. The selection of the measures
was based on reviews of the scientific
literature on access to care and preliminary
work on the development of an alternative
measures of underservice conducted by
Donald H. Taylor, Jr. (Taylor & Ricketts,
1994) . Candidate statistics were also
suggested by a working group of State
Primary Care Associations (PCAs) and Primary
Care Offices (PCOs) convened by the Division
of Shortage Designation (DSD) to gather
state-level input into the process of
revising the method. The staff and leadership
of the DSD also provided extensive input
into the design. More than 20 specific
variables were suggested during this process.
Some candidate variables could not be
used, despite being highly correlated
with low access and poor health outcomes,
due to lack of availability of data for
small areas (e.g. lack of health insurance).
Ultimately, the high intercorrelations
among candidate variables restricted the
calculation to 7-9 individual indicators
(the actual number to be tested depended
upon the specific combination of variables).
The final choice of variables and the
priority for inclusion in the analysis
was based on the degree to which the variables
best reflected underlying components of
access as qualitatively assessed by the
UNC-CH team, the PCA/PCO group, and staff
of Bureau of Primary Health Care (BPHC).
The final measures consist of demographic,
economic and health status indicators
(presented in Table 2).
Demographic: Population characteristics,
especially racial and ethnic characteristics,
have been consistently shown to affect
access to primary care (Berk, Bernstein,
& Taylor, 1983; Berk, Schur, &
Cantor, 1995; Schur & Franco, 1999)
. Measures of the percent of population
that is non-White and percent of population
that is Hispanic were used to further
adjust the ratio. The inclusion of the
percentage of population older than 65
years was also included because communities
with higher percentages of elderly have
different community characteristics not
captured in the initial population adjustment.
This is likely due to the relative lack
of younger people to provide supportive
care and the fact that communities with
declining economies, especially rural
communities, have older age profiles that
combine with other factors to create overall
lower access.
Economic: Income and employment
are very strong indicators of ability
to access primary health care and to afford
health insurance (Mansfield, Wilson, Kobrinski,
& Mitchell, 1999; Prevention, 2000;
Robert, 1999) . The unemployment rate
and the percent of population below 200
percent of the poverty level were used
to further adjust the ratio.
Health Status: Certain populations
and communities have higher than average
need for health care services based primarily
on their health status independent of
other factors. Therefore, health status
measures used to adjust the ratio include
the standardized mortality ratio (General
Accounting Office, 1996) and either the
infant mortality rate or the low birthweight
rate (Matteson, Burr, & Marshall,
1998; O'Campo, Xue, Wang, & Caughy,
1997) . These special epidemiological
conditions that increase need are not
fully represented in the age-gender adjustment.
Table 2. Variables
Used in Creating Proposed Method
Demographic |
Economic |
Health
Status |
Percent
Non-white
“NONWHITE” |
Percent
population <200% FPL “POVERTY” |
Actual/expected
death rate (adj) “SMR” |
Percent
Hispanic
“HISPANIC” |
Unemployment
rate “UNEMPLOYMENT” |
Low
birth weight rate “LBW” |
Percent
population >65 years “ELDERLY” |
|
Infant
mortality rate “IMR” |
Population
density “DENSITY” |
|
These measures are highly intercorrelated.
Table 3, below shows the Pearson-product
moment correlations. The first column
shows that poverty and unemployment are
positively correlated (+0.64), meaning,
in counties with high proportions of the
population living in poverty there is
usually a higher unemployment rate. Poverty
and density are negatively correlated
(–0.55), meaning that where there is higher
density there are lower percentages of
the population living in poverty. The
correlation matrix is population-weighted.
Table 3: Percentile
Correlation Matrix
|
Poverty |
Unemp |
Density |
Elderly |
Hispanic |
NonWhite |
SMR |
IMR |
LBW |
Poverty |
1.00 |
|
|
|
|
|
|
|
|
Unemp |
0.64 |
1.00 |
|
|
|
|
|
|
|
Density |
-0.55 |
-0.21 |
1.00 |
|
|
|
|
|
|
Elderly |
0.36 |
0.28 |
-0.47 |
1.00 |
|
|
|
|
|
Hispanic |
-0.32 |
-0.23 |
0.22 |
-0.25 |
1.00 |
|
|
|
|
NonWhite |
0.10 |
0.12 |
0.22 |
-0.29 |
0.25 |
1.00 |
|
|
|
SMR |
0.57 |
0.55 |
-0.04 |
0.04 |
-0.26 |
0.42 |
1.00 |
|
|
IMR |
0.33 |
0.25 |
-0.10 |
0.08 |
-0.08 |
0.41 |
0.43 |
1.00 |
|
LBW |
0.40 |
0.37 |
0.05 |
-0.05 |
-0.14 |
0.63 |
0.69 |
0.54 |
1.00 |
Variable definitions
Variables were assigned a percentile
based on the distribution of values of
all US counties to all U.S. counties.
This allows for continuity in the use
of the proposed scores if variables are
defined differently in the future (e.g.
the poverty measure is changed to 100
percent below poverty instead of 200 percent).
It also allows policymakers a choice of
how often (or whether) to update the percentile
values without having to change the weights.
If poverty conditions improve markedly
across the nation, scores will tend to
fall unless the percentile tables are
updated. For all variables except DENSITY
the theoretically worst value corresponded
to the 99th percentile. At
first glance, it might appear that places
with very low population density would
be worse off with regard to primary care
access and health service needs. Places
with extremely high density may also have
problems caused by overcrowding and the
population density may reflect problems
that are commonly encountered in inner-cities.
For this variable there is no apparent
“right” direction for the weights. We
arbitrarily specified the functional form
such that lower population density corresponds
to a worse off (higher percentile score)
community. Accounting for the negative
effects of very high density is described
below.
We combined low birth weight and infant
mortality into one measure (called HEALTH),
defined as the maximum percentile of low
birth weight and the infant mortality
rate for a given area. This is due to
a medium level of correlation between
the two and the fact that not all areas
report both measures. Finally, the use
of the infant mortality rate in measures
of underservice is required by existing
law and there is precedent for using these
measures as rough substitutes. The original
Index of Primary Care Shortage described
in NPRM-1 of September 1, 1998 used them
interchangeably.
We defined nonwhite as the maximum of
zero or the percentile minus 40, so that
only the top (most nonwhite) 60 percent
of areas get “points” for the nonwhite
variable. In other words, all areas
less than the 40th percentile
are treated equally. There were two
main reasons for this. The first is that
many of the areas have low nonwhite percentages
(the 40th percentile is about
2.6 percent nonwhite). By not making this
adjustment, we are differentiating areas
that have little difference in the underlying
measure. The second reason is that without
this adjustment, the scores were not stable;
small differences in the definition of
this variable resulted in wide swings
in the magnitude of the nonwhite variable
when testing multiple randomly chosen
samples. We experimented with a multitude
of cutoff points (0-50 in 10 unit increments).
In the final specification, small changes
in the definition of NONWHITE had little
substantive effect.
With the corresponding percentiles in
hand, the associated scores were transformed
to a logarithmic scale so that the highest
derivative corresponded to the theoretically
worst end of the scale. For example,
the independent variable corresponding
to poverty (lnpcpov) was defined
as
so that the fastest acceleration
in the poverty score occurs at high levels
of poverty rather than at low levels.
In other words, we specified the model
to allow a greater score to accrue to
areas “moving” from the 95th
percentile to the 96th percentile
than to areas “moving” from the 5th
percentile to the 6th percentile.
All variables were assumed to have this
shape (so that the theoretically worst
values have the largest derivative).
Basing the Scores on the Population-Practitioner
Ratio
Although this approach specifies the
shape of the function as logarithmic
and this constrains the rate of change
in the scoring as variables differ from
one percentile to another, it does not
constrain the sign nor the absolute
magnitude of the parameters that create
the weights. That is, the regression
models are indifferent to whether a parameter
comes out positive or negative or how
large or small it is when the statistical
model is run to create the weights. The
magnitude is the most important parameter
of the three and will be used for estimating
the scores but the potential effects of
the size and sign of the weights must
fit into our logic of additivity of factors.
The magnitude of the weights are expressed
as a synthetic unit which cannot be compared
to any other unit—the weight for UNEMPLOYMENT,
for example, when transformed to the log-normal
form and constrained to a positive value
in the course of the estimation, is not
a “percent of workforce not working but
seeking work” but an abstract number that
describes the relative contribution of
that factor to a total access score at
that percentile of unemployment given
all the value of all the other variables
and the population structure. The final
model creates an estimate for the weight
for each set of variables using this abstract
number but that number has to be brought
back into a logical relationship with
the key unit of access we are using—the
population portion of a practitioner-to-population
ratio. The final combined sum of these
abstract values has to be adjusted back
to an interpretable relationship with
the practitioner-population ratio. This
requires that some form of restraint on
the parameter (weight) values be imposed
or the solution set may produce a “best
result” that causes one or two variables
to dominate the weighting and others to
vary from positive indicators of barriers
to access to negative in various combinations.
In an unconstrained solution of the regression
models this is, indeed, the case. There
are possible solution sets that include
mixes of positive and negative values;
in statistical parlance the functions
are “two-sided.” The logic of the scoring
system anticipated this when we stipulated
that factors which restrain use of services
by creating barriers to access, also create
subsequent higher levels of need likely
to be met by higher levels of use, use
of services that was preventable but now
necessary. In the real community, both
things are happening, an access program
is promoting appropriate utilization by
overcoming access barriers and all practitioners
are involved in caring for people who
are using the system because emergent
conditions were not treated appropriately.
The amount of the increase in use brought
about by delayed care must be added into
the reduction in use to produce a sum
of the access “problem” in a community.
To account for the “mirror” effects of
these variables, the final value, the
sum of the weights are doubled, to produce
a population estimate that is scaled to
represent the overall effect on the population
need.
Factor analysis
Because many of these measures are highly
correlated, we perform factor analysis
in order to compute factors for
the independent variables defined above.
Essentially, factor analysis provides
a method to translate highly correlated
variables into orthogonal measures to
obtain more precise estimates and minimize
the impact of multicollinearity in the
variables of interest. Often used as
an end product statistical tool, we use
it here to improve the precision of the
estimates.
Our procedure here was to decompose the
independent variables into factors and
then create scores based on these factors.
The factor scores follow in Table 3.
The bold elements are the largest weight
in the row, or on which factor the variable
weighs most heavily (except for SMR, which
has two maximum weights of almost equal
magnitude). Four factors might be interpreted
as structuring the data:
- High health risk, nonwhite
- Geo-demographics
- Economic conditions
- Hispanic
Table 2: Factor
Scores
|
Factor |
Variable |
1 |
2 |
3 |
4 |
Poverty |
-0.005 |
0.208 |
-0.423 |
0.044 |
Unemp |
-0.044 |
-0.074 |
-0.338 |
0.009 |
Elderly |
-0.039 |
0.355 |
0.021 |
-0.226 |
Density |
0.042 |
0.440 |
0.051 |
0.189 |
Hispanic |
0.018 |
-0.002 |
0.046 |
0.291 |
NonWhite |
0.408 |
-0.012 |
0.136 |
0.099 |
SMR |
0.206 |
-0.107 |
-0.226 |
-0.124 |
Health |
0.353 |
0.066 |
0.100 |
-0.046 |
Step 5: Run
Regressions
We regress the base population-to-private
supply practitioner ratio on the scores
obtained from the factor analysis (Ratio
= Factor I + Factor II … + error). By
combining the scores from the factor analysis
with the estimated coefficients from the
regression, we obtain the effect of our
underlying variables on the ratio.
As an example, the factor analysis might
yield a result such as:
Variable |
factor1 |
factor2 |
Poverty |
.2 |
.4 |
Unemployment |
.3 |
-.1 |
Which we could translate into a matrix

Suppose regressing the ratio onto these
two scores yields estimates of
Variable beta
factor1 1
factor2 -.4
which would translate to a vector

By multiplying these two matrices, we
can obtain the total effect of one variable
on the ratio:
(1) 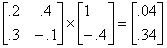
Thus, (in this simple example) the overall
effect of Poverty on the ratio is calculated
as .04 and the overall effect of Unemployment
is .34. We use the rightmost matrix for
computing the scores (see the next section)
except for one correction (see below).
Weights/Heteroskedasticity
Because the dependent variable is a ratio
with population in the denominator, we
are concerned about possible heteroskedasticity
in the dependent variable. This is the
property that the sampling variability
in the dependent variable is not constant
across the sample. Specifically, we expect
the ratio to be estimated more precisely
as the population grows. See Figure 1
below for support of this hypothesis—the
ratio tends to become less variable as
the population increases (population category
1 is the lowest population category and
population category 10 is the highest
population category). (The upper and
lower bands are the values for the 25th
and 75th percentiles). The
consequence of this violation is that
the standard errors from the regression
are biased and a more efficient estimator
may exist. As such, we weight the regressions
by the total population of the county.
Percentile for variables, 1-99
There is a question of whether we are
even dealing with a “sample” in the conventional
statistical sense. If our analysis is
composed of the population of interest,
then classical statistical inference is
a bit artificial; there is no uncertainty
if we have data on all the units of interest.
We argue that this is a sample in the
conventional sense, for reasons including
but not limited to the following:
- Measurement error occurs more often
then we expect. County population values
are estimated in 1997 and the accuracy
of provider supply is not 100 percent.
As the nation observed in the presidential
vote count in Florida, even simple computations
are not immune from error. Thus, because
the data used here are affected by measurement
error we have a sample drawn from the
possible data for the population of
counties.
- The units used here are a sample of
a much bigger population of interest.
Not only are we interested in counties
other than those included in the analysis
due to sample criteria, ultimately we
are using counties as approximations
for “health care markets” or rational
primary care service areas, whether
they follow the boundaries of a county
or not. These methods are designed
to be applied to data for future years
and the construction of the areas may
vary from one based on geography to
ZIP code boundaries.
Other considerations, such as errors
in model specification or the discrete
“lumpiness” associated with using a dependent
variable like this one provide support
for the use of factor scores.
Sampling error
in the regression
We wish to reduce the error in predicting
the designation of communities. As such,
we seek to incorporate the precision with
which the regression parameters are estimated
into the scoring procedure. As an example,
it is entirely possible, given two factors,
to have one coefficient be estimated as
100 with a standard error of 1 and the
other coefficient to be estimated as 400
with a standard error of 1000. If asked
which factor is more important, most people
would probably admit that although the
400 is a larger point estimate, the 100
is probably more important given its statistical
significance. As such, the regression
estimates are adjusted for the statistical
significance by the algorithm defined
below.
[1]
- Obtain the variance-covariance matrix
V of the parameter estimates
from the regression.
- Compute the weighting matrix W
defined as the inverse of the Cholesky
transformation of a zero matrix except
for the diagonal, which consists of
the diagonal of V. (This is
identical to a zero matrix with diagonal
elements equal to the reciprocal of
the standard errors of the parameter
estimates).
- Transform the vector of parameter
estimates (omitting the constant) b
by b* = b *W * number of
factors/trace(W). The trace()
portion of the expression ensures the
weights sum to the number of factors.
- Compute F = S b*
as above.
As an example, return to the hypothetical
results for poverty and unemployment above.
Suppose the (estimated) variance-covariance
matrix from the regression was
then
so
(2)
The estimated scores in equation (2)
differ from those obtained in equation
(1) (page 17) due to the weight. Because
the regression estimate for the first
factor is estimated with roughly three
times the precision as the estimate for
the second factor (5/1.42 »3), the estimate
for the first factor (1) is weighted more
heavily than the estimate for the second
factor (-.4). In this case, this has
the end result of increasing the scores
from .04 to .24 for poverty and .34 to
.4844 for unemployment. Vector F
is the scoring vector used in the next
step.
Although the process for obtaining matrix
F is complex and multi-stage the
process was completed for all possible
values of the variables. Having done
this, data describing a service area can
be translated readily into percentile
scores using a look-up table, a simple
spreadsheet, or a web-based application.
This parallels the existing MUA scoring
process. Applicants do not need to perform
Cholesky transformations or any other
mathematical calculations.
Task 2: Computation
Step 6: Calculate
the base population-provider ratio for
designation determination
Using the same age-sex adjusted population
from Step 1, we calculate the population-practitioner
ratio. All primary care practitioner
FTEs in the area are counted to initially
determine designation, this is termed
the “Tier 1 designation.” For applicants
not meeting the threshold criterion, the
FTEs for practitioners who are supported
by safety net programs (e.g., NHSC providers,
J-1 visa practitioners, CHC providers)
are subtracted from the supply total and
the applicant ratio is compared to the
threshold. That step is termed “ Tier
2 designations.”
Step 7: Calculate
Scores
With row vector F in hand, we
then turn to computing scores for geographical
units. We compute the ratio of population
to providers using the algorithm outlined
above. We use the percentile scores as
computed above for the counties. See
the document “Completing the NPRM2 Application”
for these percentiles.
We then calculate the score for the communities
and add this score, upweighted by 2 to
account for the 2-sided properties of
the regression estimates so the total
score for the community equals
ADJUSTED RATIO (or “INDEX”) = RATIO
+ 2 * SCORE
This is the total score for the community
and determines its designation status.
The applicants never see the regression
multiplier; it is embedded in the tables.
Because the use of the multiplier for
the score is applied at this stage of
the process, it may be seen as an ad-hoc
adjustment. The statistical logic for
this has been described above, the policy
logic for applying this adjustment is
supported by these points:
- The multiplier is used to account
for the fact that the existing measures
and processes including: the HPSA formula,
the IPCS/MUA formulae, and the practical
application of the CHC/RHC clinic placement
process—all recognize the importance
of the basic population-to-practitioner
ratio in determining need. Indeed,
some simple models run on the study
sample provide evidence that the multiplier
should be closer to 10 rather than 2 if
the goal were to include every area containing
a CHC under the proposed designation process
(this assumes that the presence of a CHC
is an indicator of need in and of itself
as opposed to the result of the calculation
of pre-existing unmet need). The IPCS
mechanism provided for a maximum score
from the population-practitioner ratio
of 35 points. The maximum score available
from other factors (poverty 35 points,
IMR/LBW 5 points, minority 5 points, Hispanic
5 points, LI 5 points, density 10 points
= 65 points) are, collectively, almost
twice that in terms of potential contribution.
Thus, the weighted contribution of the
factors besides the ratio is roughly twice
that of the ratio itself. Multiplying
the ratio denominator by two intensifies
the relative effect of the underlying,
basic population to practitioner ratio
in the designation process providing continuity
with prior policy.
- The multiplier functions as a scale
/weighting factor. The score has a much
smaller variance than the ratio. This
is not just an annoyance—it is used
to generate a prediction, and thus will
have smaller variance than the dependent
variable. The dependent variable and
the score used here have some sort of
meaning, a person per provider, although
the various adjustments make this unit
of measurement not as meaningful as
we might think. One alternative we
considered is rescaling the ratio and
the score into z-scores and using
these standardized measures rather than
the unscaled measures. This rescaling
would involve multiplying the score
by a larger factor than the ratio.
- The multiplier helps control for the
(observed) low ratios in, (eg, metro)
areas with high scores. The following
example illustrates this:
- The multiplier fills a statistical
role. The score is (likely) more stable
across years; e.g., if one physician
moves out of a rural area, the ratio
varies dramatically. The score is not
going to change drastically across years.
Thus, it should be given more weight.
- The multiplier creates a standard
which designates roughly the same number
of people as the IPCS and the current
HPSA designations.
- It performs better than without the
doubling. Although this particular
argument has little theoretical basis,
it is still compelling
Why is a portion of the density score
function negative?
The astute reader will note that the
constant from the regression was dropped
and never used. The reason for this is
that the constant has no clear meaning
in this context. We decided to norm the
scores so that the minimum score—that
is, the best area in the country—was zero.
Thus, although in theory an area
could receive a negative score if it had
very favorable demographics and had a
high population density, in practice
no area had a negative score (by definition).
Step 8: Compare
to Threshold
Areas are designated if and only if the
“adjusted ratio” (or ratio+score) is greater
than 3000. This threshold was adopted
for its reflection of the clear need for
a single full-time equivalent primary
care physicians, its consistency with
prior threshold values, and its familiarity
to stakeholders.
Areas with No Practitioners
The problem of how to treat areas with
zero providers emerged early in the process
of ranking areas as medically underserved.
There is an informative treatment of the
phenomenon in Black and Chui (1981).*
For areas with zero providers, we have
not made any firm recommendations and
have treated them in one of three ways
for various parts of the analysis
- Every area with zero providers automatically
gets an adjusted ratio of 3000 (which
guarantees them designation), to which
a score for community need indicators
are added. This results in all areas
having a NPRM2 score, including areas
with zero providers. This method was
used in early tabulations and compilations.
- Automatically designate areas with
zero providers without assigning an
adjusted ratio or a score for community
need indicators. Therefore, areas with
zero providers will not have a NPRM2
total score. This has occurred when
calculations and tabulations of the
database using the NPRM2 scoring system
was applied. The places with no score
were dropped. This method was used
in the final impact analysis.
- Assigning an arbitrarily small FTE
to the area, such as 0.1 to create a
score that is primarily dependent upon
the denominator population. This was
used only in selected tests of the scoring
system as an alternative.
Addition to Technical Document:
EXAMPLE OF HOW TO CALCULATE SCORES
FOR HIGH NEED INDICATORS
The chart below shows for how the scores
for each individual factor for one county
are determined, using the tables below;
look up the percentile for each actual
value (i.e. 49.8% @ <200% poverty is
in the 79th percentile on Table
IV-2; the 79th percentile for
poverty from Table IV-3 shows 466 additional
need factor should be added-very high
poverty is correlated with greater need
and less access to care). The same process
is followed for each of the nine need
factors to get total score to be added
to the effective barrier free ratio calculated
above
|
Wichita
County, KS |
HIGH
NEEDINDICATORS |
|
%<200%
POVERTY [U1] |
49.8% |
PERCENTILE |
79 |
SCORE |
466 |
UNEMPLOYMENT
RATE |
3.95 |
PERCENTILE |
31 |
SCORE |
43 |
%
65+[U2] |
15.6 |
PERCENTILE |
54 |
SCORE |
42 |
POPULATION
/SQ MILE[U3] |
3.767 |
PERCENTILE |
8 |
SCORE |
475 |
%HISPANIC |
16.36 |
PERCENTILE |
91 |
SCORE |
195 |
%NON-WHITE |
1.18 |
PERCENTILE |
22 |
SCORE |
0 |
DEATH
RATE |
.673 |
PERCENTILE |
182 |
SCORE |
.8 |
LBW
(low birth weight) |
7.77 |
PERCENTILE |
70 |
SCORE |
86 |
IMR
(infant mortality rate) |
Na |
PERCENTILE |
|
SCORE |
|
TOTAL
SCORE TO BE ADDED |
1308 |
[1] An alternative treatment would be
to discard any statistically insignificant
estimates. We have strong conceptual
biases against employing such stepwise
procedures.
* Black, R. A., and Chui, K.-F. (1981).
Comparing schemes to rank areas according
to degree of health manpower shortage.
Inquiry, 18(3), 274-280.
[U1]Is this level set high enough to
capture the working poor, who are disproportionately
represented among the uninsured?
[U2] Since HRSA is already adjusting
for age and gender in “step 1,” why is
HRSA adjusting for age again here in “step
2”?
[U3] Please explain why low population
density is a good proxy for higher healthcare
need and/or utilization and/or demand
for primary care.
Prepared for the
Division of Shortage Designation
Bureau of Primary Health Care
Health Resources and Services Administration
Department of Health and Human Services
Under a Cooperative Agreement with the
Office of Rural Health Policy (HRSA) (1
UIC RH 0027-01)
Prepared by the Cecil G. Sheps Center
for Health Services Research
The University of North Carolina at Chapel
Hill |