2. Clinical Trial Design and Interpretation of Results
Research Team Members
Components of a Clinical Trial
Using Statistics to Interpret Results
Learning Objectives
Define key members of the research team
Review key components of a clinical trial
Describe the purpose of the randomization,
stratification, and blinding in clinical trial protocols
Name common statistical methods used to interpret
clinical trial results
|
Clinical trials follow strict scientific guidelines that dictate how
a study is designed and who participates in it. The reasons for these
guidelines may not be immediately clear to a person urgently seeking
treatment, but they protect people and provide scientifically sound
results that can lead to truly effective therapies and
techniques.
Designing and implementing a clinical trial requires the many
talents of a multidisciplinary research team. Each team may be set up
differently, depending on an institution's policy and resources.
Typical team members and their responsibilities include:
Principal investigator - oversees all aspects of a
clinical trial, specifically, concept development, protocol
writing, protocol submission for institutional review board (IRB)
approval, participant recruitment, informed consent, and data
collection, analysis, interpretation, and presentation.
Research nurse - coordinates the clinical trial and
educates staff, participants, and referring health care providers.
This nurse acts as an information conduit from the clinical
setting to the principal investigator and vice versa, and assists
the principal investigator with toxicity and response monitoring,
quality assurance, audits, and data management and analysis.
Data manager - handles the management of clinical trial
data, including electronic data entry. Collaborates with the
principal investigator and research nurse to identify what
participant data will be tracked. The data manager also provides
data to monitoring agencies and prepares summaries for interim and
final data analysis.
Staff physicians and nurses - administer treatment to
participants as specified in the protocol; assess and record
toxicity, drug tolerance, and adverse events; collaborate with the
principal investigator and research nurse in observing and
reporting clinical trends; and provide clinical management and
participant education.
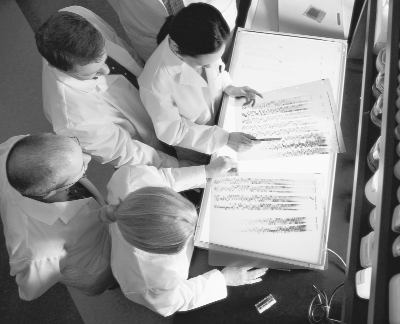
Protocol
Every trial has a written, detailed action plan, called a
protocol. The protocol provides the background, specifies the
objectives, and describes the design and organization of the trial.
Every site participating in the trial uses the same protocol,
ensuring consistency of procedures and enhancing communication. This
uniformity ensures that results from all sites can be combined and
compared.
The clinical trial protocol answers the following questions:
What is the scientific rationale or basis for
conducting the trial?
What are the objectives?
How many participants will be in the trial?
Who is eligible to participate? (This is determined on
the basis of factors such as age and disease status.)
What is the intervention, and what is its duration or
schedule?
What side effects might there be?
What medical tests or followup visits will participants
have? How often?
What information will be gathered about
participants?
What are the endpoints of the trial?
The following FDA-required protocol elements help investigators
answer the questions above and assist participants and health care
professionals in understanding the goals of a clinical trial:
General information
Background information (with relevant references from
the scientific literature)
Trial objectives and purpose
Trial design
Participant selection and withdrawal
Participant treatment
Efficacy assessment
Safety assessment
Statistics
Direct access to source data and documents
Quality control and quality assurance
Ethics
Data handling and record keeping
Financing and insurance
Publication policy
Supplements
Eligibility Criteria
Participant eligibility criteria can range from general (age, sex,
type of cancer) to specific (prior treatment, tumor characteristics,
blood cell counts, organ function). Eligibility criteria may also
vary with trial phase. In phase 1 and 2 trials, the criteria often
focus on making sure that people who might be harmed because of
abnormal organ function or other factors are not put at risk. Phase 2
and 3 trials often add criteria regarding disease type and stage, and
number of prior treatments.
Eligibility criteria might be very detailed if researchers think
that a drug will work best on a specific type of cancer or
population. Trials with narrow eligibility criteria might be
complicated to conduct and might produce less widely applicable
results.
Researchers therefore attempt to include as many types of people
as possible in a clinical trial without making the study population
too diverse to tell whether the treatment might be as effective on a
more narrowly defined population. The more diverse the trial's
population, the more useful the results could be to the general
population, particularly in phase 3 trials. Results of phase 3 trials
should be as generally applicable as possible in order to benefit the
maximum number of people.
The trend today is toward broadening eligibility criteria for
phase 3 clinical trials. Less restrictive criteria may enable more
researchers and people with cancer to participate in these trials.
With more participants, the disadvantages of having a more diverse
population will be outweighed by the results applying more generally
to the population.
Endpoints
An endpoint is a measurable outcome that indicates an
intervention's effectiveness. Endpoints differ depending on the phase
and type of trial. For instance, a treatment trial endpoint could be
tumor response or participant survival. Quality-of-life or supportive
care trial endpoints could include participants' welfare and control
of symptoms.
Examples of endpoints include:
Tumor response rate - the proportion of trial
participants whose tumor was reduced in size by a specific amount,
usually described as a percentage. If 7 of 10 patients responded,
the response rate is 70 percent.
Disease-free survival - the amount of time a participant
survives without cancer occurring or recurring, usually measured
in months.
Overall survival - the amount of time a participant
lives, typically measured from the beginning of the clinical trial
until the time of death.
Tumor response rate is a typical endpoint in a phase 2 treatment
trial. However, even if a treatment reduces the size of a
participant's tumor and lengthens the period of disease-free
survival, it may not lengthen overall survival. In such a case, side
effects and failure to extend overall survival might outweigh the
benefit of longer disease-free survival. Alternatively, the
participant's improved quality of life during the tumor-free interval
might outweigh other factors.
Because tumor response rates are often temporary and may not
translate into long-term survival benefits for the participant,
response rate is a reasonable measure of a treatment's effectiveness
in a phase 2 trial, whereas participant survival and quality of life
are better endpoints in a phase 3 trial.
Randomization
In phase 3 trials (and some phase 2 trials) participants are
assigned to either the investigational or control group by chance,
via a computer program or table of random numbers. This process,
called randomization, gives each person the same chance of being
assigned to either group. Randomization ensures that unknown factors
do not influence the trial results.
|
Randomization is a method used to prevent bias in research. A
computer or a table of random numbers generates treatment
assignments, and participants have an equal chance to be assigned to
one of two or more groups (e.g., the control group or the
investigational group).
|
If physicians or participants themselves chose the group,
assignments might be biased. Physicians, for instance, might
unconsciously assign participants with a more hopeful prognosis to
the experimental group, thus making the new therapy seem more
effective than it really is. Conversely, participants with a less
hopeful prognosis might pick the experimental treatment, leading it
to look less effective than it really is.
Randomization tends to produce comparable groups in terms of
factors affecting prognosis and other participant characteristics. In
this way, randomization guarantees the validity of the conclusion
concerning the effectiveness of the treatment.
Stratification
Stratification is used in randomized trials when factors that can
influence the intervention's success are known. For instance,
participants whose cancer has spread from the original tumor site can
be separated, or stratified, from those whose cancer has not spread.
Assignment of interventions within the two groups is then randomized.
Stratification enables researchers to look at factors in both
groups.
|
Stratification is a process used in randomized trials when
factors that can influence the intervention's success are known.
Assignment of interventions within the two groups is then randomized.
Stratification enables researchers to look in separate subgroups to
see whether differences exist.
|
Blinding
Trials set so that participants do not know which intervention
they are receiving are known as single-blinded trials. Those in which
neither researchers nor participants know who is in the
investigational or control group are called double-blinded trials.
Double-blinded trials ensure that people assessing the outcome will
not be influenced by knowing which intervention a participant is
receiving and also that ancillary followup treatment will be the
same.
Data Collection and Management Tools
Most research teams use standardized and newly created tools to
collect, process, analyze, and audit data. Tools vary in format from
visual analog scales to open-ended questionnaires. Examples of tools
for participants to use to self-report data include diaries,
calendars, logs, and surveys.
The case report form is the basic tool of data abstraction. Many
reports use a Web-based format, others are paper-based. NCI is
constructing an informatics system that will reduce the extensive
paperwork often associated with clinical trials. For example, the
Common Toxicity Criteria (CTC), a Web-based, interactive application,
uses standardized language to identify and grade adverse events in
cancer clinical trials. Forms are also available for rapid reporting
of adverse events, electronically or by telephone, to alert
researchers to potential safety issues. The Adverse Event Expedited
Reporting System (AdEERS) is a Web-based program that enables
researchers using NCI-sponsored investigational agents to expedite
the reporting of serious and/or unexpected adverse events directly to
NCI and FDA.
Researchers use statistical methods to determine whether an effect
observed in a clinical trial is real (statistically significant) or
caused by chance (not statistically significant). Although the
examples included here use terminology and illustrations from
treatment trials, these statistical techniques apply to all types of
clinical trials.
Key Terms
Familiarity with the following terms is useful in understanding
how researchers use statistics to interpret clinical trial
results:
p-values reflect the likelihood that the results
of a clinical trial are because of chance rather than due to a
real difference between the tested treatments. The smaller the
value of p, the greater the likelihood that the results are not
because of chance. A p-value of 0.05 (that is, 1 in 20) or smaller
is widely accepted as an indication that the results are
statistically significant.
Confidence intervals reflect a range of values of the
true value that would be obtained if everyone with a particular
cancer were treated with the treatment under study. The wider the
interval, the more variable the result and the less likely it is
to be close to the true value. Confidence intervals are typically
thought of as the approximate bounds or limits of the true value.
Researchers frequently use either a 95 or a 99 percent confidence
interval.
Sample size is the number of people participating in a
trial.
Statistical power refers to the chance of finding a
statistically significant result when there is one. Ideally,
statistical power should be 0.80 or 0.90 - reflecting an 80 to 90
percent chance of detecting that the true difference in treatment
effectiveness is the smallest size considered medically important
to detect.
Relative risk is the likelihood that cancer will occur
within a specific timeframe in one group versus another.
Statistical Significance
The result of a clinical trial can be statistically significant
(not due to chance) without being clinically significant (medically
important). Suppose, for instance, that a group receiving an
experimental treatment has a 2 percent higher survival rate than the
group receiving the standard treatment. This difference could be
statistically significant, but if participants who survive longer
experience serious side effects, it may not be medically important.
In this case, the side effects might be worth tolerating only if the
experimental treatment group has a 10 percent higher survival rate.
Good trial planning and interpretation take into consideration both
medical importance and statistical significance.
The results of a trial are usually considered statistically
significant when data comparison results in a p-value of 0.05 or
smaller. If the p-value is 0.01 or even 0.001, the results are
considered even more significant because there is less likelihood
that the results are due to chance.
Confidence intervals are often useful data for researchers because
they enable researchers to generalize the results of the trial to the
population.
For example, in a treatment trial with an investigational and a
control group, the mean (average) values of the endpoints (e.g.,
survival for 5 years after treatment) are calculated separately for
each group. Then the standard error - how far the values extend on
either side of the mean - is calculated for each group. The less
overlap between the confidence interval for the standard treatment
group and the experimental treatment group, the more likely the
difference between the groups is statistically significant. Research
reports typically include confidence intervals, for example:
The rate of 5-year survival for group A was 73% (95%
confidence interval, 65.7% to 80.3%). The rate of 5-year survival
for group B was 58% (95% confidence interval, 49.8% to 66.2%). p =
0.004.
In this case, the confidence intervals come close to each other -
65.7 percent and 66.2 percent - but do not overlap. The p-value is
definitely statistically significant.
Confidence intervals can give an indication of whether the results
of small-sized trials that are not statistically significant are
nevertheless medically significant. They can be particularly
important tools when the trial size is limited because the type of
cancer is rare.
Trial Size
The number of participants in a clinical trial greatly influences
its statistical significance. With too few participants, a trial does
not generate enough information to draw a conclusion, and important
results may be missed. On the other hand, by testing more people than
needed to obtain statistically significant results, a trial takes
longer to produce results and may give ineffective or unsafe therapy
to more people than necessary.
When planning a clinical trial, researchers first decide how large
a difference between treatment groups is medically important. Next,
they calculate sample size, or how many people should be enrolled in
the trial. The sample should include enough participants to get a
statistically significant result (a p-value of 0.05 or smaller).
Sample size also influences the statistical power of the research
and is calculated before the trial begins. As sample size increases,
statistical power increases. Ideally, power should be 0.80 or 0.90.
Calculating statistical power helps a researcher decide how many
people to enroll in a trial.
Relative Risk
Relative risk usually describes the risk of getting cancer based
on lifestyle, environmental exposure to cancer-causing agents, or
family history of disease. However, when used in cancer clinical
trial reports, relative risk usually indicates the likelihood that cancer will
occur within a specific timeframe in one group versus another.
Intention to Treat
Phase 3 trials are often analyzed on an intention-to-treat basis -
that is, all participants who were initially admitted into the trial
and randomized are included in the primary analysis.
Intention-to-treat analysis therefore includes people who:
Did not follow instructions
Can no longer be located or contacted
Withdrew from the trial
Did not receive treatment
Including data from the groups above may weaken the results of a
trial, but excluding the data would bias the trial. For instance, if
half of the people in a treatment group withdrew because they thought
the drug they were taking was ineffective and had severe side
effects, and if the other half of the group had a 50 percent response
rate, then excluding the data from the participants who withdrew
makes the drug appear to be 50 percent effective. The actual response
rate is 25 percent. Intention-to-treat analysis typically excludes
participants who were ineligible to be included in the trial but were
randomized.
Refer to the case study for a review and summary of content covered in this workbook.
Back to Top
< Previous Section | Next Section > |