2001 Progress Report: Development of Techniques for Assimilating GOES Satellite Data into Regional-Scale Photochemical Models
EPA Grant Number: R826770Title: Development of Techniques for Assimilating GOES Satellite Data into Regional-Scale Photochemical Models
Investigators: McNider, R. T. , Biazar, A. P. , Norris, W. B.
Current Investigators: McNider, R. T. , Biazar, Arastoo , Norris, W. B.
Institution: University of Alabama in Huntsville
EPA Project Officer: Shapiro, Paul
Project Period: July 1, 1998 through June 30, 2001 (Extended to January 17, 2004)
Project Period Covered by this Report: July 1, 2000 through June 30, 2001
Project Amount: $404,127
RFA: Air Pollution Chemistry and Physics (1998)
Research Category: Engineering and Environmental Chemistry
Description:
Objective:The objective of this research project is to enlist the methods of satellite remote sensing to reduce the uncertainty in the cloud and soil-moisture information, which meteorological models pass to their photochemical counterparts.
Progress Summary:The focus of the work for this year was to finish our MM5 simulations with and without satellite assimilation for the summer of 1999, and to start the data analysis. Our efforts are summarized in the following discussion.
Discussion. The period of study was from June 28, 1999 to August 15, 1999. This period coincides with the Southern Oxidant Study intensive field campaign during the summer of 1999. During Year 1 of this project, we retrieved surfaced properties from satellite images. Control MM5 simulations also were performed.
This year, we developed the necessary preprocessors for MM5 to interface with the satellite products, and we carried out MM5 simulations with satellite assimilation for the period of the study. The satellite retrievals also were shared with the U.S. Environmental Protection Agency (EPA). The photochemical modeling was delayed because of the lack of emissions input for the Congestion Mitigation and Air Quality (CMAQ) program.
In the following report, we present the results from the MM5 simulations. The focus of this study is the assimilation of satellite information in MM5 to evaluate the impact of inclusion of remotely sensed information on the prediction of meteorological fields.
MM5 Simulations With Satellite Assimilation. Similar to control simulations, MM5 was configured for a 32-km grid resolution over the Continental United States and 8-km resolution over the Southeastern United States. MM5 version 3.4 (with some modifications for incorporating satellite information) was used in these simulations.
The modifications to MM5 include the addition of subroutines that ingest satellite information, subroutines that replace standard MM5 routines with additions for processing and assimilating satellite information, and modifications of many routines in the MM5 modeling system to allow for additional arrays to be carried within the system. Also, preprocessors were developed to process the retrievals and prepare them for use in MM5. The preprocessor calculates the surface temperature tendencies from the retrieved skin temperature retrievals, checks the data integrity, flags the data for bad and missing information, and inserts a time record consistent with the MM5 time.
The 32-km domain (127x181x30) covers 127 grid points in south-north direction, 181 points in east-west direction, and 30 vertical layers. It is centered at 40-north, 100-west, using Lambert-Conformal map projection. The 8-km domain (145x145x30) over the Southeastern United States, extends 145 grid points in each direction horizontally, and 30 layers vertically.
The model was configured to use Dudhia's simple ice parameterization for explicit moisture scheme, Kain-Fritsch scheme for cumulus parameterization, Hong-Pan (MRF) scheme for planetary boundary layer, simple cloud-radiation scheme for atmospheric radiation, and five-layer soil model for the surface temperature scheme. No shallow convection was allowed in these simulations.
In these runs for the early part of the day, from 7:00 a.m. to 10:30 a.m., satellite retrievals for surface temperature tendencies were used to adjust the soil moisture availability in the model. Furthermore, the adjusted moisture availability is carried over for the rest of the day. It remains unchanged until the following morning, when it is time for another assimilation. Also, for the entire period of the day, the model radiation field at the surface and surface albedos were replaced with the retrieved values from satellite observation whenever/wherever the data were available.
Satellite Assimilation. The surface energy budget in MM5 based on a "force-restore" method developed by Blackadar (Blackadar, 1979; Zhang and Anthes, 1982) can be represented by Equation 1:
![]() |
(1) |
where dTG/dt is the surface temperature tendency,
C
is the surface heat capacity (per unit area), RN
is the net radiation (including incident shortwave, incoming atmospheric longwave,
and outgoing longwave), H is the sensible heat flux, G is the
soil heat flux, and E is the latent heat flux.
Following McNider, et al. (1994), we invoke the critical assumption that all of the terms in the model's surface energy budget are the same as for the actual energy budget observed by the satellite, except for the latent energy term E. This is based on the idea that we know the least about evapotranspiration, and that in the mid-morning the energy budget is most sensitive to moisture availability (Wetzel, et al., 1984). With this assumption, we take the difference of the surface energy budgets for the model and satellite to obtain:
![]() |
(2) |
where (dTG/dt)s is calculated from hourly Geostationary
Operational Environmental Satellite (GOES)-derived surface temperature products
retrieved at model grid points. Hereafter, we represent the model quantities
by the subscript m and satellite quantities by s. Allowing
![]() |
to represent the adjustment needed to bring the model moisture flux in agreement with the satellite inferred flux; (2) can be written as:
![]() |
(3) |
The way in which the moisture flux is adjusted within the model is dependent
upon the flux formulation used. In MM5, surface specific humidity is not a prognostic
variable. Therefore, we adjust what is called the moisture availability parameter
(M), which represents the fraction of possible evaporation for
a saturated surface (equal to 1 over open water and 0 over a non-evaporating
surface). The latent heat flux in the Blackadar boundary layer scheme in MM5
is given by:
![]() |
(4) |
where qsat(Tg) is
the saturation mixing ratio of the surface, qa
is the mixing ratio of the air immediately above the surface, k is the
von Karman constant, u* is the frictional velocity,
za is the height of the lowest model layer, zl
is the depth of the molecular layer, ka is a background
molecular diffusivity, and h is a non-dimensional stability parameter
for heat and water vapor. To obtain the satellite-inferred moisture availability,
we solve (4) for using the satellite-inferred latent flux as derived from (2)
and (3), and get the satellite inferred moisture availability:
![]() |
(5) |
In addition to the recovery of moisture availability, as mentioned above, the
GOES derived net solar radiation also is assimilated into the MM5 surface energy
budget via direct insertion. This is critical to meet the assumptions of the
moisture recovery technique. It also is critical in getting the variation in
surface insolation correct during the dynamical initialization.
Results and Discussions. The simulations for both the 32-km and 8-km domains were performed for June 28, 1999 to August 20, 1999. The satellite assimilation runs finished on August 10. Figures 1 and 2 illustrate the extent of each domain, the MM5 land use categories, and the volumetric heat capacities used in the simulations. In the control simulation, the albedo, the heat capacity, and the moisture availability are prescribed at the beginning of the simulation based on the land use category, and remain constant for the entire simulation.


Figure 1. MM5 24-Land Use Category and the Volumetric Heat Capacity for Domain 1 (32-km Domain)
Figure 2. Same as Figure 1, but for the 8-km Domain
Shortly after the start of the run, a pattern appears in the assimilation simulations for domain 1 that remains consistent for the rest of the simulation period. We see a drying for most of the domain with the exception of an increase in moisture availability in the southeastern and northeastern part of the domain. Also, the areas with increased moisture availability are noisy. Figure 3 exhibits the moisture availability on July 9 for domain 1 both for the control run and the assimilation run. We also should note that the model moisture availability does not show much structure, and for most of the areas to the west of Minnesota, Iowa, Missouri, Arkansas, and Louisiana it is low to begin with. This means that if there is a cold bias in the model for these areas, we expect the moisture availability to reach its lower limit in a short period of time. Also, changing moisture availability alone may not be enough to bring the model tendency in agreement with the satellite tendency (perhaps recovering the slab heat capacity can help in such a situation).

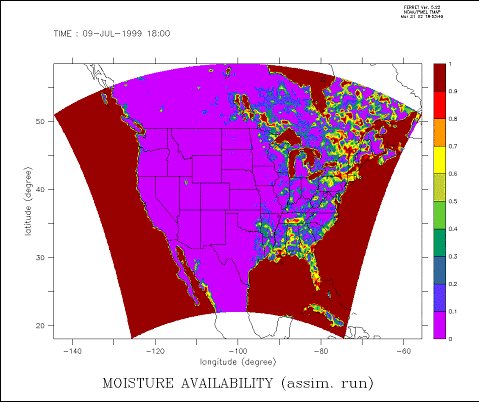
Figure 3. Moisture Availability for Domain 1, (a) Control Run, (b) Assimilation Run
In the implementation of the satellite assimilation in these simulations, the model tendency also was replaced with the satellite tendency during the assimilation period. This means that even in situations where the adjusted moisture availability is out of the valid range and has to be reset to its limits, the new model skin temperature is being calculated according to the satellite tendency. Although this implementation was not intended in McNider, et al., 1994, these runs caused the model temperature variation in the assimilation period to agree with the satellite tendency. Figure 4 exhibits the difference in moisture availability between the assimilation run for July 9, 1999, and the control run. This figure shows the contrast between the eastern and western part of the domain for this period. The negative values in this plot indicate the drying of the surface, while the positive values indicate the increase in the moisture availability in the assimilation run.

Figure 4. Difference in Moisture Availability Between the Assimilation Run and Control up to July 9, 1999, for Domain 1
Figures 5 and 6 show the moisture availability for the control run, the assimilation run, and the difference between the two runs for the same period as above but for domain 2 in this case. The assimilation run in this case produces a noisier field, with most of the values being at either upper or lower limit of the moisture availability. There are several reasons for this behavior that will be discussed in the following.


Figure 5. Moisture Availability for Domain 2, (a) Control Run, (b) Assimilation Run
Figure 6. Difference in Moisture Availability Between the Assimilation Run and Control up to July 9, 1999, for Domain 2
Figure 7 shows a snap shot of the skin temperature difference between the assimilation run and the control run. As expected, the temperature differences follow the pattern that we have seen in the moisture availability fields. That is, the assimilation run is warmer than the control run in the areas where we have dried the surface, and cooler in the areas that we have increased the moisture availability.


Figure 7. Difference Between Skin Temperatures From Assimilation Run Versus Control Run for July 9, 1999, at 18:00 GMT
Replacing the model insolation with the satellite-retrieved insolation in these runs has helped to correct the forcing term in Equation 1. Comparing the insolation fields from the control run to the assimilation run exhibits the poor model performance in the prediction of the clouds. Figures 8 and 9 show the insolation fields from the control run and the assimilation run for July 9 at 6:00 p.m., and also show the difference between the two simulations. A pattern seen here is that the model not only could miss some of the clouds altogether, or have them at the wrong place at the wrong time, but that the model also is too opaque for the clouds that are predicted at the right place and at the right time. For example, in Figure 8 the model has predicted the cloud mass in the northeastern part of the domain, but the clouds are so thick that almost no light is passing through them. In the satellite observations, more of the shortwave radiation is reaching the surface. However, in the southeast and the northwest parts of the domain, the model is predicting clouds that cannot be seen from the satellite.


Figure 8. Surface Incident Shortwave Radiation for Domain 1, (a) Control (b) Satellite-Retrieved
Figure 9. The Differences Between the Satellite-Retrieved Insolation and MM5 Insolation in the Control Run
Although the previous studies implementing the above satellite assimilation method for short-term forecasts had shown improvements in model-predicted 2-m air temperatures, we encountered many problems in applying this method to a long simulation. Investigating the cause of these problems has led us to make improvements to our methods.
Key findings are:
(1) We realized that there are large variations in the satellite-retrieved surface albedos from the morning hours to mid-day, with mid-day albedos being too low (lower retrieved albedo in turn can lead to higher retrieved insolation). Because we are replacing model albedo and insolation with the satellite-retrieved values for the entire day, this problem causes more energy being absorbed by the surface and can lead to a warm bias in the satellite assimilation runs. The problem with low albedo retrievals was attributed to GOES sensor degradation that can reach up to 30 percent. Because of this finding, the new retrievals at the Global Hydrology and Climate Center (GHCC) are correcting for sensor degradation.
(2) Our satellite assimilation method for 1999 simulations is replacing the model insolation with the satellite-retrieved values during the temperature tendency assimilation (between 7:00 a.m. to 10:30 a.m.). Therefore, the insolation used in the model will remain constant until the next satellite observation is available (usually 1 hour). This is similar to the treatment of the radiation field in MM5, in which the user decides how frequent the radiation calculations are to be performed. Because in Equation 1, the main forcing term is the radiation term, it is natural that by keeping this term constant, the skin temperature tends to converge to a value that maintains a balance in the flux terms. This means that the model tendency is likely to go to zero and therefore cause a dry bias in our method. Another side effect of this method is the sharp increase in the moisture availability in the beginning of the assimilation period that is followed by a prolonged decrease. We have corrected our method by interpolating for the insolation in time at each model time step, so that the forcing term in equation 1 is consistent with the land surface temperature (LST) tendency calculated from satellite-retrieved LST.
(3) Equation 1 is extremely sensitive to moisture availability changes and can become unstable. This high sensitivity/instability causes oscillation in the model LST tendency and produces large disagreements between the model and the satellite tendencies. At times the disagreement is so large that the adjustment term ( ) causes the adjusted moisture availability to become negative or exceeds its upper limit, 1. We have modified our method to constrain .
(4) We are still faced with a dry bias in the satellite assimilation method. We think that this could be because of the error in the slab heat capacity. We plan on recovering the heat capacity from the afternoon LST retrievals.
We are in the process of evaluating the results from these simulations.
References:
Blackadar AK. High resolution models of the planetary boundary layer. Advances in Environmental and Scientific Engineering, Volume 1. New York: Gordon and Breach, 1979.
McNider RT, Song AJ, Casey DM, Wetzel PJ, Crosson WL, Rabin RM. Toward a dynamic-thermodynamic assimilation of satellite surface temperature in numerical atmospheric models. Monthly Weather Review 1994;122:2784-2803.
Wetzel PJ, Atlas D, Woodward RH. Determining soil moisture from geosynchronous satellite infrared data: a feasibility study. Journal of Climate and Applied Meteorology 1984;23:375-391.
Zhang D, Anthes RA. A high-resolution model of the planetary boundary layer: sensitivity tests and comparison with SESAME-79 data. Journal of Climate and Applied Meteorology 1982;21;1594-1609.
Future Activities:Our future work will focus on further investigating the root causes of the problems that we encountered with the assimilation technique (as listed above), finishing the data analysis, and continuing our assimilation work within the air quality model.
Journal Articles:No journal articles submitted with this report: View all 5 publications for this project
Supplemental Keywords:ambient air, precipitation, southeast, oxidants. , Air, Scientific Discipline, RFA, Engineering, Chemistry, & Physics, Ecology, Environmental Engineering, particulate matter, Environmental Chemistry, Environmental Monitoring, cloud condensation, photochemical processes, biogenic emissions, GOES satellite, regional scale, remote sensing, PM 2.5, PM2.5, meterology, air quality standards, air modeling, boundary layer, soil, photolysis wavelength, actinic flux
Progress and Final Reports:
1999 Progress Report
2000 Progress Report
Original Abstract
2002 Progress Report
Final Report