By Patrick Marshall
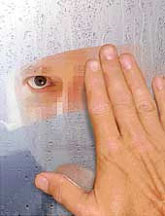 |
Image: Jeff Langkau
If technologies can have bad days, Jan. 28, 2001, was a notably dark one for face recognition technology. While football fans enjoyed the hoopla of that Super Bowl Sunday, face recognition technology received a kick in the groin that still hobbles the industry.
At the game that day in Tampa, Fla., Super Bowl security officials used a face recognition system to scan the faces of the crowd and compare the images to a database of sought-after suspects. The system identified more than a dozen potential matches, all of which turned out to be false.
|
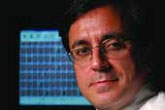 |
“For a time, customers wanted the technology to perform at the levels that you would see in a James Bond film. The technology took a while to get there.” — Joseph Atick, L-I Identity Solutions
Image: Rick Steele
|
The Super Bowl failure was quickly followed by the adoption — and then abandonment — of similar surveillance systems elsewhere in Tampa and in Virginia Beach, Va. And officials at Boston’s Logan International Airport in 2002 declined to implement a face recognition system after tests demonstrated an accuracy rate barely higher than 50 percent.
“The technology was going through growing pains,” said Joseph Atick, executive vice president at L-1 Identity Solutions. “I think there was a setback in the sense that people established an expectation that it wasn’t going to work,” he said of the string of failures in the early 2000s.
But there was an upside. “If you look at the history of any technology, whether it’s the Internet or cell phones, you go through these things where the environment challenges you,” he said. “That sets a program for development. These failures were failures in the sense of an implementation, but they were successes in the sense that they informed the agenda for research for the next five years. And now we’re seeing the results of that focused effort.”
That face recognition technologies have improved significantly in recent years was evident in the results of the most recent Face Recognition Vendor Test sponsored by the National Institute of Standards and Technology. The FRVT acted as a benchmark for the face recognition industry, allowing vendors to come forth and show what they could offer.
The results of the test, released in March, showed improvements in recognition accuracy of an order of magnitude, or 10 times better than in the previous test in 2002.
The FRVT 2006 results showed a false recognition rate (FRR) of only 0.01 while maintaining a false acceptance rate (FAR) of 0.001. That compares to an FRR of 0.2 in 2002.
What does this mean? If the face recognition algorithm of the software was set so that it would not falsely match two images more than once in every 1,000 attempts (that’s what the 0.001 means), the algorithm would fail to match two images of the same person only once in every 100 attempts (the 0.01). In contrast, the 2002 tests mismatched 20 times for every 1,000 attempts. The science of face recognition assumes an inverse relationship between the FRR and the FAR.
Another watershed in the 2006 test was that the accuracy of face recognition software was documented to exceed that of humans. According to the report, “In an experiment comparing human and algorithm performance, the best-performing face recognition algorithms were more accurate than humans.”
So what accounts for the dramatic improvement in face recognition? First, it’s important to understand the basic technologies involved in face recognition. In the initial step, an image must be captured, either by a still camera or a video camera. Next, the image may be “preprocessed” to adjust for lighting, angle or other elements of the recorded image. Finally, an algorithm is applied to extract features — known as landmarks or nodal points — from the image and compare them to data derived from other images.
Typical landmarks are the distance between the eyes, the width of the nose and the length of the jaw line.
A common problem with early face recognition technologies was that changes in lighting conditions and viewing angles could dramatically change the appearance of these features and result in different measurements for the same subject.
The better algorithms made some adjustments for such changes, but with limited data from the captured image, only limited adjustments could be made. Accordingly, face recognition systems could only deliver reasonably reliable results under very controlled conditions, where the viewing angles and lighting are controlled.
Researchers have made some progress in accommodating viewing angles by taking 3-D images — or video — of subjects and then using algorithms to analyze the data. Since subjects are in motion, the algorithm has multiple “reads” of features under changing lighting conditions and angles and can, in principle, make more accurate measurements.
Another promising development has been the introduction of microfeature analysis, which essentially is a detection of patterns in skin texture. This method has only become possible with the introduction of higher-resolution cameras, and it offers an entirely new category of face landmarks.
“In the old days when cameras were 640 x 480 pixels, all you could hope for was to characterize faces based on macro features, such as eyes, noses, mouths,” Atick said. Higher-resolution cameras mean the microstructure of a face — the pattern of pores and the grain of skin — can be recorded and analyzed. This pattern, Atick said, is unique to the point that even identical twins do not share one.
“Now, you’ve established a new secondary signature of the human face,” he said. “When you fuse it with the geometry signature of the human face, you get a significant improvement in performance.”
Atick also attributes the dramatic rise in accuracy of face recognition technologies to the development of better algorithms for compensating for viewing angles.
“In the past, the algorithms were somewhat dumb,” he said. “There has been major improvement in understanding how a two-dimensional image can be projected into three dimensions. It’s called 2-D to 3-D mapping. So we don’t need 3-D cameras. 3-D cameras are a red herring.”
FRVT 2006 was a test of those algorithms. The test was held by NIST’s Face Recognition Grand Challenge, a cross-agency coalition dedicated to improving capabilities of face recognition technology. Participants include the FBI and the Homeland Security Department.
All of the vendor algorithms were tested using the same images, which were of various resolutions and taken under both controlled and uncontrolled lighting conditions. (For more information on FRVT, go to GCN.com, Quickfind 780.) According to Jonathon Phillips, NIST director of the Grand Challenge, the aims of his program have been met, and it’s now up to implementers to carry things forward.
“The Grand Challenge was part of an effort sponsored by numerous government agencies to reduce the error rates by an order of magnitude,” said Phillips. “So we have met the goal here. We have shown that face recognition can meet these goals. Now that the goals have been met, it’s up to these agencies to take the numbers and to decide if it meets their goals or not.”
There is general agreement that the most recent NIST tests will likely result in increased implementation of face recognition technologies in federal agencies. The key question, however, is just what types of tasks face recognition is now up to performing.
Patrick Flynn, professor of computer science and electrical engineering at the University of Notre Dame and one of the FRVT 2006 investigators, noted that FRVT measured the performance of the technology in cooperative, controlled situations but not in uncontrolled conditions with uncooperative subjects.
“Certainly the technology is already useful for many cooperative identification tasks,” Flynn said. “But trying to catch terrorists at the border is a very different thing.”
In fact, the use of face recognition technologies for identification tasks in controlled and cooperative situations at federal agencies has already been growing quickly.
One of the world’s largest face recognition implementations is the US-VISIT program launched in 2004 by the Homeland Security and State departments. When someone applies for a visa, State staff take a photograph of the person, which is then added to the database of images.
More recently, DHS completed a successful pilot program in January 2006 that uses 3-D face recognition to control access to the General Services Administration building.
Although federal agencies and departments have been somewhat slow to implement face recognition technologies, 19 states are already using them for driver’s license applications.
Walter Hamiliton, chairman of the International Biometric Industry Association, said the system in Illinois, for example, snags about five fraudulent applications per business day. The face recognition system does the initial comparison, Hamilton said, then suspect applications are forwarded to a human analyst. “The computer can go quite far, but it can’t make the final adjudication decision.”
Vendors and some analysts are convinced that face recognition technology can play a much bigger security role than just checking visas and driver’s licenses. They argue, in fact, that the technology may already be advanced enough to be useful in many surveillance situations. But the technology is having a hard time living down its reputation from the early years.
“For a time, customers wanted the technology to perform at the levels that you would see in a James Bond film,” Atick said. “The technology took a while to get there.”
Some vendors are, however, still a little skeptical about the federal market. “The bureaucracy within federal agencies is so slow in moving forward,” said Roger Kelesoglu, business development executive at Cognitec Systems. “Now we’re getting a lot of requests and projects that have been on the table for 18 months to two years. They’ve been delayed and delayed and delayed.”
Kelesoglu said he is hopeful that the recent FRVT 2006 report may convince federal agencies that it’s time to move forward. “But I am a businessman,” he added. “The result for me is when I see a purchase order.”
As camera resolutions, image preprocessing and face recognition algorithms improve, the technology becomes capable of performing more sophisticated tasks.
Five years ago, face recognition systems were pretty much limited to matching single still images taken under controlled conditions against a database of images to see if that person was already there.
How good can face recognition technology get? At the very least, Flynn sees an increasing role for face recognition in surveillance of controlled situations, such as checking staff members at the elevator.