The Impacts of Bypasses on Small- and Medium-Sized Communities: An Econometric Analysis
SIVARAMAKRISHNAN SRINIVASAN
KARA MARIA KOCKELMAN*
The University of Texas at Austin
ABSTRACT
A relief route is a segment of a highway that moves traffic around the central business district of a city. Planners perceive it as a means of enhancing mobility and often associate regional economic progress with construction of bypasses. Though a bypass often means safer, quieter, less-congested downtowns, the communities receiving a bypass generally worry about potential negative impacts to the local economy. Hence, to make well-informed decisions on constructing relief routes, impact studies are needed. This paper examines the economic impacts of highway relief routes on small- and medium-size communities in Texas. Per capita sales in four different industry sectors were chosen as the indicators of impact.
The models developed suggest that the bypassed cities suffered a loss in per capita sales in all four industrial sectors considered. The magnitude of the traffic volume diverted appeared to be the greatest determinant of the impact. The overall impacts of the bypass were the most negative for gasoline service stations and the least for service industries. The impacts were less negative for cities that had high per capita traffic volumes. In addition, city demographics, regional trends, and proximity to a large city were estimated to have important impacts on the local economy. The industrial sectors considered for analysis represent only a portion of the total economy of the city. Therefore, negative impacts to these sectors do not necessarily mean that the economy as a whole suffers.
INTRODUCTION
Highway relief routes, also known as bypasses, move traffic around central business districts of cities. Relief-route users may experience travel time and cost savings, as well as increased safety. For some, rerouting through traffic is perceived as an advantage as it makes the downtown quieter, safer, and a more pleasant place for shopping (Otto and Anderson 1995). These routes may also affect the local economy negatively in terms of employment and income and sales volume. Thus, the overall impacts of a relief route on the city it bypasses are not obvious and cannot be easily generalized.
Planners view relief routes from a wider regional and state level and see them as one way to enhance intercity travel. On the other side, communities receiving bypasses may be concerned about potential negative economic impacts. These concerns can be critical for small- and medium-size cities that greatly depend on highway traffic. The challenge is to improve statewide mobility without hampering the local economies. Identification and quantification of economic impacts can inform decisionmaking regarding the construction of relief routes.
LITERATURE REVIEW
Considerable research exists on the impacts of bypasses. Some of the earliest studies date back to the 1950s. These and later studies examined the impacts on sales, employment levels, income, land use, land values, and other economic indicators. A wide array of methodologies has been employed, from the simplest forms involving before-and-after studies to more complex, indepth case studies and econometric modeling. Data at different levels of aggregation have also been used. A critique of some of the latest efforts to understand the economic impacts of highway bypasses is presented here.
A Wisconsin study by Yeh et al. (1998) used case study and survey and control-area methodology involving a nonpaired comparison of bypasses and control cities. An analysis of sales and employment data, travel surveys, and focus group interviews indicated little adverse impacts on the overall economy and little retail flight. The communities perceived their bypasses to be generally beneficial. This study shows that most of the medium and large cities bypassed were “natural destinations” and growth was one of the reasons these bypasses were needed. These may be cities where urban planners knew the impacts would not be too negative and so requested a bypass; hence, the sample may be biased. Since all communities that get bypassed will not fall into this category, results of this study cannot be generalized.
A survey and control-area method was adopted to study impacts in Iowa and Minnesota (Otto and Anderson 1995). The impact of the bypass, determined by a “pull factor” (defined as the ratio of the per capita sales in the bypassed community to that in the control group), indicated there was no significant difference in total sales reported by bypassed and control communities. Some redistributional effects were observed when the sales were broken down into components. This analysis, however, did not compare the sales levels before the bypass opened. It is possible that the bypassed cities had higher sales before the bypass, when compared with the control cities. A survey of the local business community helped identify perceptions, and it found that a majority of the respondents favored the bypass. However, results from such opinion-based data could be subjective and biased.
A classic example of a recent application of the before-and-after method is a study undertaken in Yass, Australia (Parolin and Garner 1996). Businesses were surveyed the year before the bypass opened. When surveyed a year later, 43.8% of the retail businesses reported a decrease in gross annual sales, 14% of jobs were lost (mostly in casual and part-time employment), and traffic surveys showed a 50% decrease in highway-generated trade. While these consequences appear significant, the study could not completely isolate the impact of the bypass on the local economy; there were other factors (like the withdrawal of construction workers and the opening of a service station near the bypass) that could not be entirely quantified but clearly affected the results.
Research undertaken for the Kansas Department of Transportation (Burress 1996) resulted in the development of a family of regression equations to explain a multitude of variables, such as city and county sales and employment levels. The effort also resulted in the calibration of a gravity model to obtain estimates of through and local traffic. The impact of the relief route was predominantly captured with indicator (dummy) variables, but several other variables, unrelated to relief routes (e.g., city demographics and regional trends in the industrial sector) were not controlled. The results indicated there were short-term negative impacts on some traffic-related businesses, but all such businesses did not suffer and effects were transitory. The study discovered that the impact of background effects—like the recession of 1990–91—were more significant than the bypass-related effects. Unfortunately, however, most of the models developed in this study had few explanatory variables.
A study of the economic impacts of highway bypasses on small Texas communities was done at the University of Texas at Austin (Anderson et al. 1992). Several methods, including projected development, multiple regression analysis, and cluster analysis, were used. The study concluded that highway bypasses might reduce business in small cities in rural settings. The models indicated a 15% drop in gas station sales and a 10% to 15% drop in sales at eating and drinking establishments. The study used pooled cross-sectional and time-series data; however, it did not use sophisticated methodologies like random-effects models that could extract more meaningful information from the panel data.
Work by Buffington and Burke (1991) used regression analysis on a panel dataset to examine the impacts of bypasses, loops, and radials on employment and wages. The impacts of bypass investments on manufacturing employment at the city level were positive. At the county level, the impacts were positive for both employment and wages. However, all the cities included in this study had some form of highway improvement (relief route, radial, or loop). There were no control cities in the dataset. This could lead to biased results, because all cities receiving highway improvements could have certain characteristics that are different from those cities not receiving such improvements. It is also possible that the general economy was improving, so that net effects appeared positive when in fact they were not as positive as they would have been without the bypass.
In 1996, the National Cooperative Highway Research Program consolidated the state of knowledge in the area of relief-route impacts (NCHRP 1996). Based on a literature review and responses to survey questionnaires sent to state departments of transportation, no conclusive evidence was found of a loss of sales, even in vulnerable locations, due to bypassing alone. This leaves open the possibility that relief routes can mean a loss in certain sales conditions.
The ability of multivariate regression analysis to isolate the marginal influence of a relief route from other factors that can possibly impact local economies makes it appealing for the current work. Studies that employed other methods failed to satisfactorily isolate the bypass impacts and those reviewed here that used regression analysis exhibit some deficiencies (e.g., excluding possibly relevant explanatory variables and sampling bias in the data used).
The work presented here takes a rigorous statistical and methodological approach to model the effects of bypasses on local economies. The impacts on four different industry types are examined using a panel dataset. Further, the impacts are modeled jointly, an approach that has not been adopted before. This research effort focuses on small- and medium-size cities in Texas. The results are intended to assist planners and engineers by providing reliable information on likely economic impacts in communities for which relief routes have been proposed.
While the strength of this study lies in the use of a sophisticated econometric methodology to model the impacts, its limitation lies in the fact that the impacts are measured primarily in terms of changes in the per capita sales in four different industrial sectors. Since the cities modeled here are of small and medium size, non-availability of data limited the extension of the methodology to model other sectors. For the same reasons, other factors characterizing the local economy, like income and employment levels, could not be modeled.
The paper is organized as follows. Data details are described, followed by a description of the modeling methodology. Analytical results are then presented and followed by a summary of conclusions and identification of areas of improvement to the current work.
DATA
We first created a list of cities in Texas with populations between 2,500 and 50,000, and then
traffic maps were reviewed to classify the cities into those that are bypassed and those that are
not. We further classified the bypassed cities based on the nature of their bypass(es), and only
cities with a single bypass were considered for the study. This is the simplest form of bypassing,
where the relief route splits from the old route at one side of the city and rejoins the same
route on the other side. This exercise resulted in the identification of 23 bypassed
cities1
for analysis; 19 other, nonbypassed cities were chosen as “control” cities. For each of the 42 cities, 9 years of data (in years falling between 1954 to 1992) were collected. The sample, therefore, has a total of 378 data points.
We collected sales data for four industrial sectors from the U.S. Economic Census. These include total retail sales (Standard Industrial Classification (SIC) major groups 52–59), sales in gasoline service stations (SIC 554), sales at eating and drinking places (SIC 58), and service receipts (SIC major groups 70 through 89). Gasoline service stations and eating and drinking establishments are subcategories within the retail trade category. The data years are approximately five years apart. Both city- and state-level data were collected. All sales dollars were then adjusted for inflation and converted to current year 2000 dollars using the Consumer Price Index (University of Michigan 2000).
We obtained data on city demographics (e.g., overall population, unemployment, and elderly population), median household income, and average household size from the U.S. Census of Population. The data were used to derive an estimate of income per capita, as the ratio of median household income to the average household size, and incomes were converted to 2000 dollars. Census of Population data covered 1950, 1960, 1970, 1980, and 1990. These data were then linearly interpolated for the required data years.
The proximity of a city in this study to a large city was seen as an influential factor. A large city is defined here as the central city of a metropolitan statistical area (MSA) in 1990. The nearest large city was identified for each city sample, and distances were obtained from the Texas Mileage Guide (Texas Comptroller of Public Accounts 1999). The populations of these large cities were obtained from the U.S Census of Population and linearly interpolated for the required data years.
Using district traffic maps from the Texas Department of Transportation, we were able to infer the year when traffic first appeared on a city’s relief route. These maps were used to determine the opening year of every relief route, and thus the number of years since opening for each data year. The traffic maps also provided data on the average annual daily traffic (AADT) at different count locations along the highways. Counts along the bypass were averaged to get an estimate of the traffic volume on the bypass. Counts on all state, U.S., and Interstate highways that pass through the city were averaged to get an estimate of the total traffic volume approaching the city.
Distances along the old and the new routes were obtained from county maps. The distances were measured from the point where the relief route branches off the old route to the point where it rejoins the old route. The county maps also provided information on the presence of frontage roads along the relief route.
ANALYSIS
Variable Specification
Per capita sales in four different industrial sectors were identified as indicators of the local economy. The industrial sectors are total retail (establishments that primarily sell merchandise for personal or household consumption), gasoline service stations (establishments that primarily sell gasoline and automotive lubricants), eating and drinking places (establishments that primarily sell prepared food and beverages), and service industries (establishments that provide a wide variety of services—e.g., lodging, repairs, health, amusement, legal, and technical—to individuals, businesses, government establishments, and other organizations).
We identified several variables to explain the four types of sales investigated. The impact of city demographics on a local economy is captured by introducing the fraction of population that is elderly (ELDERLY), the fraction of labor force that is unemployed (UNEMP RATE), and per capita income (INCOME PERCAP) as explanatory variables. Per capita income is expected to have a positive impact on per capita sales, while the unemployment rate is expected to have a negative impact. Elderly people may be more likely to shop locally, as opposed to driving out in search of more variety. Hence, an a priori expectation for this explanatory variable may be for a positive effect.
It also is hypothesized that the sales levels of small and medium cities are significantly influenced by the proximity of a large city, and the closer and more populated the large city is, the greater its influence. Thus, the ratio of the population of the nearest large city to its distance from the community under study is introduced as an explanatory variable (LARGECITY POP/DIST). More traffic moving through the city indicates a larger market for local goods and services. Since the models are developed at per capita level, the traffic volume approaching the city was normalized by the population of the city and this (TOT TRAFFIC PERCAP) was introduced as another explanatory variable.
Bypassed cities are identified by introducing an indicator variable (RELIEF ROUTE) that takes a value of one once the relief route is opened to traffic. The impact of a bypass depends on how much traffic and how far away traffic is diverted from a city’s downtown. An estimate of the magnitude of the traffic diverted from the old route to the bypass is obtained as the ratio of traffic volume on the bypass to the total traffic volume approaching the city (TRAFFIC SPLIT). The greater the diversion, the greater the adverse impact on local sales is expected to be. The length of the bypass and the old route could be used as proxies for how far the diverted traffic is moved away from the old route. Variables, DISTOLD and DIST RATIO were introduced to capture this effect. The farther the diversion, the greater the expected negative impact.
The impacts of a relief route can be expected to change with time. The impacts may cease or there might be lagged effects on the community. The coefficient on the NUM YEARS variable captures this effect. A NUM YEARS SQ variable is also introduced to capture possible nonlinear time trends. The signs on these variables can be either positive or negative.
The per capita sales at the state level (STATE SALES PERCAP) for the specific industrial sector also is introduced as an explanatory variable to capture and control for more global trends in industry sales over time. The data year (YEAR) is used to capture other time-related trends.
The 1982 economic censuses provided sales data only for establishments with payrolls. Data for all other economic census years were available for all establishments. To characterize this data issue, an indicator variable (YEAR 1982) was introduced for observations in 1982 in all but the retail sales model (this problem was not observed for retail industry data). Sample means of the variables described are presented in
table 1.
Model Specification
This section describes the econometric model structure and estimation method. A regression model developed on panel data from N cross-sections and T time periods can be represented as follows:
Yit = α + X1,itβ1 + X2,tβ2 + uit, i = 1 to N, t = 1 to T (1)
where
Yit is the dependent variable,
X1,it are variables that vary over both cross section and time,
X2,t are variables that are time specific (the X2,t are cross-section invariant),
α, β1, and β2 are the model parameters to be estimated.
The error terms uit can be broken down into unobservable
cross-section-specific (i.e., city-specific) effects μi and
a remaining term νit. This is the conventional “one-way error components model” (see Baltagi 1995). Alternate model formulations arise depending on the assumptions made regarding the cross-sectional error term. One such formulation is the fixed-effect model, where the cross-sectional error term is estimated as a single constant for each city. Another is the random-effects model, where the cross-sectional error term is assumed to be randomly distributed with a variance
of .
The random-effects formulation has several statistical and practical advantages over the fixed-effects formulation (Maddala 1987) and hence is more suitable for the current work. This is the adopted error structure.
The specification just described models each industrial sector independently. In reality, there could be several unobserved characteristics of the cities that are impacting all modeled sectors of the economy. Therefore, the error terms can be correlated across equations. Estimation of the four equations separately ignores this correlation; hence, the resulting parameter estimates would not be as efficient as they could be (i.e., the standard errors of the unbiased parameters would not be minimized). This facet can be addressed by estimating the four regression equations as a set of “seemingly unrelated regression” (SUR) equations (Baltagi 1995).
In the case of SUR equations, we consider a set of M equations:

where
Yj is the dependent variable,
Zj is the set of explanatory variables,
δj is the vector of parameters to be estimated for equation j (of the M equations estimated jointly).
The error terms uj can again be broken down into unobservable
cross-section-specific (i.e., city-specific) effects μj and a
remaining term νj. The error structure, therefore takes the form

where
IN is an identity matrix of size N,
1T is a T*1 matrix of ones.
Since the equations are permitted to be correlated in their error terms, the cross-sectional
error terms are distributed with a mean of zero and a variance-covariance
matrix, ,
and the remainder error terms are distributed with a mean of zero and variance-covariance
matrix,
(INT is an identity matrix of size NT). In essence, the one-way random-effects model structure is extended to incorporate correlations across equations. The variance-covariance matrix for the set of equations takes the following form (see Baltagi 1995, p. 104):

where
JT is a T*T matrix of ones.
Defining transformation matrices P and Q as

the covariance-matrix can be rewritten as

where
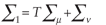
The set of regression equations can be estimated using feasible generalized least squares (FGLS) methods (Baltagi 1995). This requires an estimate of the covariance matrix. A methodology to estimate the variance components from the ordinary least squares (OLS) residuals was developed by Avery (Baltagi 1995) and can be summarized as the following:
![the summation from 1 to hat equals [uppercase u hat superscript {uppercase t} times uppercase p times uppercase u hat] divided by [uppercase n]; the summation from lowercase v to hat equals [uppercase u hat superscript {uppercase t} times uppercase q times uppercase u hat] divided by [uppercase n times (uppercase t minus 1)]](https://webarchive.library.unt.edu/eot2008/20090514093657im_/http://www.bts.gov/publications/journal_of_transportation_and_statistics/volume_05_number_01/images/equ_04_07.gif)
where
is an NT*M matrix of disturbances,
are the OLS residuals for the M equations.
An alternate way of estimating the model is by using maximum likelihood estimation (MLE). Asymptotically, MLE methods are more efficient than FGLS methods, but they require strong error-distribution assumptions and thus may render less robust predictors. Furthermore, Avery’s FGLS estimation is as asymptotically efficient as GLS estimation (Prucha 1984).
If correlations across equations do not actually exist, then the independent estimation of equations is efficient. Therefore, it is useful to test the hypothesis
that all the covariances are zero. This can be accomplished by a statistical test (detailed by
Griffiths et al. 1993, p. 570). If the correlation matrix across the set of M equations
is Σ=[σij], the null hypothesis that all σij
are zero (for i ≠ j) can be tested against the alternate hypothesis
that at least one σij is non-zero using the test statistic λ.
![lowercase lambda equals (uppercase n t) times (the summation from lowercase i equals 2 to uppercase m) times (the summation from lowercase j equals 1 to lowercase i minus 1) times lowercase r hat subscript {lowercase i j} superscript {2}, lowercase r hat subscript {lowercase i j} superscript {2} equals [(lowercase sigma hat subscript {lowercase i j} superscript {2})] divided by [(lowercase sigma hat subscript {lowercase i i} times lowercase sigma hat times lowercase j j)], lowercase sigma hat subscript {lowercase i j} equals [(lowercase u subscript {lowercase i} superscript {uppercase t} times lowercase u subscript {lowercase j})] divided by [uppercase n t]](https://webarchive.library.unt.edu/eot2008/20090514093657im_/http://www.bts.gov/publications/journal_of_transportation_and_statistics/volume_05_number_01/images/equ_04_08.gif)
where
uj is the vector of OLS residuals for equation j.
Under the null hypothesis, the test statistic, λ, is chi-square distributed with M(M–1)/2 degrees of freedom. The results of this hypothesis test are described below.
RESULTS
The estimation methods were coded in the matrix programming language GAUSS (Aptech 1995).
Random-effects models were developed independently for per capita sales in each industrial
sector, and SUR models were developed to model the four industrial sectors jointly.
In each case, the initial specification uses all available explanatory variables;
statistically insignificant variables (t statistic < 1.6) were removed in a
stepwise manner to arrive at the final specification. However, since the relief-route
indicator variable is of fundamental interest, it is left in, regardless of its level
of statistical significance.
The statistical test for the presence of correlation in the error terms across equations
was performed, and the test statistic was estimated to be 239.4. This is significantly
greater than the critical chi-square value; hence, the null hypothesis that all error
correlations are zero is strongly rejected. The random-effects model was then extended
to incorporate correlations across equations, and the system was estimated as a set of
SUR equations. The correctness of the one-way error components structure was not tested
statistically in the case of SUR. However, the null hypothesis that the variance of the
city-specific error term is zero was rejected in the case of random-effects models. This
may be expected to hold even for the SUR case. The SUR models are presented in
tables 2 through 5
(table 2,
3,
4,
5). Correlations between the error terms are presented in
table 6.
The city-specific error term accounts for 40% of the total variance in the model for per capita retail sales. This fraction is 25% for the sales model for gasoline service stations, 36% for sales in eating and drinking places, and 27% for sales in service industries. Based on the estimates of the covariance matrix, it can be inferred that models for per capita sales in service industries and eating and drinking places are correlated the most in their unobserved error terms. The models for sales in retail and eating and drinking places are almost equally correlated in their unobserved error. The other correlations are much less.
The models developed indicate that the draw in traffic from the old to the relief route has a significant negative impact on the sales in the different industrial sectors. Characteristics of the relief route (access control, ratio of distance along the old route to the relief route) and time trends (NUM YEARS and NUM YEARS SQ) are not statistically significant in most of the models.The NUM YEARS SQ variable is, however, negative and statistically significant for the service sales model. This suggests that the longer a relief route has been in place, the lower the per capita sales in the service industries. The coefficient on the relief-route indicator variable, which captures effects not picked up by other relief-route variables, was positive and statistically significant in all models except sales in gasoline service stations, where it was statistically insignificant.
Based on the coefficients estimated on the indicator variable and the percentage split in traffic, it can be inferred that the overall impact of the bypass on each of the sectors examined is negative when the traffic split exceeds a critical value. This critical traffic split is 31% for retail sales, 26% for eating and drinking places, and 43% for service industries. The impact on sales in gasoline service stations is negative irrespective of the magnitude of split. In 1992, the average traffic split was 47%.
We also found that per capita traffic levels in the city are major determinants of the per capita sales levels. Many of the city demographic variables were also estimated to be statistically significant. The nearness to a large city seems to have a positive impact on the sales in the different industrial sectors considered, except the gasoline service stations sector. Sales in the different industrial sectors also seem to be positively influenced by regional trends, as indicated by the coefficient on the state-level sales variables.
Thus, the models developed suggest that the marginal impact of the traffic split due to the bypass on the per capita sales in the four industrial sectors examined is negative. The net impact of the relief route, however, depends on the magnitude of all the variables considered in the model. To get a sense of this magnitude and to compare the impacts across the sectors, the estimated percentage difference in the per capita sales in the four industrial sectors before and two years after the opening of the relief route was calculated.

Four hypothetical cases were considered based on per capita traffic levels. The data for 1992 were divided into quartiles based on per capita traffic volumes. The mean per capita traffic volume was determined for each quartile; these were 1.326, 1.959, 3.180, and 5.132 AADT per person. The mean values for 1992 were used for the other explanatory variables. The impact measures derived are plotted as a function of per capita traffic volumes
(figure 1).
The impact measure derived indicates an overall negative impact of the relief route on the per capita sales of the four industrial sectors analyzed. The impacts are most negative for gasoline service stations but negligible for service industries. The graph indicate that the negative impact decreases as the per capita traffic volumes in the city increase. Higher traffic levels can sustain the local economy even if a fraction of traffic is removed from the old route. It should be noted that average values of the explanatory variables were used to compute the measure and hence it represents the impact on an “average” city. For specific cases, the impact could be more or less severe, depending on the characteristics of the city in question.
As discussed earlier in this work, a higher traffic split to the bypass is estimated to have significant negative impacts on a city’s per capita sales, after controlling for demographic and bypass-related variables. Several city and bypass characteristics were believed to influence traffic splits. Thus, an OLS model was run using the percentage split in traffic as the independent variable and the city and bypass characteristics as predictors. The 87 data points used to develop these models are those where a bypass exists in the larger dataset. The model results are presented in
table 7. Results of this model elucidate some
second-order effects of demographic and city variables on the economies of bypassed cities.
The population of the city was estimated to reduce traffic split (to the bypass). Cities that are highly populated carry higher fractions of approaching traffic. Larger cities were less affected in the original models, after controlling for traffic split, and they were also less likely to lose traffic to the bypass. Thus, a city’s size provides a significant buffer, both directly and indirectly. A city’s proximity to a large city, however, increases the traffic split. Nearby, large cities provide an alternative, and often more attractive, destination; so motorists may rather stop there (as opposed to stopping in the bypassed city). Therefore, proximity to a large city offers conflicting effects: it increases sales, after controlling for traffic split, but also increases traffic split (which is estimated to reduce sales).
The longer the city has been bypassed, the greater is the estimated traffic split. However, the positive effect tapers off with time, as indicated by the negative coefficient on the NUM YEARS SQ variable. In addition, the longer the old route, the greater the estimated traffic split. However, perhaps to offset this, the coefficient on the distance ratio variable was estimated to be positive (and very statistically significant). This is not intuitive, since one would expect many motorists to avoid the bypass when it is longer than the old route. However, many bypassed cities have another highway passing through the city, and the bypass’s location may facilitate traffic turning onto this other road (from the bypassed highway). The distance ratio may indicate the presence of such situations, and thereby be associated with a positive effect. Finally, from the model shown in table 7, the presence of frontage roads along the bypass was also estimated to increase the traffic split.
CONCLUSIONS
In this work, models were developed to study the influence of relief routes on several sectors of local economies of cities. Per capita sales in four industrial sectors (retail, gasoline service stations, eating and drinking establishments, and service industries) were considered as primary indicators of impact. Recognizing the panel nature of the data, a one-way random-effects error structure was chosen. The models were estimated as a system of seemingly unrelated regression equations, allowing correlation among unobserved factors impacting sales in the different sectors.
The models developed suggest that of the four sectors examined, the impact of a bypass is most negative on the per capita sales in gasoline service stations. The impact on the per capita sales in the other three sectors studied depended critically on the magnitude of the traffic diverted. When about half the approaching traffic was diverted to the bypass, all three sectors were negatively impacted. So, the better a relief route works from a traffic standpoint, the greater its adverse impact on local per capita sales. Of all the sectors studied, the service industries were minimally impacted by the bypass. As expected, per capita traffic volumes are estimated to strongly influence local sales. As the traffic levels per capita increase, the negative impacts due to the bypass are lessened.
The study also tried to identify the impact of city demographics and relief-route characteristics on the magnitude of traffic split. Larger cities lost less traffic to the bypass. Proximity to a large city increased the split. The magnitude of the split also increased with time after the opening of the bypass.
Though per capita sales were chosen as key indicators, random-effects models were also developed for the number of establishments per thousand population for each of the four industrial sectors studied (see Srinivasan (2000) for a detailed description of the methodology and empirical model results). These models again suggest that increasing traffic diversion to the new route had a negative impact on the number of establishments in all sectors but the gasoline service stations. Gasoline service stations were also negatively impacted, but this depended on the magnitude of the traffic split.
OLS models were also developed independently to study the impacts of the relief route on the population growth rates and the per capita income levels in the city. Once the city was bypassed, the population growth rate dropped 0.036% every year after the bypass opened. The bypass was also estimated to have a negative impact on the per capita income levels in the city (a decrease of approximately $50 every year after the bypass opened).
The sectors studied in this research can be expected to be the most vulnerable to a relief route. Gasoline service stations and eating and drinking places, respectively, account for only 7% and 8% of retail sales, and retail sales represent about 50% of the total sales (defined as the sum of retail, service, and wholesale industries). Sales in service industries constitute about 16% of total sales. Therefore, any negative impacts on these industrial sectors do not necessarily mean a significant negative impact on a bypassed city’s overall economy.
The total traffic volumes approaching cities with relief routes were larger than those for control cities. This was probably an important reason for constructing the relief routes. Reduction in traffic volumes due to the relief route may have made the bypassed cities more like the less trafficked control cities. The average split in traffic to the new routes was about 47% in 1992. If not for the relief route, the entire traffic volume would have been carried by the old route and the congestion levels probably would have been high.
In light of these findings, transportation planners and cities should carefully consider proposals for relief routes, in order to determine if a bypass is in fact desirable and socially beneficial. Certain sectors of the economy, like gasoline service stations and eating and drinking establishments, could be critically impacted depending on the magnitude of traffic diverted. On the other hand, there are several non-economic benefits that can accrue to affected populations (e.g., safety and ease of movement downtown), and these need to be given fair weight when balancing any costs of concern. The models developed in this research provide means for assessing the magnitude of the impacts on certain sectors.
The current study used econometric modeling methods to study the impact of relief routes on the local economies at an aggregate city level. If spatially disaggregate data were available, similar methods could be extended to study the impacts along specific corridors. It would then be possible to examine possible relocation of businesses. A two-way random-effects model also would be a useful methodological extension to the current work, recognizing systematic variation in unobserved time-specific effects.
In this research effort, the impacts were measured primarily by changes in per capita sales in four different industrial sectors. Other impacts like changes in the number of establishments, population growth rates, and income levels were also modeled, but these were studied independently. A rigorous approach would be to develop a modeling framework that recognizes the dynamic interactions among the several economic indicator variables. Case studies and other investigative methods can illuminate issues like changes to quality of life, which are difficult to quantify and model statistically. Studies that employ a judicious mix of methodologies can help illuminate the different benefits and costs of bypasses. Findings from such studies will aid the planning of future bypasses in ways that improve service levels for through traffic while causing minimal distress to communities bypassed.
ACKNOWLEDGMENTS
The authors wish to acknowledge the financial support of the Texas Department of Transportation and the research assistance of Dr. Susan Handy and Scott Kubly.
REFERENCES
Anderson, S.J., R. Harrison, M.A. Euritt, H.S. Mahmassani, M.C. Walton, and R. Helaakoski. 1992. Economic Impacts of Highway Bypasses, Report 1247-3F. Center for Transportation Research, University of Texas, Austin, Texas.
Aptech Systems Inc. 1995. GAUSS 3.2. Maple Valley, WA.
Baltagi, B.H. 1995. Econometric Analysis of Panel Data. New York, NY: John Wiley and Sons.
Buffington, J.L. and D. Burke, Jr. 1991. Employment and Income Impact of Expenditures for Bypass, Loop and Radial Highway Improvement. Transportation Research Record 1305:224–32.
Burress, D. 1996. Impacts of Highway Bypasses on Kansas Towns, prepared for the Kansas Department of Transportation. Policy Research Institute, University of Kansas, Lawrence.
Griffiths, W.E., R.C. Hill, and G.G. Judge. 1993. Learning and Practicing Econometrics. New York, NY: John Wiley and Sons.
Maddala, G.S. 1987. Recent Developments in the Econometrics of Panel Data Analysis. Transportation Research A 21A(4/5):303–26.
National Cooperative Highway Research Program (NCHRP). 1996. Effects of Highway Bypasses on Rural Communities and Small Urban Areas. Research Results Digest 210. Washington, DC: Transportation Research Board.
Otto, D. and C. Anderson. 1995. The Economic Impact of Rural Bypasses: Iowa and Minnesota Case Studies, Final Report. Midwest Transportation Center, Ames, Iowa.
Parolin, B. and B. Garner. 1996. Evaluation of the Economic Impacts of Bypass Roads on Country Towns, R&D Project TEP/93/6. New South Wales Roads and Traffic Authority, New South Wales, New Zealand.
Prucha, I.R. 1984. On the Asymptotic Efficiency of Feasible Aitken Estimators for Seemingly Unrelated Regression Models with Error Components. Econometrica 52 (1):203–8.
Srinivasan, S. 2000. Economic Impacts of Highway Relief Routes on Small- and Medium-Sized Cities: An Econometric Analysis. Masters Thesis, University of Texas, Austin, Texas.
Texas Comptroller of Public Accounts. 1999. Texas Mileage Guide. Available at http://www.window.state.tx.us/comptrol/texastra.html, as of May 2000.
University of Michigan. 2000. University of Michigan Documents Center, CPI Calculator. Available at http://www.lib.umich.edu/libhome/Documents.center/steccpi.html, as of May 2000.
Yeh, D., M. Gannon, and D. Leong. 1998. The Economic Impacts of Highway Bypasses on Communities, Technical Report SPR-0092-45-93. Wisconsin Department of Transportation, Economic Planning and Development, Madison, Wisconsin.
Address for Correspondence and End Notes
Kara Maria Kockelman, Assistant Professor of Civil Engineering, The University of Texas at Austin, 6.9 E. Cockrell Jr. Hall, Austin, TX78712-1076. Email: kkockelm@mail.utexas.edu.
1 These 23 cities were bypassed between 1965 and 1990.
|