2002 Progress Report: Development of Techniques for Assimilating GOES Satellite Data into Regional-Scale Photochemical Models
EPA Grant Number: R826770Title: Development of Techniques for Assimilating GOES Satellite Data into Regional-Scale Photochemical Models
Investigators: McNider, R. T. , Biazar, A. P. , Norris, W. B.
Current Investigators: McNider, R. T. , Biazar, Arastoo , Norris, W. B.
Institution: University of Alabama in Huntsville
EPA Project Officer: Shapiro, Paul
Project Period: July 1, 1998 through June 30, 2001 (Extended to January 17, 2004)
Project Period Covered by this Report: July 1, 2001 through June 30, 2002
Project Amount: $404,127
RFA: Air Pollution Chemistry and Physics (1998)
Research Category: Engineering and Environmental Chemistry
Description:
Objective:The objective of this research project is to enlist the methods of satellite remote sensing to reduce uncertainty in the cloud and soil-moisture information, which meteorological models pass to their photochemical counterparts.
Progress Summary:The focus of the work for this year was to finish analyzing the results from the MM5 simulations, investigate the cause of dry bias in the satellite assimilation method, and improve our techniques accordingly. The following discussion summarizes our progress.
During this year (2002), we continued our efforts to refine and improve our satellite assimilation techniques within MM5. The MM5 simulations that are discussed here span a period of 52 days during the summer of 1999, from June 28 through August 20. This period coincides with the Southern Oxidant Study (SOS) intensive field campaign during the summer of 1999. During the previous years of this project, surface properties were retrieved from satellite images and control MM5 simulations were performed. In addition, MM5 source code was modified for the assimilation of satellite retrievals, preprocessors were developed to prepare the satellite data for use in MM5, and finally utilizing the satellite retrievals, MM5 simulations with satellite assimilation were performed. The results from these simulations were less than satisfactory. The model crashed during the simulations for the 8-km nest, and unreasonable skin temperatures were observed in the 32-km runs.
During the period of this report, we continued to perform simulations with satellite assimilation, analyzed the results, and investigated the deficiencies in our assimilation method. We revisited the assumptions used in McNider, et al., 1994, and examined the implementation of this technique in MM5. The process that led us to revisit our technique was costly. First, due to undesirable results, we noticed the problem of cloud contamination in the satellite retrievals. This problem was fixed and the simulations were redone. This time, the examination of the results indicated that although there was an overall improvement in the model results with respect to skin temperature predictions compared to the control run, at certain grid points, the results were unreasonable. Therefore, we had to redo part of the simulations and look at the problem in detail. We also had a disk crash on our computer system that delayed our efforts. The following details the steps taken in this process.
MM5 Configuration. The simulations were configured for a 32-km grid resolution
over the Continental United States and 8-km resolution over the Southeast United
States. MM5 version 3.4, with some modifications for incorporating satellite
information, was used for the simulations presented here. The simulations (with
satellite assimilation) covered the period of June 28 through August 20, 1999.
The satellite retrievals used in this study are available from the National
Space Science and Technology Center (NSSTC) Web Site at http://www.ghcc.msfc.nasa.gov/irgrp/1999_epa_study .
The model is configured to utilize the National Centers for Environmental Protection (NCEP) Reanalysis data products, Dudhia's simple ice parameterization for explicit moisture scheme, the Kain-Fritsch scheme for cumulus parameterization, the Hong-Pan (MRF) scheme for planetary boundary layer, the simple cloud-radiation scheme for atmospheric radiation, and the 5-layer soil model for the surface temperature scheme. No shallow convection was allowed in these simulations. The assimilation period for the skin temperature tendencies is from 7:00 a.m. to 10:30 a.m. Surface insolation and albedo was assimilated for the entire daytime periods.
Discussion. Our first simulations with satellite assimilation indicated that shortly into the run, the western part of the domain (Continental United States) completely dried, while the northeastern and southeastern parts of the domain exhibited pockets of increased moisture availability. These features became more pronounced as the simulations proceeded, and by mid July, this pattern became permanent and persisted until the third week of July. Towards the end of July, as the Eastern United States experienced a warmer period, the model predicted drier surface and higher skin temperatures for the east. By the end of the simulations, most of the domain was dry, with isolated pockets of moist surface in the northeast, and to a lesser degree in the southeast.
Figure 1 exhibits the results for July 15, 1999. Model skin temperatures from the satellite assimilation simulation show a better agreement with the satellite retrievals compared to the control run. The large area of missing data in the satellite retrieval is due to presence of clouds, where retrieval is not possible. What is noticeable is that the control simulation predicts cooler skin temperatures in the northwestern part of the domain, while the assimilation run captures both the spatial pattern and the magnitude of skin temperatures correctly. The assimilation run shows higher skin temperatures in northern Nevada and lower temperatures for northern California, that are in agreement with satellite retrievals. The assimilation run also is in better agreement with satellite observations for the South/Southeast, especially over Texas, predicting the observed spatial gradient of skin temperatures. However, the model predicts higher temperatures for Missouri, Iowa, Illinois, and Wisconsin.

Figure 1. Results for July 15, 1999, at 20:00 GMT. (a) Satellite retrievals of skin temperature; (b) adjusted moisture availability field; (c) model skin temperatures from control simulation; (d) model skin temperatures from satellite assimilation run.
Overall, the control simulations suffer from a cold bias, while the assimilation runs (although improving the predictions in some areas) suffer from a warm bias compared to the observations. Part of the reason for this dry bias can be traced back to our satellite assimilation technique. The adjustment term for moisture availability can be expressed as:
![]() |
(1) |
where dTG/dt is the surface temperature tendency,
Cb the surface heat capacity (per unit area),
and E is the latent heat flux. Subscripts m and s represent
model and satellite quantities respectively. As it is seen in Equation 1, having
the surface heat capacity constant, a reduction in moisture availability will
reduce the moisture flux. A reduction in E will in turn increase the
adjustment factor and can cause more drying. The increase in the model tendency
can counteract this effect if it is large enough. However, from our experience,
such increases are not adequate. Also, from Equation 1, it is obvious that changing
the surface heat capacity in connection with moisture availability will be the
balancing factor.
There were other problems in the simulations. Certain grid points exhibited unrealistic behavior with respect to skin temperature. Some grid points became unrealistically hot, while others became unrealistically cold. We realized that some of this behavior was due to cloud contamination in the satellite retrievals. Therefore, we reran the model with appropriate remedies to alleviate this problem. We also realized that there are large variations in the satellite-retrieved surface albedos from the morning hours to midday, with midday albedos being too low (lower retrieved albedo in turn can lead to higher retrieved insolation). Higher insolation coupled with low albedos caused more energy to be absorbed by the surface and led to a warm bias in the satellite assimilation runs. The problem was attributed to geostationary satellite server (GOES) sensor degradation, which can be up to 30 percent. Due to this finding, the new retrievals at the Global Hydrology and Climate Center (GHCC) are correcting for sensor degradation.
The 8-km simulations crashed around July 20. Investigating the cause of the crash, we realized that at certain locations earlier in the simulations, the skin temperatures started to deviate sharply from their normal trend. As the simulations continued, the error increased and propagated to adjacent areas. Finally, the model crashed at a later time in the simulation. Figure 2 shows the results from two grid points over the southeast that exhibited unrealistic behavior. As the figure shows, the skin temperatures start to show large diurnal oscillations after July 7, and by July 23, the skin temperatures are either unrealistically warm (in one case) or cold (in another case).

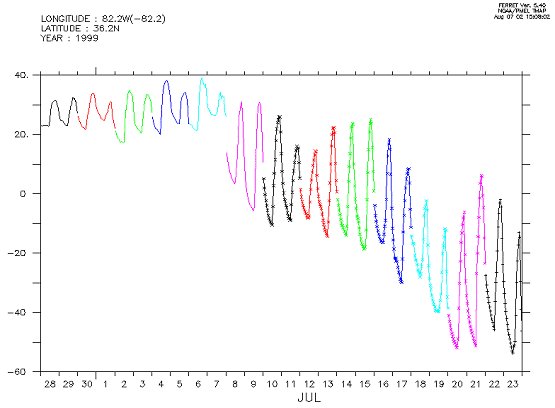
Figure 2. Model Skin Temperatures From Two Grid Points That Exhibited Unrealistic Behavior. Both points are over the Southeast.
Investigating the cause of such behavior led us to discover few minor bugs in the code. After fixing the bugs in the code and rerunning the model, problems persisted. We started looking at the results at each time step. Figure 3 shows the model behavior at each time step for skin temperature tendency and corresponding moisture availability. This figure sheds light on several problems in the implementation of our technique within MM5.

(b)

Figure 3. MM5 Skin Temperature Tendency Compared to Satellite Retrieved Tendency. (a) Moisture availability; (b), Moisture availability at each model time step for a selected grid box.
The model tendency is highly sensitive to changes in moisture availability. This is evident from the high amplitude oscillations of the model tendency. At the start of the assimilation period, because the model tendency is much higher than the satellite tendency, the moisture availability (M) is set to a wet surface. This causes a rapid cooling effect in the model to the point that the model tendency reaches the satellite value in few time steps. At this point, further cooling in the model causes a decrease in M (drying of the surface). Over-adjustment to M leads to oscillations in tendency until the surface is completely dry. We also observe that at the beginning of each assimilation period (1 hour), there is a sharp increase in M followed by a gradual decrease.
The cause of this behavior can be explained by revisiting the energy balance equation.
![]() |
(2) |
First, in our technique, we represent a continuous function by a time series
of satellite-retrieved insolations. Second, the insolation remains constant
until the next satellite record is available (1 hour). Therefore, at the start
of the period, when the satellite-retrieved insolation is higher than the model
value (in the model it was calculated every 45 minutes), it leads to an increase
in the model tendency that in turn introduces a wet bias in our technique. However,
because this forcing term remains constant for 1 hour, the terms on the right-hand
side of Equation 2 tend to reach a new balance. This means that the model tendency
is inclined to converge to zero. Thus, our technique will dry the surface to
compensate for the disagreement with the satellite tendency.
In Figure 3, this behavior is captured well. For the second hour of assimilation, M is reduced to compensate for the difference between model and satellite tendency. However, halfway through the assimilation period, when surface is completely dried up and our technique ceases to be effective, model tendency deviates from the satellite and continues to decrease. Because the increase in the insolation during the early hours of the day (for the clear sky pixels) is almost linear, then interpolating the successive satellite retrievals can represent the continuous function of insolation. We have chosen this approach as a remedy for the above problem.
Also in Figure 3, it is obvious that due to high amplitude oscillations in model tendency, the calculated adjustments to the moisture availability are large and cannot be justified. These are the conditions that our basic assumptions for our satellite technique are violated. Therefore, because there is no correction to the surface-heat capacity, the adjustment we make to M must be constrained by some other means.
Finally, by representing as a continuous function and constraining
the magnitude of the adjustments made to M, we were able to make improvements
to our technique. Figure 4 exhibits the results from the modified version of
our technique for the same model grid point as in Figure 3.
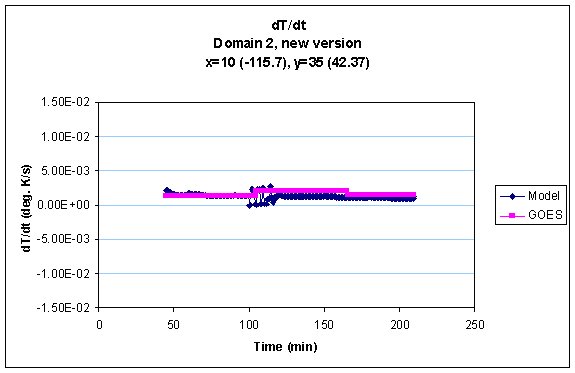
(b)

Figure 4. Results From the Modified Version of Satellite Assimilation Technique for the Same Grid Point as in Figure 3. (a) Skin temperature tendency compared to satellite-retrieved tendency; and (b) moisture availability, at each model time step for a selected grid box.
References:
McNider RT, Song AJ, Casey DM, Wetzel PJ, Crosson WL, Rabin RM. Toward a dynamic-thermodynamic assimilation of satellite surface temperature in numerical atmospheric models. Monthly Weather Review 1994;122:2784-2803.
Future Activities:Because we spent more time on unforeseen problems in our satellite assimilation techniques within MM5 (as mentioned above), we were not able to perform any photochemical (CMAQ) simulations. Another bottleneck hindering our photochemical modeling effort has been the unavailability of emissions. Recently, we have received emissions from Tennessee Valley Authority (TVA), our collaborator, for the first 10 days of July. The next step in this project will be our photochemical modeling activity.
Journal Articles:No journal articles submitted with this report: View all 5 publications for this project
Supplemental Keywords:ambient air, precipitation, Southeast, oxidants, atmospheric chemistry, geostationary satellite server, GOES, mesoscale meteorology, particulate matter, PM2.5, actinic flux, air modeling, air quality standards, biogenic emissions, boundary layer, cloud condensation, latent heat flux, meteorology, photochemical processes, photolysis wavelength, regional scale, remote sensing. , Air, Scientific Discipline, RFA, Engineering, Chemistry, & Physics, Ecology, Environmental Engineering, particulate matter, Environmental Chemistry, Environmental Monitoring, cloud condensation, photochemical processes, biogenic emissions, GOES satellite, regional scale, remote sensing, PM 2.5, PM2.5, meterology, air quality standards, air modeling, boundary layer, soil, photolysis wavelength, actinic flux
Relevant Websites:
http://www.ghcc.msfc.nasa.gov/irgrp/1999_epa_study
Progress and Final Reports:
1999 Progress Report
2000 Progress Report
2001 Progress Report
Original Abstract
Final Report